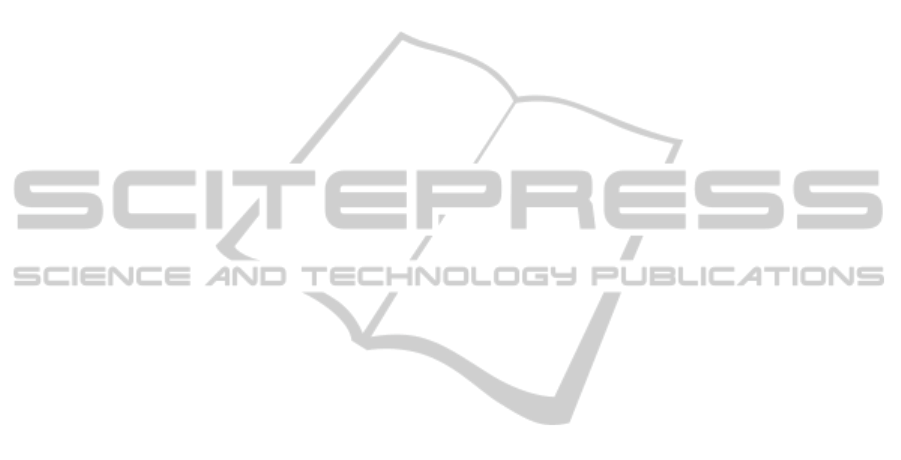
pose to use an EEG test setup analog to the one de-
scribed in (Nonclercq and Mathys, 2010), compris-
ing a generator that produces cerebral-like waves, a
dummy head, an electrode/gel/skin interface model,
electrodes, and leads. Placing this setup in the ref-
erence frame of a subject walking on a treadmill
would produce realistic gait-related motion artifacts
(it would indeed undergo the same shocks as the EEG
electrodes) and should give valuable information to
subsequently reject them.
Finally, if we correctly reject motion artifacts, pro-
vided we know the signals due to descending com-
mands (voluntary rhythmic movements) and those
due to tactile stimulation (tactors, mimicking the sen-
sation of walk), we should be able to disentangle the
contribution of posture and balance control when the
subject is standing and walking.
ACKNOWLEDGEMENTS
This work was funded by the FEDER support (BIO-
FACT). M. Duvinage is a FNRS (Fonds National de
la Recherche Scientifique) Research Fellow. This
paper presents research results of the Belgian Net-
work DYSCO (Dynamical Systems, Control, and Op-
timization), funded by the Interuniversity Attraction
Poles Programme, initiated by the Belgian State, Sci-
ence Policy Office. The scientific responsibility rests
with its author(s).
REFERENCES
Castermans, T., Duvinage, M., and Dutoit, T. (2011). Op-
timizing the performances of a P300-based Brain-
Computer Interface in ambulatory conditions. IEEE
Journal on Emerging and Selected Topics in Circuits
and Systems (JETCAS), Special Issue on ”Brain Ma-
chine Interfaces” (in Press).
Do, A.-H., Wang, P.-T., King, C.-E., Abiri, A., and Nenadic,
Z. (2011). Brain computer interface controlled func-
tional electrical stimulation system for ankle move-
ment. Journal of NeuroEngineering and Rehabilita-
tion, 8(49).
Fitzsimmons, N. A., Lebedev, M. A., Peikon, I. D., and
Nicolelis, M. A. L. (2009). Extracting kinematic pa-
rameters for monkey bipedal walking from cortical
neuronal ensemble activity. Frontiers in integrative
neuroscience, 3(March):19.
Govindan, R., Raethjen, J., Kopper, F., Claussen, J., and
Deuschl, G. (2005). Estimation of time delay by co-
herence analysis. Physica A: Statistical Mechanics
and its Applications, 350(2-4):277 – 295.
Gwin, J. and Ferris, D. (2011). High-density EEG and in-
dependent component analysis mixture models distin-
guish knee contractions from ankle contractions. In
Proc. of the 33rd Annual International Conference of
the IEEE EMBS, Boston, Massachussets, USA, pages
4195 – 4198.
Gwin, J., Gramann, K., Makeig, S., and Ferris, D. (2010).
Removal of movement artifact from high-density EEG
recorded during walking and running. Journal of Neu-
rophysiology, 103(6):3526–3534.
Gwin, J., Gramann, K., Makeig, S., and Ferris, D. (2011).
Electrocortical activity is coupled to gait cycle phase
during treadmill walking. NeuroImage, 54(2):1289 –
1296.
Hanakawa, T. (2006). Neuroimaging of standing and walk-
ing: Special emphasis on parkinsonian gait. Parkin-
sonism and Related Disorders, 12(Supplement 2):S70
– S75. Proceedings of the 1st International Sympo-
sium on Dopaminergic and Nondopaminergic Mecha-
nisms in Parkinson’s Disease (ISDNMPD).
Harada, T., Miyai, I., Suzuki, M., and Kubota, K. (2009).
Gait capacity affects cortical activation patterns re-
lated to speed control in the elderly. Experimental
Brain Research, 193(3):445–454.
Kerick, S. E., Oie, K. S., and McDowell, K. (2009). Assess-
ment of EEG signal quality in motion environments.
Army Research Laboratory Report.
Kiehn, O. (2006). Locomotor circuits in the mammalian
spinal cord. Ann. Rev. of Neuroscience, 29:279 – 306.
Neuper, C. and Pfurtscheller, G. (1996). Post-movement
synchronization of beta rhythms in the EEG over
the cortical foot area in man. Neuroscience Letters,
216(1):17 – 20.
Nonclercq, A. and Mathys, P. (2010). Quantification of the
movement artifact reduction in EEG recording. IEEE
trans. on Biom. Eng., 57(11):2746 – 2752.
Nunez, P., Srinivasan, R., Westdorp, A., Wijesinghe, R.,
Tucker, D., Silberstein, R., and Cadusch, P. (1997).
EEG coherency. Electroencephalography and Clini-
cal Neurophysiology, 103(5):499–515.
Pascual-Marqui, R. (2002). Standardized low-resolution
brain electromagnetic tomography (sloreta): techni-
cal details. Methods Find. Exp. Clin. Pharmacol.,
24(D):5–12.
Presacco, A., Goodman, R., Forrester, L., and Contreras-
Vidal, J. (2011). Neural decoding of treadmill walking
from non-invasive, electroencephalographic (EEG)
signals. Journal of Neurophysiology. in press.
Raethjen, J., Govindan, R., Binder, S., Zeuner, K. E.,
Deuschl, G., and Stolze, H. (2008). Cortical represen-
tation of rhythmic foot movements. Brain Research,
1236:79 – 84.
Solis-Escalante, T., M¨uller-Putz, G., and Pfurtscheller, G.
(2008). Overt foot movement detection in one single
laplacian EEG derivation. Journal of Neuroscience
Methods, 175(1):148 – 153.
Suzuki, M., Miyai, I., Ono, T., and Kubota, K. (2008). Ac-
tivities in the frontal cortex and gait performance are
modulated by preparation. An fNIRS study. Neuroim-
age, 39(2):600 – 607.
Wieser, M., Haefeli, J., B¨utler, L., J¨ancke, L., Riener, R.,
and Koeneke, S. (2010). Temporal and spatial pat-
terns of cortical activation during assisted lower limb
movement. Exp. Brain Res., 203:181–191.
BIOSIGNALS 2012 - International Conference on Bio-inspired Systems and Signal Processing
314