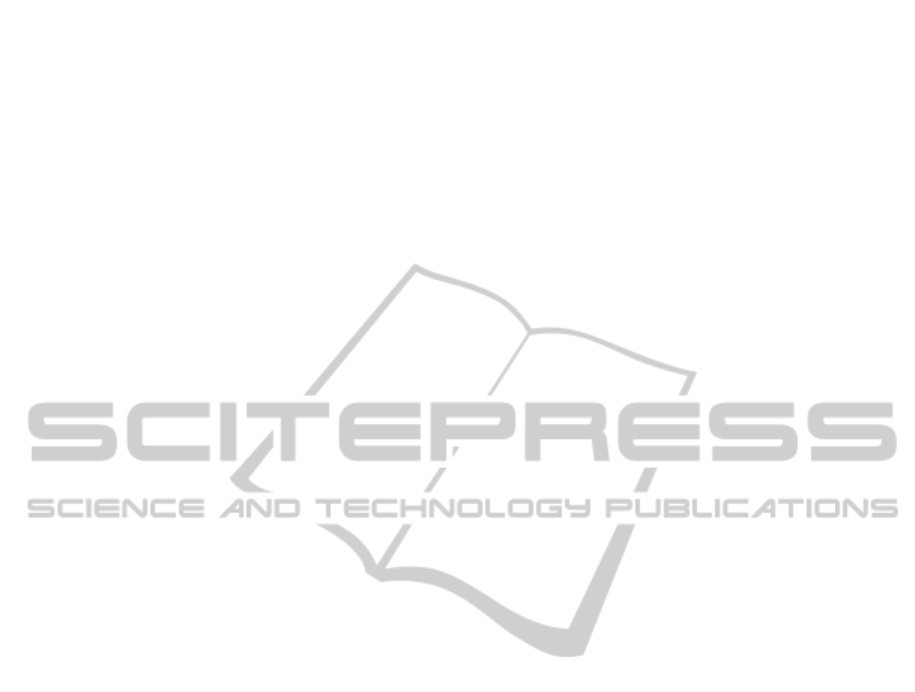
within a short period of time. This model helped to
proactively control data, reactively resolve data
issues, and monitor breaches of data rules and
policies. However, it still needs more
experimentation.
This research has clearly shown evidence of data
errors that are related to the absence of data
governance. Such data errors resulted from the lack
of data privacy and security rules, the lack of data
policies, and the absence of accountability on the
data entered into the Electronic Medical Record
system. The outcomes of this study were presented
to the board of decision-makers in the chosen
healthcare organization. The board decided to
formally start two initiatives: Data Governance
Project and Book of Measures Project, the latter of
which aimed to build an enterprise dictionary of
KPIs, terminologies, and definitions in order to unify
the language used in the hospital.
7 CONCLUSIONS
We believe that applying data governance in
healthcare will provide a solid start for data-driven
projects such as data quality improvement, data
warehousing, healthcare analytics, and business
intelligence. The analytical measures of data alerts,
data quality improvement, policy violation
provenance, rules monitoring, and authority
monitoring will increase the reliability and
transparency of data governance for all users and
regulatory bodies. Studying and comparing the
outcomes of different data governance framework is
an essential piece of future work. This research is a
starting point that directly impacts many interesting
research disciplines pertaining to healthcare data
governance such as business governance, strategic
decisions effectiveness, data error tracking and
assessments of improvements to data quality.
REFERENCES
AHIMA. 2008. Quality Data and Documentation for
EHRs in Physician Practice. Journal of AHIMA, 79(8),
43-48.
Alkouri, A. 2012. DATA OWNERSHIP: WHO OWNS
'MY DATA'?. International Journal of Management
and Information Technology, 2(1). Available from:
<http://www.slideshare.net/alkhouri/data-ownership-
who-owns-my-data>.[10 August 2013].
Batini, C., Mecella, M., & Viscusi, G. 2010. Information
Systems for EGovernment (1sr Edition ed.): Springer.
Bergeron, B., AlDaig, H., Hoque, E., AlBawardi, F. S., &
Alswailem, O. 2007. Developing a Data Warehouse
for the Healthcare Enterprise: Lessons from the
Trenches: HIMSS.
Brown, C. V., & Khatri, V. 2010. Designing Data
Governance. Communications of the ACM, 53(1), 148-
152.
Eppler, M. J. 2006. Managing Information Quality. Berlin,
Heidelberg: Springer
Fisher, T. 2009. The Data Asset: How Smart Companies
Govern Their Data for Business Success (1 ed.):
Wiley; 1 edition (June 22, 2009).
Fu, X., Wojak, A., Neagu, D. Ridley, M., & Travis, K.
2011. Data governance in predictive toxicology: A
review. Journal of Cheminformatics, 3(1). doi:
10.1186/1758-2946-3-24
Househ, M. S., & AlTuwaijri, M. 2011. Early Development
of an Enterprise Health Data Warehouse. In
Borycki,E.,M. et al. (Eds.), International Perspective in
Health Informatics (Vol. 164, pp. 122-126). doi:
10.3233/978-1-60750-709-3-122
Hsu, V. 2009. How Technology Enables Data
Governance. Available from: <http://www.oracle.com/
us/products/applications/master-data-management/
042941.pdf>. [23 August 2013].
Kooper, M. N., Maes, R., & Lindgreen, E. R. 2011. On the
governance of the information: Introducing a new
concept of governance to support the management of
information. International Journal of Information
Management, 31(3), 195-200. doi:10.1016/
j.ijinfomgt.2010.05.009
Lenzerini, M. 2002. Data integration: A theoretical
perspective. Proc. of the 21st ACM SIGMOD-SIGART
Symposium on Principles of Database<p> Systems
(PODS).
Nigel, C. 2012. Data governance. Health Informatics
Society of Australia. 1. Available from:
<http://www.hisa.org.au/?page=thought_lship>.[13
July 2013].
Orr, J. C. 2011. Data Governance for the Executive.
Colorado Springs: Senna Publishing, L.L.C.
Palczewska, A., Fu, X., Trundle, P., Yang, L., Neagu, D.,
Ridley, M., Travis, K. (2013). Towards model
governance in predictive toxicology. International
Journal of Information Management, 33(3),567–582.
Available from: <http://www.inf.brad.ac.uk/~mick/
papers/IJIM1227.pdf>.[1 September 2013].
RapidBI 2007. Business Transformation – a change
strategy. Available from: <http://rapidbi.com/
created/businesstransformation/>.[10 August 2013].
Rishel, W. 2001. HIPAA: An Industry Progress Report.
Available from: <http://www.ehcca.com/presentations
/HIPAA3/202.pdf>. [27 July 2013].
Russom, P. 2008. Data Governance strategies Helping
your Organization Comply, Transform, and Integrate.
TDWI Best Practices Report.
Sarsfield, S. 2009. Data Governance for Imperative.
Cambridge, UK: IT Governance Publishing.
Shay, E. F. (1999). Legal implications of the informatics
revolution. Physician's News Digest. Available from:
HEALTHINF2014-InternationalConferenceonHealthInformatics
186