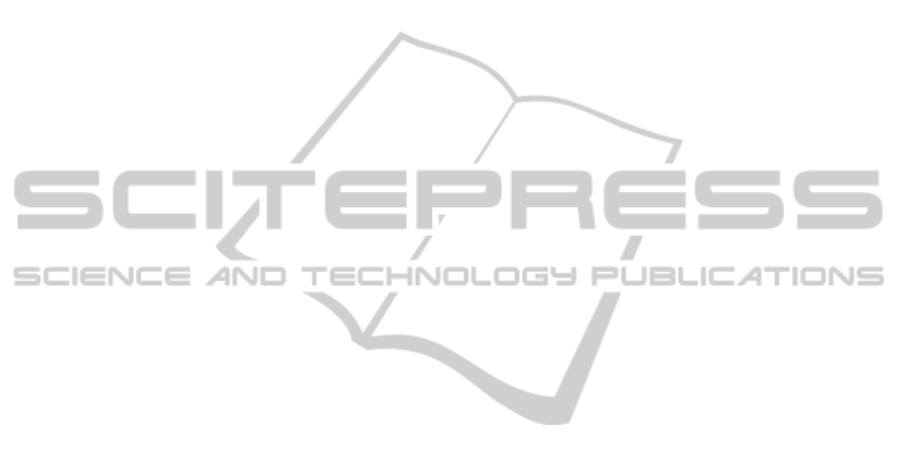
related to the focus of our study. Section 3 describes
the asymmetry between different sellers and
strategies they can adopt against competition. The
stable state of e-marketplace is introduced in section
4, and an analysis of the result is presented in section
5. In section 6 we conduct a simulation to verify the
effectiveness and performance of this model.
Finally, section 7 concludes this paper with a
summary, and explains the potential applications of
this study.
2 LITERATURE
Evolutionary game theory (Maynard Smith, 1982)
applied game theory to evolving populations of life
forms in biology. Despite its original use,
evolutionary game theory has become of increasing
interest to other fields, and many researchers
examined the applications of evolutionary game
theory in economics. An extended analysis of
‘Prisoners’ Dilemma’ by Daniel et al., (2005)
identified four conditions of the game and observed
that each condition has different evolutionary and
informational requirement for cooperation. Witt
(2008) studied the differences between major
approaches in evolutionary economics, and analysed
details of “evolutionary” aspects within the
economy. Hodgson and Huang (2012) inquired both
the differences and similarities between evolutionary
game theory and evolutionary economics, and
proposed potential for mutual emulation in these two
fields. In recent years, the evolutionary game theory
observed significant development, and is now
applied to assist decision-making processes. Altman
et al., (2008), Niyato and Hossain (2009) used
evolutionary game approach in their wireless
network selection study. Barari et al., (2012)
proposed a decision framework that employed
evolutionary game approach in the analysis of green
supply chain contracts. Lee et al., (2010) proposed
an evolutionary game theory based mechanism for
adaptive and stable application in cloud computing.
The classic game models focus on symmetric
competition between players. However, the players
are usually different from each other in real
scenarios, which is the case for our research on e-
marketplaces. The sellers are heterogeneous, varying
in size, location and service level etc. There are less
literature that focus on asymmetric competition but
the following studies provide insight towards our
work. Fishman (2008) extended the analytical
framework of evolutionary game theory to games
that have two distinct types of players, where the
type-specific payoff functions are nonlinear. That is,
asymmetric games where the payoffs for interactions
are influenced by strategies from both types of
players. Liu et al., (2012) proposed a game model
considering the asymmetric interaction and the
selection pressure of resources. Combining
evolutionary game theory with dynamic stability
theory, they concluded that evolutionary results
depend on the asymmetric relation between players,
and on the cost-to-benefit ratio of conflict.
There are also studies on evolutionary game
theory focusing on the economic market. Although
their content does not directly relate to our research,
it still provides context and ground for this study. Ba
et al., (2000) investigated the risk of frauds in e-
marketplace and identified different equilibrium in
the market using an evolutionary game theory
approach. Then the authors explored the best method
to effectuate transactions within the market, and
justified the necessity of trusted third parties for e-
marketplaces. Zheng et al., (2014) provided us
insight into the charging mechanism in e-
marketplaces, as they studied this topic by adopting
the Leontief’s model and drew interesting
conclusions that less sellers generate more profit for
e-marketplace service providers. After studying
previous literature, we base our research on the
hawk-dove game and study the asymmetric between
sellers in e-marketplace. By employing evolutionary
game theory we study the competition between
vendors in the e-marketplace environment and
analyse the optimal profit of the e-marketplace
service providers.
3 SETUP OF ASYMMETRIC
COMPETITION GAME MODEL
IN E-MARKETPLACE (ACGME)
The Hawk-Dove Game is a classic example of
evolutionary game theory applied in animal
behaviour. In this model, we have two animals (not
necessarily birds) that are capable of choosing from
two strategies when in conflict with each other. An
animal can choose the "hawk" strategy and escalate
conflict to a fight or the animal can choose the
"dove" strategy and peacefully back down. Hawk
type animals will always choose to fight, so if two
hawks meet, there will always be a fight. Winners
receive the benefit, while losers be charged with the
cost of the fight. Dove type animals always choose
to flee, and will never be involved in a fight. There
is no cost to be a dove, there is only the possibility
AGame-theorybasedModelforAnalyzingE-marketplaceCompetition
651