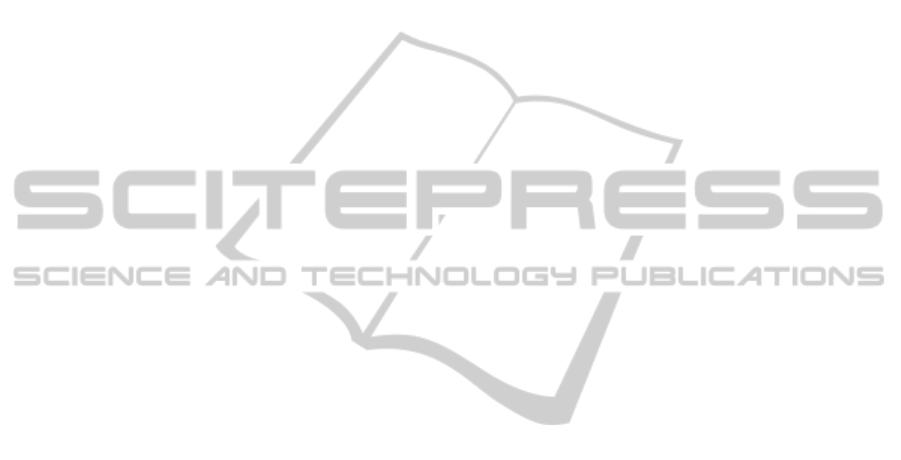
tegrated. Healthcare was seen as the most impor-
tant application area (66.1%), followed by energy
(53.6%), manufacturing and logistics (50.0%) and e-
science (50.0%) with big potential in the short term
future. Other areas were judged less important, such
as tourism (23.2%) and law enforcement (17.9%),
with high uncertainty. We found that our literature-
informed list of challenges were confirmed by our
respondents, however only privacy/security and the
challenge to get qualified personnel was strongly
attributed to the very near future. Algorithm is-
sues, data preservation, evaluation and data owner-
ship were seen as challenges that become more rel-
evant only in the longer run. Research priorities
and funding requests where strongly targeted to pri-
vacy/security (mentioned 22 times), healthcare (men-
tioned 17 times) and semantic issues (mentioned 16
times). This result conforms largely to the findings in
the other parts of the study.
Based on the results of the survey presented in this
paper, along with the outcomes of three workshops
and interviews, a technology roadmap consisting of a
number of objectives was drawn up. The roadmap ac-
tions are described in much greater detail in (Berger
et al., 2014) as part of the complete report that focuses
on all parts of the study. This is outside the scope of
this paper that focuses on the details of the online sur-
vey. In summary, the identified challenges, together
with their careful evaluation, have led to three cate-
gories of actions that are manifested in this roadmap.
First, to meet the challenges of data sharing,
evaluation and data preservation, an objective in the
roadmap is to create a “Data-Services Ecosystem” in
Austria. This is related to an objective to create a le-
gal and regulatory framework that covers issues such
as privacy, security and data ownership, as such a
framework is necessary to have a functioning Ecosys-
tem. In particular, it is suggested to fund a study
project to develop the concept of such an Ecosystem,
launch measures to educate and encourage data own-
ers to make their data and problems available, and
progress to lighthouse projects to implement and re-
fine the Ecosystem and its corresponding infrastruc-
ture. Furthermore, it is recommended to develop a
legal framework and create technological framework
controls to address the pressing challenges of privacy
and security in data analytics.
Second, technical objectives are to overcome chal-
lenges related to data integration and fusion and algo-
rithmic efficiency, as well as to create actionable in-
formation and revolutionise the way that knowledge
work is done. We suggest to fund research that fo-
cuses on future data preservation, to develop fusion
approaches for very large amounts of data, to create
methods that assure anonymity when combining data
from many sources, to enable real time processing,
and to launch algorithmic challenges. A full list of
suggestions are described in more detail in (Berger
et al., 2014).
The third and final objective in the roadmap is to
increase the number of data scientists being trained.
We suggest a comprehensive approach to create these
human resources and competences through educa-
tional measures at all levels: from schools through
universities and universities of applied sciences to
companies. The issue of having more and highly
skilled data scientists soon is an issue that requires
immediate action to secure the future prosperity of the
Austrian (big) data analytics landscape.
ACKNOWLEDGEMENTS
This study was commissioned and funded by the
Austrian Research Promotion Agency (FFG) and the
Austrian Federal Ministry for Transport, Innovation
and Technology (BMVIT) as FFG ICT of the Fu-
ture project number 840200. We thank Andreas
Rauber for his valuable input. Information about the
project and access to all deliverables are provided at
http://www.conqueringdata.com/.
REFERENCES
Agrawal, D., Bernstein, P., Bertino, E., Davidson, S., Dayal,
U., Franklin, M., Gehrke, J., Haas, L., Halevy, A.,
Han, J., Jagadish, H. V., Labrinidis, A., Madden, S.,
Papakonstantinou, Y., Patel, J. M., Ramakrishnan, R.,
Ross, K., Shahabi, C., Suciu, D., Vaithyanathan, S.,
and Widom, J. (2012). Challenges and Opportunities
with Big Data. http://www.cra.org/ccc/files/docs/init/
bigdatawhitepaper.pdf, last visited: August 2013.
Batinic, B. (2003). Internetbasierte Befragungsverfahren.
¨
Osterreichische Zeitschrift f
¨
ur Soziologie, 28(4):6–18.
Berger, H., Dittenbach, M., Haas, M., Bierig, R., Hanbury,
A., Lupu, M., and Piroi, F. (2014). Conquering Data
in Austria. bmvit (Bundesministerium f
¨
ur Verkehr, In-
novation and Technology, Vienna, Austria.
Birnbaum, M. H. (2004). Human research and data col-
lection via the internet. Annual review of Psychology,
55:803–832.
Boyd, D. and Crawford, K. (2012). Critical Questions for
Big Data. Information, Communication & Society,
15(5):662–679.
Dandawate, Y., editor (2013). Big Data: Challenges and
Opportunities, volume 11 of Infosys Labs Briefings.
Infosys Labs. http://www.infosys.com/infosys-
labs/publications/Documents/bigdata-challenges-
opportunities.pdf, last visited: August 2013.
DATA2014-3rdInternationalConferenceonDataManagementTechnologiesandApplications
186