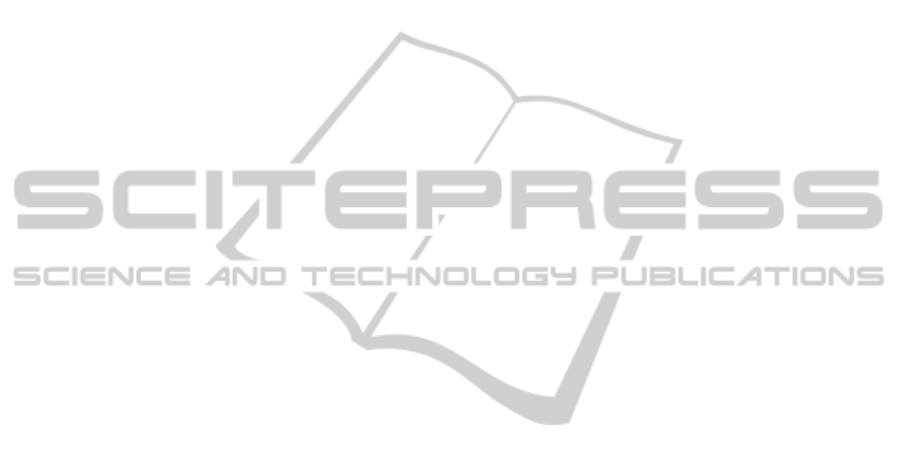
allows concluding that it is impossible to generalise
results in a rigorous manner, as the changes induced
in the load diagrams depend of a large number of
factors that are different from network to network.
The location of the fast charging stations should
be carefully analysed, as they might provoke severe
voltage violations or branches overloading, due to
the large amount of power that they may consume
when in full operation. In fact, the studies performed
have demonstrated that the overload problems
identified in two of the studied networks were likely
provoked by the power consumed in fast charging
stations.
As it happened with the load diagrams, the
simulations performed for the critical mass allow
concluding that it is impossible to generalise results
in a rigorous manner. From the analysis of the
results obtained, it is only possible to conclude that
the critical mass, besides being dependent of the
network considered, increases with the EV
integration level.
In what regards the feasibility of the forecasted
EV integration scenario for 2020 and 2030, it was
possible to conclude that, independently of the
charging strategy adopted, no relevant problems in
the MV networks are expected to occur until 2020.
Conversely, in 2030, several problems are expected
to arise, namely if dumb charging or dual tariff
approaches are adopted. However, as results
presented in section 3.2 show, the forecasted
problems may be entirely solved if the smart
charging is implemented on a large scale.
From the results obtained with the steady-state
analysis performed within the MERGE project, it is
clear that the path to safely integrate large quantities
of EV in distribution networks, without making
large investments in grid reinforcements, is to
implement mechanisms that allow managing the EV
charging not only taking into account their owners’
requests, but also the networks’ technical
restrictions. Nevertheless, it should be remarked that
the adherence to these controlled charging schemes
will ultimately be always a decision of the EV
owners. Thus, it is of utmost importance to timely
define and implement adequate incentives’ policies,
attractive enough to make EV owners willing to
participate in such controlled charging schemes.
ACKNOWLEDGEMENTS
This work was supported in part by Fundação para a
Ciência e Tecnologia under Grants
SFRH/BD/48491/2008 and SFRH/BD/47973/2008
and by the European Union within the framework of
the European Project MERGE – Mobile Energy
Resources in Grids of Electricity, Contract 241399
(7th Framework Programme).
REFERENCES
Bower, E. T., Lopes, J. A. P., Soares, F. J., Rua, D.,
Hatziargyriou, n., Strunz, K. & Ferdowsi, m. 2011.
Initial findings of ‘merge’ (mobile energy resources in
grids of electricity). JSAE EVTeC’11. Japan.
Hasset, B., Bower, E. & Alexander, M. 2011. Evaluation
of the impact that a progressive deployment of EV will
provoke on electricity demand, steady state operation,
market issues, generation schedules and on the volume
of carbon emissions Deliverable D3.2 of the European
Project MERGE.
Lopes, J. A. P., Soares, F. J. & Almeida, P. M. R. 2011.
Integration of Electric Vehicles in the Electric Power
System. Proceedings of the IEEE, 99, 168-183.
Rosa, M., Issicaba, D., Gil, N., Soares, F. J., Almeida, P.
M. R., Moreira, C., Ribeiro, P., Heleno, M., Ferreira,
R., Sumaili, J., Meirinhos, J., Seca, L., Lopes, J. A. P.,
Matos, M., Soultanis, N., Anestis, A., Karfopoulos, E.,
Mu, Y., Wu, J., Ekanayake, J. & Narayana, M. 2011.
Functional Specification for Tools to Assess Steady
State and Dynamic Behaviour Impacts, Impact on
Electricity Markets and Impact of High Penetration of
EV on the Reserve Levels. Deliverable D2.2 of the
European Project MERGE, .
Sánchez, C., Gonzalez, A., Rosa, M., Ferreira, R., Cabral,
P., Batista, F., Issicaba, D., Gil, N., Moreira, C.,
Ribeiro, P., Diaz-Guerra, B., Papadopoulos, P., Grau,
I., Voumvoulakis, E., Zountouridou, E., Karfopoulos,
E., Bourithi, Ferdowsi, M. & Abbasi, E. 2010.
Scenarios for the evolution of generation system and
transmission, distribution grid evolution requirements
for different scenarios of EV penetration in different
countries. Deliverable D3.1 of the European Project
MERGE.
SMARTGREENS2012-1stInternationalConferenceonSmartGridsandGreenITSystems
100