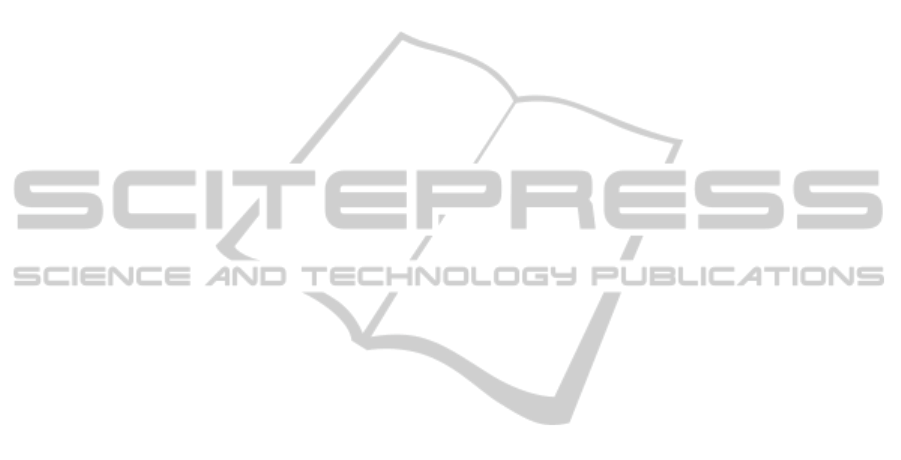
and human energy rates, combined with BSP modal
distribution for any route (as is presented in Figures
2 and 3), this methodology allows estimating the
total energy expenditure (human and electric),
electric autonomy, as well as HR variations,
according to the trip profile. As a result, this
methodology can be applied to evaluate the potential
use of EB in specific situation, namely bike-sharing
routes, providing significant support to bike-sharing
systems design and deployment.
ACKNOWLEDGEMENTS
The authors would like to acknowledge the sponsors
of the research: Prio.e and Eco-critério. Thanks are
also due to Fundação para a Ciência e Tecnologia
for the PhD and Post-Doctoral financial support
(SFRH / BPD / 79684 / 2011, SFRH / BPD / 62985 /
2009).
REFERENCES
Ainslie, P., Reilly, T., Westerterp, K., 2003. Estimating
Human Energy Expenditure, A Review of Techniques
with Particular Reference to Doubly Labelled Water.
Sports Med, 683-698.
Baptista, P., 2013. On-road Monitoring of Electric
Bicycles and its use in Bike-sharing Systems, in:
Silva, C. (Ed.), Grid Electrified Vehicles:
Performance, Design and Environmental Impacts.
Nova Science Publishers, Inc.
Baptista, P., Duarte, G., Mendes, M., Rolim, C., Farias, T.,
2013a. Scenarios for electric bicycle use: from on-road
monitoring to possible impacts of largeintroduction,
NECTAR Conference on Dynamics of Global and
Local Networks, São Miguel Island, Azores (Portugal).
Baptista, P., Pina, A., Duarte, G., Rolim, C., Pereira, G.,
Silva, C., Farias, T., 2013b. On road evaluation of
electric and conventional bicycles for urban mobility.
submitted to Transport Policy.
Barclays Cycle Hire, 2012. Barclays Cycle Hire -
Bikesharing in London.
Buehler, R., Pucher, J., 2012. Walking and Cycling in
Western Europe and the United States - Trends,
Policies, and Lessons. TR News May-June 2012:
Active Transportation: Implementing the Benefits.
Callabike, 2012. Callabike bike sharing system.
Cap'Vélo, 2012. Se déplacer à vélo en Grand Poitiers -
Bikesharing scheme in Saint-Etienne.
Cherry, C., Cervero, R., 2007. Use characteristics and
mode choice behavior of electric bike users in China.
Transport Policy 14, 247-257.
Cherry, C., Weinert, J., Xinmiao, Y., 2009. Comparative
environmental impacts of electric bikes in China.
Transportation Research Part D 14, 281–290.
Dill, J., Rose, G., 2012. E-bikes and Transportation
Policy: Insights from Early Adopters, 91th Annual
Meeting of the Transportation Research Board.
Eco-critério, 2013. http://www.ecocriterio.pt/.
EUROSTAT, 2013. Environment and Energy, EUROPA
Eurostat – Data Navigation Tree,
http://ec.europa.eu/eurostat.
Freemark, Y., 2010. Transit Mode Share Trends Looking
Steady; Rail Appears to Encourage Non-Automobile
Commutes, The Transport Politic.
Galp Energia, 2012. Ciclovias e percursos pedonais.
Gardner, G., 2010. Power to the Pedals. World Watch
Magazine 23.
Gastinger, S., Nicolas, G., Sorel, A., Sefati, H., Prioux, J.,
2012. Energy Expenditure Estimate by Heart-Rate
Monitor and a Portable Electromagnetic-Coil System.
International Journal of Sport Nutrition and Exercise
Metabolism, 117 -130.
Gastinger, S., Sorel, A., Nicolas, G., Gratas-Delamarche,
A., Prioux; J., 2010. A comparison between
ventilation and heart rate as indicator of oxygen uptake
during different intensities of exercise. Journal of
Sports Science and Medicine, 110-118.
Jiménez-Palacios, J., 1999. Understanding and
Quantifying Motor Vehicle Emissions with Vehicle
Specific Power and TILDAS Remote Sensing.
Massachusets Institute of Technology.
Keytel, L., Goedeck, J., Noakes, T., Hiiloskorpi, H.,
Laukkanen, R., Van Der Merwe, L., Lambert, E.,
2005. Prediction of energy expenditure from heart rate
monitoring during submaximal exercise. Journal of
Sports Sciences, 289 – 297.
Lathia, N., Ahmed, S., Capra, L., 2012. Measuring the
impact of opening the London shared bicycle scheme
to casual users. Transportation Research Part C:
Emerging Technologies 22, 88-102.
Lindsay, G., Macmillan, A., Woodward, A., 2011. Moving
urban trips from cars to bicycles: impact on health and
emissions. Australian and New Zealand Journal of
Public Health 35.
Martinez, L., Caetano, L., Eiró, T., Cruz, F., 2012. An
optimisation algorithm to establish the location of
stations of a mixed fleet biking system: an application
to the city of Lisbon, 15th Edition of the Euro Working
Group on Transportation, Paris.
Parkin, J., 2011. The importance of human effort in
planning networks, NECTAR Workshop Abstract.
Prio Energy, 2012. Prio Energy - Electric Mobility.
Pulkkinen, A., Saalasti, S., Rusko, H., 2005. Energy
expenditure can be accurately estimated from HR
without individual laboratory calibration, 52nd Annual
Meeting of the American College of Sports Medicine,
Nashville, Tennessee , USA.
The Bike-sharing Blog, 2011. Japanese Bike-share is
Electric.
Urban Audit, 2012. Urban Audit: Welcome to the Urban
Audit web site.
Vélib, 2012. Vélib - Bikesharing scheme in Paris.
VéliVert, 2012. VéliVert - Bikesharing scheme in Saint-
Etienne.
InfluenceofElectricBicycleUsageonBikerEffort-On-roadMonitoringApplicationinLisbon,Portugal
273