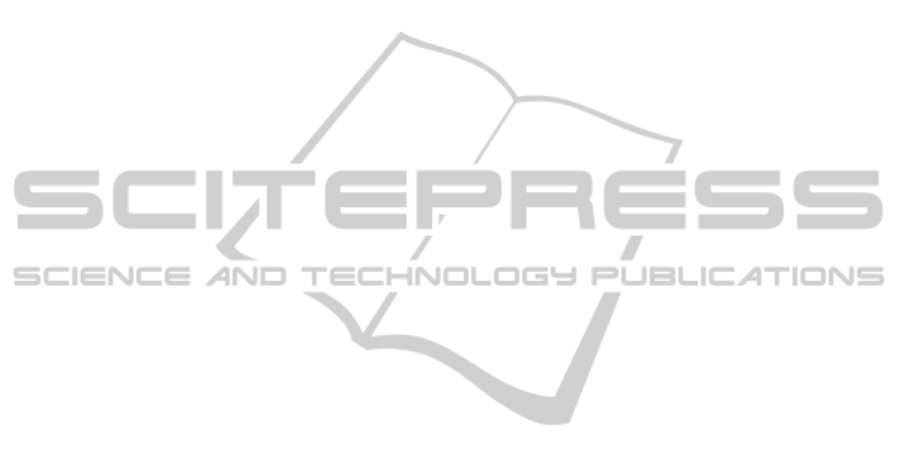
2000). Groups have explicit membership: it is
almost impossible to unknowingly become a
member of a group and it is at least in principle
possible to know the names of all the other group
members. The overhead needed to organize,
schedule and maintain a group is significant. Groups
require commitment and do not scale well in a
learning context to large numbers of people.
2.2 The Network
Over the past few decades there has been an
increasing amount of research into an equally or
more important social form for learning, the
network. Every individual’s networks are different
from every other’s, because networks are constituted
of the people we know. From the weakest ties of
recognition to the strongest friendships, we are
normally members of many overlapping networks,
often without even being particularly aware of it.
Networks are mostly emergent structures based on
the connections we make with others, and their
edges are typically fuzzy and constantly shifting.
Ideas, norms, behaviours and other forms of learning
can and do spread through networks, often with
amazing speed and effect. The Internet has played a
major role in making networks more tangible, most
notably through social networks like Facebook,
LinkedIn and Google+. However, many other
Internet-based systems from emails to instant
messaging to blogs enable the nurturing and growth
of social networks. The network is a fundamental
social form for learning, described by Wenger as a
community of practice (Wenger, 1998), later refined
to the notion of the network of practice (Wenger et
al., 2011) and providing the basis of Siemens’s
Connectivist model (Siemens, 2005). Indeed,
networks play a crucial role in groups, connecting
members within the group as well as sustaining the
exchange of knowledge beyond the group.
2.3 The Set
A third important social form can also be described
that extends beyond groups and networks, and that
has not received anything like as much recognition
in literature on learning: the set. Sets are simply
collections of people with shared attributes who
share the same virtual or physical space. In a
learning context, the most significant shared
attribute tends to be a shared interest in a topic but
others may matter too, such as prior knowledge or
location. In our non-virtual lives we can and do
make use of sets to learn. For example, when we
publish a book or a web page we normally provide
categories (tags) so that people with a particular set
of interests or attributes can find it. We do not know
who they are but, as authors, we are communicating
with and to the set of people who may find it
valuable. Equally, the set can communicate with us:
for instance, the fact that there is a set of people
outside who are carrying umbrellas tells me that I
should probably do the same when I go out. More
deliberate uses of sets are common: shows of hands
in a classroom, divisions of crowds by demographic,
gender, or other lines are a regular feature of our
lives, for better or worse.
Part of the reason for the lack of recognition of
sets for learning till now is due to the fact that, in
most social contexts before the advent of the mass
Internet, sets performed relatively little useful work.
The Internet makes it possible to interact with a vast
number of people with whom we have no shared
social connection at all. Much of the activity that
drives Wikipedia, for instance, is from anonymous
people whose only interaction is in editing one
another’s words. While networks and groups exist
on the Wikipedia site and can play a strong role in
the development of pages, there are at least as many
people contributing to the site who are helping one
another without ever being aware (or caring) who is
helping whom. Likewise, though networks and
groups exist on Q&A sites like Slashdot, Yahoo
Answers or StackExchange, much of the learning
that results from their use emerges from virtually
anonymous interactions between people unknown to
one another and not organized into groups or
networks. Sets are the basis of Google Search,
arguably the most significant learning technology
invented in the last millennium. Sets underpin
crowd-mining technologies such as Amazon’s book
recommendations, Netflix’s movie
recommendations and Pandora’s music
recommendations. Countless specialist sites cater for
particular interests that are, by nature and our
definition, set-oriented. Curation sites like Pinterest
and Learnist are largely set-oriented, focusing on
topics rather than communities. Twitter hashtags are
primarily concerned with sets, not networks or
groups. Usenet newsgroups and email listservs have
long been an important source of knowledge and
dialogue, often among strangers sharing nothing but
an interest in a topic or need for topic-specific
information. Despite the popularity of group-
supporting tools like learning management systems
and network-nurturing tools like Facebook,
Academia.edu and LinkedIn, set-based interactions
are the dominant social form in Internet-based
CSEDU2014-6thInternationalConferenceonComputerSupportedEducation
100