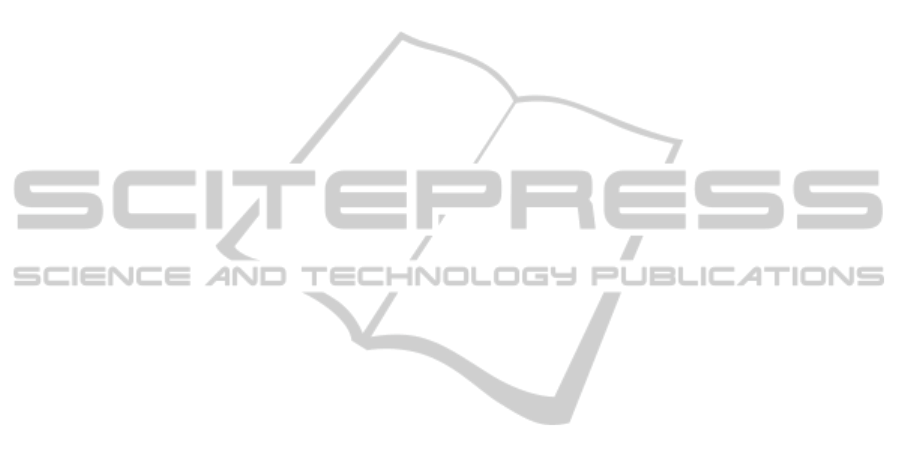
comprehensively described a structured
methodology that focuses on minimizing and
mitigating project specific delay risks and
highlighted that based on utility function, milestones
during project and/or the end of projects or
programme may be categorized in what are called
soft-deadline and hard-deadline (Podean et al, 2010).
In contrast with the soft-end projects, the hard-
end projects posses a decrease of utility function
with a vertical asymptote character around the
deadline for project completion. In extreme
situations, the utility function itself may fall under
zero (projects may generate losses to both
constructor and customer). Existing risk analysis
methodologies observe risks from monetary terms.
The typical risks are correlated with an increase in
final project costs. In order to estimate hard-deadline
milestones and/or end of projects or programme is
critical to employ the time dimension rather than the
typical cost-based risk analysis. Economists
distinguish between cardinal utility and ordinal
utility, the last being a rank-comparison of: options,
contracts, projects, execution quality etc. In risk
assessment activities, customer made already a
decision that company “YZ” is executing the
project. Therefore, the cardinal utility function over
time is more appropriate, while ordinal utility may
captures only ranking and not strength of
preferences (Podean et al, 2010).
2.2 Prospect Theory
The prospect theory was defined by Daniel
Kahneman and Amos Tversky (Kahneman, 1979a),
(Kahneman, 1979b) and is the basic theory in
Reference Class Forecasting.
Reference Class Forecasting for a particular
project requires the following three steps (Flyvbjerg,
2007):
Identification of a relevant reference class of
past, similar projects. The class must be broad
enough to be statistically meaningful, but
narrow enough to be truly comparable with
the specific project;
Establishing a Probability distribution for the
selected reference class. This requires access
to credible, empirical data for a sufficient
number of projects within the reference class
to make statistically meaningful conclusions;
Comparing the specific project with the
reference class distribution, in order to
establish the most likely outcome for the
specific project.
Those theories, that helped Kahneman win the
2002 Nobel Prize in Economics, are based on
some well-observed deviations from rationality,
including the following (Damodaran, 2008),
(Kahneman, 1979a), (Kahneman, 1979b):
Framing: Decisions often seem to be affected by
the way choices are framed, rather than the
choices themselves;
Nonlinear Preferences: If an individual prefers
A to B, B to C, and then C to A, she is violating
one of the key axioms of standard preference
theory (transitivity). In the real world, this type
of behavior is common;
Risk Aversion and Risk Seeking: Individuals
often simultaneously exhibit risk aversion in
some of their actions while seeking out risk in
others;
Source: The mechanism through which
information is delivered may matter, even if the
product or service is identical. For instance,
people will pay more for a good, based on how it
is packaged, than for an identical good, even
though they plan to discard the packaging
instantly after the purchase;
Loss Aversion: Individuals seem to feel more
pain from losses than from equivalent gains.
Individuals will often be more willing to accept a
gamble with uncertainty and an expected loss
than a guaranteed loss of the same amount, in
clear violation of basic risk-aversion tenets.
In his paper, (Flyvbjerg, 2007) agrees that when
contemplating what planners can do to improve
decision making, we need to distinguish between
two fundamentally different situations: (1) planners
and promoters consider it important to get forecasts
of costs, benefits, and risks right, and (2) planners
and promoters do not consider it important to get
forecasts right, because optimistic forecasts are seen
as a necessary means to getting projects started.
Kahneman and Tversky (Kahneman, 1979a),
(Kahneman, 1979b) replaced the utility function,
which defines utility as a function of wealth, with a
value function, with value defined as deviations
from a reference point that allows for different
functions for gains and losses. In keeping with
observed loss aversion, for instance (Damodaran,
2008), the value function for losses was much
steeper (and convex) than the value function for
gains (and concave) as presented in Figure 1.
The implication is that how individuals behave
will depend on how a problem is framed, with the
decision being different if the outcome is framed
relative to a reference point to make it look like a
gain as opposed to a different reference point to
ICE-B 2011 - International Conference on e-Business
44