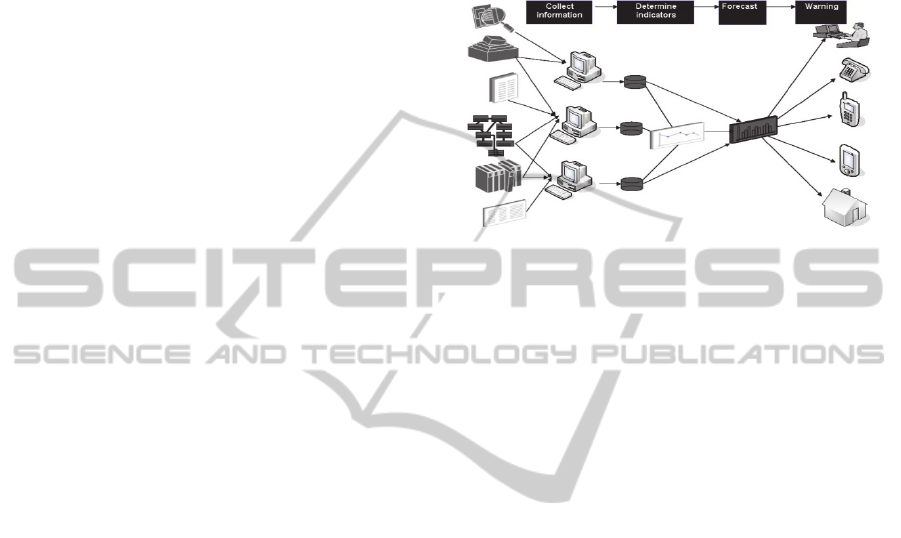
military domains to predict risks and potential
attacks before they occur. Until the early eighties;
the concept of early warning had not evolved
noticeably due to a number of reasons; such as the
difficulty of creating its applications and its high
cost. However, the concept has been rediscovered
again after a series of crises and disasters had taken
place in the world and after witnessing their major
impact on lives and property (Eldin, 2011). The
expression ‘Early Warning’ is used in many fields to
mean the provision of information on an emerging
dangerous circumstance where that information can
enable action in advance to reduce the risks involved
(Basher, 2006). A universally accepted definition of
an EWS does not yet exist and most probably never
will (Sivakuma, 2009). There are many definitions
of an EWS that are used to guide the actions of
individuals, groups, and governments. The formal
UN definition is as follows: “The provision of
timely and effective information, through identifying
institutions, that allows individuals exposed to a
hazard to take action to avoid or reduce their risk
and prepare for effective response” (ISDR, 2003).
An EWS can also be defined as “a social process for
generating maximally accurate information about
possible future harm and for ensuring that this
information reaches the people threatened by this
harm, as well as others disposed to protect them
from the harm” (Glantz, 2004); (Davies and Gurr,
1998). An 'Early Warning Information System
(EWIS)' (see Figure 1.) can be understood as a set of
institutional and technical solutions designed and
implemented in a coherent way to make available, to
a wide range of users and more particularly to
decision makers, information useful to carry out
vulnerability analyses, to evaluate and manage the
risk of a hazard that can become a disaster, and to
manage disasters from prevention to recovery and
rehabilitation (Scott, 2003); (ISCRAM, 2008); (IAD,
2002). The objective of EWIS is to generate accurate
information to empower individuals and
communities threatened by hazards to act in
sufficient time and in an appropriate manner so as to
reduce the possibility of personal injury, loss of life
and damage to property or the environment. We can
use the term EWIS for any information system that
collects, shares, analyzes data, produces future
predictions about potential crises and gives
recommendations or warnings for those involved.
Early Warning Information Systems are still not
widely practiced around the world. .The applications
of EWIS are costly, limited and not widely available
especially in some international organizations. The
best known EWIS is the HEWS- Humanitarian Early
Warning System- used by the Department of
Humanitarian Affairs in the United Nations, and the
GIEWS -The Global Information and Early Warning
System- used by the Food and Agriculture
Organization of the United Nations (Verstegen,
1999).
Figure 1: EWIS architecture.
3 EFFECTIVE EARLY WARNING
INFORMATION SYSTEMS
Many developing countries, in particular the least
developed among them, have limited capacities for
effective early warning systems, and in some cases
they are virtually non-existent (Villagran 2006). The
challenges facing any early warning system are
ineffectiveness and failure to achieve its goals. Any
information system can be called effective if it
supports the organization in reaching its objectives
(Malik, 2001). Effective early warning systems not
only save lives but also help protect livelihoods and
national development gains (United Nations, 2006).
Early warning systems are widely recognized as
worthwhile and necessary investments. However in
many cases, early warning systems do not exist, are
ineffective, or break down at critical points – risking
devastation, death, and destitution (ISDR, 2003).
Two international conferences on early warning, in
1998 and 2003 produced a set of internationally
agreed upon guiding principles for effective early
warning systems. The 1998 Potsdam Conference on
Early Warning Systems and the 2003 Second
International Conference on Early Warning in
Germany addressed technical considerations,
strategic issues and institutional requirements in the
early warning field, Moreover, the conferences made
specific recommendations for strengthening early
warning systems; including increasing the ability of
these systems to be more accurate and flexible
(United Nations, 2006); (EWC, 1998); (EWC-ll,
2003); (EWC-lll, 2006). Furthermore, the core
message of the session “People-Centred Early
AConceptualModelforEffectiveEarlyWarningInformationSystems(EEWIS)
135