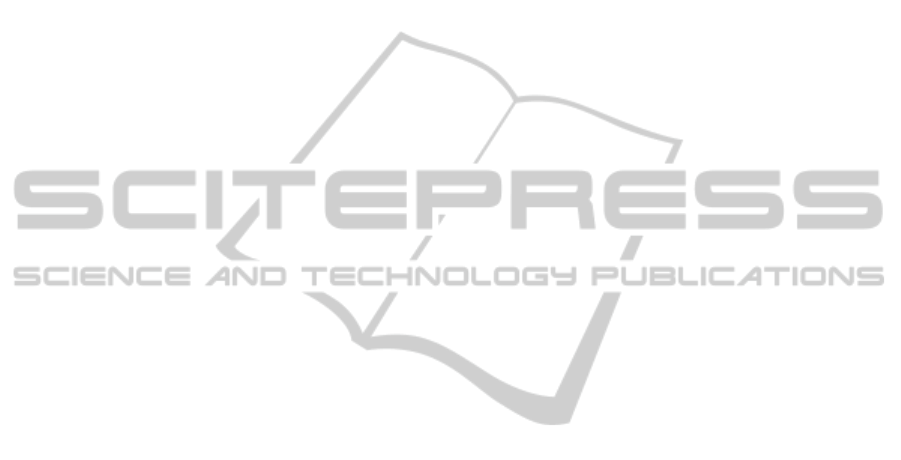
municipality is due to the actions from inhabitants
from other municipalities) and effects at different
scale (as the GWR use the same bandwidth for all
the explanatory variables). It worth noting that some
drivers cannot act with very fuzzy spatial pattern or
no pattern at all (e.g. global economy effect such as
import/export of agriculture goods).
It is likely that the effect of a driver on a given
region is related to the time such driver has been
shaping the landscape and that different drivers have
affect at different temporal and spatial scales, which
makes the interpretation of the results difficult.
Considering the rate of deforestation during different
past periods of time will enable us to analyze the
dynamic of deforestation in its temporal and spatial
dimensions.
5 CONCLUSIONS
Some limitations of this study have been identified
and will be addressed in forthcoming researches.
However, results clearly show the advantages of a
local approach (GWR) over a global one, to assess
different drivers’ effect on LUCC over such a
complex and diverse territory as Mexico.
In future researches, alternative deforestation
rates will be computed, new explanatory variables
such as land tenure will be integrated into the model,
the effect of correlation between explanatory
variables at local scale will be tested and a workshop
will be organized to carry out deep interpretation of
the results.
ACKNOWLEDGEMENTS
This research has been funded by the Consejo
Nacional de Ciencia y Tecnología (CONACyT) and
the Secretaría de Educación Pública (grant
CONACYT-SEP CB-2012-01-178816) and
CONAFOR project: “Construcción de las bases para
la propuesta de un nivel nacional de referencia de las
emisiones forestales y análisis de políticas públicas”.
The authors would like to thank the four reviewers
for their careful review of our manuscript and
providing us with their comments and suggestion to
improve the quality of the manuscript.
REFERENCES
Alix-Garcia, J., de Janvry, A., Sadoulet, E. (2005) 'A Tale
of Two Communities: Explaining Deforestation in
Mexico', World Development, 33(2): pp. 219-235.
Bivand R., Yu D. (2012) Package ‘spgwr’, Geographically
weighted regression. Available at: http://cran.open-
source-solution.org/web/packages/spgwr/spgwr.pdf
(Accessed: 12 April 2013)
Bonilla-Moheno, M., Redo, D. J., Mitchell Aide T., Clark,
M. L., Grau, H. R. (2013) 'Vegetation change and land
tenure in Mexico: A country-wide analysis', Land Use
Policy, 30(1), pp. 355-364.
Bray, D. B., Duran, E., Ramos, V. H., Mas, J. F.,
Velázquez, A., McNab, R. B., Barry, D. and
Radachowsky, J. (2008) 'Tropical deforestation,
community forests, and protected areas in the Maya
Forest', Ecology and Society, 13(2), p. 56.
Bezaury Creel J. E., Torres, J. F., Ochoa-Ochoa, L., Castro
Campos, M., Moreno Díaz, N. G. (2011) Bases de
datos georeferenciadas de áreas naturales protegidas
y otros espacios dedicados y destinados a la
conservación y uso sustentable de la biodiversisad en
México. The Nature Conservancy.
CONAPO (2010) 'Índices de marginación por localidad'.
Available at: http://www.conapo.gob.mx/es/CONA
PO/Indice_de_Marginacion_por_Localidad_2010
(Accessed: 15 April 2013)
ESRI (2011) ArcGIS Desktop: Release 10. Redlands, CA:
Environmental Systems Research Institute.
FAO (2001) 'Global resources assessment', Forestry paper
140.
Figueroa, F., Sánchez-Cordero, V., Meave, J. A., Trejo, I.
(2009) 'Socioeconomic context of land use and land
cover change in Mexican biosphere reserves',
Environmental Conservation, 36 (3), pp. 180–191.
Fotheringham, S. A., Brunsdon, C., Charlton, M. (2002)
Geographically Weighted Regression: the analysis of
spatially varying relationships. John Wiley & Sons.
García-Barrios, L., Galván-Miyoshi, Y. M., Valdivieso
Pérez, I. A., Masera, O. R., Bocco, G., Vandermeer, J.
(2009) 'Neotropical forest conservation, agricultural
intensification and rural outmigration: The Mexican
Experience', BioScience, 59(10), pp. 863-873.
Hansen, M. C., Potapov, P. V., Moore, R., Hancher, M.,
Turubanova, S. A., Tyukavina, A., Thau, D. ,
Stehman, S. V., Goetz, S. J., Loveland, T. R.,
Kommareddy, A., Egorov, A., Chini, L., Justice, C. O.
and Townshend, J. R.G. (2013) 'High-Resolution
Global Maps of 21st-Century Forest Cover Change'
Science 342 (15 November), pp. 850–53. Available at:
http://earthenginepartners.appspot.com/science-2013-
global-forest.
INEGI (2004) 'Carta topográfica escala 1: 250000'. Ed.
INEGI, México.
INEGI (2005) 'Conteo de población y vivienda 2005.
Indicadores del censo de Población y vivienda'. Ed.
INEGI, México.
INEGI (2010) 'Censo de población y vivienda 2010'. Ed.
INEGI, México.
Klooster, D. (2000) 'Beyond Deforestation: The Social
Context of Forest Change in Two Indigenous
Communities in Highland Mexico', Journal of Latin
LocalDeforestationPatternsinMexico-AnApproachusingGeographiccallyWeightedRegression
59