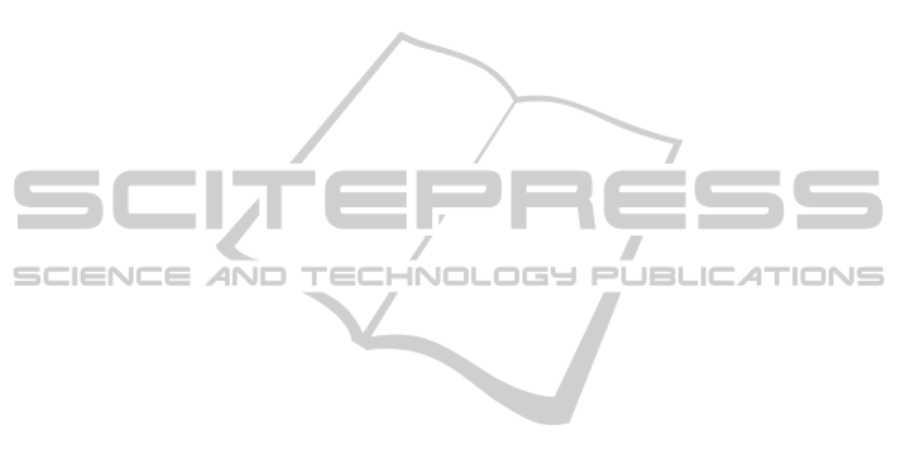
project, Elizabeth Kendall, Scott Baum, Heidi
Muenchberger and Tan Yigitcanlar.
REFERENCES
Bennett, C, Graham, ID, Kristjansson, E, Kearing, S. A.,
Clay, K. F. & O'Connor, A. M. (2010) Validation of a
Preparation for Decision Making Scale. Patient
Education and Counseling 78: 130-133 Elsevier.
Bishop, P. and Davis, G. (2001). Developing consent:
consultation, participation and governance. In Are You
Being Served? Eds. G. Davis and P. Weller. Sydney,
Allen & Unwin: 175–195.
Campbell, S. and Fainstein, S. S. (1996). Introduction: the
structure and debates of planning theory. In Readings
in urban theory, Eds. S. Campbell and S.S. Fainstein.
Oxford, Blackwell Publishing:1-16.
Cromley, E. K. and McLafferty, S. L. (2003). GIS and
public health. Health & Place 9(3): 279.
Dean J. R., J. W. and Sharfman, M. P. (1993). Procedural
rationality in the strategic decision-making process.
Journal of Management Studies 30(4): 587-609.
Duhl, L. J., & Sanchez, A. K. (1999). Healthy cities and
the city planning process. A Background
Documentation On Links Between Health And Urban
Planning, Copenhagen, WHO, Regional Office For
Europe.
Dur, F., Yigitcanlar, T. and Bunker, J. (2009). A decision
support system for sustainable urban development: the
integrated land use and transportation indexing model.
Proceedings of the Postgraduate Infrastructure
Conference. Brisbane, Australia, 26 March.
Queensland University of Technology.
Flood, P., Hannan, E., Smith, K., Turner, T., West, M., &
Dawson, J. (2000). Chief executive leadership style,
consensus decision making, and top management team
effectiveness. European Journal of Work &
Organizational Psychology 9(3), 401-420.
Flynn, B. C. (1996). Healthy cities: toward worldwide
health promotion. Annual Review of Public Health 17:
299-309.
Gudes, O., Kendall, E., Yigitcanlar, T., Pathak, V. and
Baum, S. (2010). Rethinking health planning: a
framework for organising information to underpin
collaborative health planning. Health Information
Management Journal 39 (2): 18-29.
Han J. H., Kendall, E., Sunderland, N., Gudes, O. and
Henniker, G. (2010) Chronic disease, geographic
location and socioeconomic disadvantage as obstacles
to equitable access to e-health. Health Information
Management Journal 39 (2): 30-36.
Healthy Cities Illawarra (2010). Working with the
community for a healthier, safer, greener and more
caring Illawarra. Wollongong: Illawarra city council.
Available at: http://www.healthycitiesill.org.au/
contactus.htm (Accessed 10 October 2010).
Higgs, G. and Gould, M. (2001). Is there a role GIS in the
'new NHS'? Health & Place 7(3): 247-59.
Mooney, G. and Fohtung, N.G. (2008). Issues in the
measurement of social determinants of health. Health
Information Management Journal 37(3): 26-30.
Murray, D. J. (2006). A critical analysis of communicative
planning theory as a theoretical underpinning for
integrated resource and environmental management.
Unpublished PhD thesis, Griffith University,
Queensland.
National Electronic Decision Support Taskforce. (2008).
Electronic Decision Support Systems Report.
Department of Health and Ageing. Canberra:
Australia.
NHHRC (2009). A healthier future for all Australians.
Canberra, ACT, National Health and Hospitals Reform
Commission.
Northridge, M. E., Sclar, E. D. and Biswas, P. (2003).
Sorting out the connections between the built
environment and health: a conceptual framework for
navigating pathways and planning healthy cities.
Journal of Urban Health 80(4): 556-568.
Parnell, J. A. and Bell, E. D. (1994). ‘The propensity for
participative decision making scale: A measure of
managerial propensity for participative decision
making.’ Administration & Society 25(4): 518-533.
Reeves, P. N. and Coile, R. C (1989). Introduction to
health planning. Arlington, VA: Information
Resources Press.
Reinke, W. A. (1972). Health planning: qualitative
aspects and quantitative techniques. Baltimore, MD:
Johns Hopkins University School of Hygiene and
Public Health, Department of Internet Health.
Schulz, A. and Northridge, M.E. (2004). Social
determinants of health: implications for environmental
health promotion. Health Education & Behaviour
31(4): 455-471.
Turban, E. (1993). Decision support and expert systems:
management support systems: Prentice Hall PTR
Upper Saddle River, NJ, USA.
Wave, V. (2009). mHealth for development: the
opportunity of mobile technology for healthcare in the
developing. United Nations Foundation and Vodafone
Foundation.
WHO (1997). Twenty steps for developing a healthy cities
project. WHO Regional Office of Europe.
WHO (1999). Health: creating healthy cities in the 21st
century. In The Earthscan reader in sustainable cities.
Ed. D, Satterthwaite. London, Earthscan: 137–172.
WHO (2010). Urban health Healthy Cities. Available at:
http://www.euro.who.int/en/what-we-do/health-
topics/environmental-health/urban-
health/activities/healthy-cities (Accessed 9 October
2010).
HEALTHINF 2011 - International Conference on Health Informatics
154