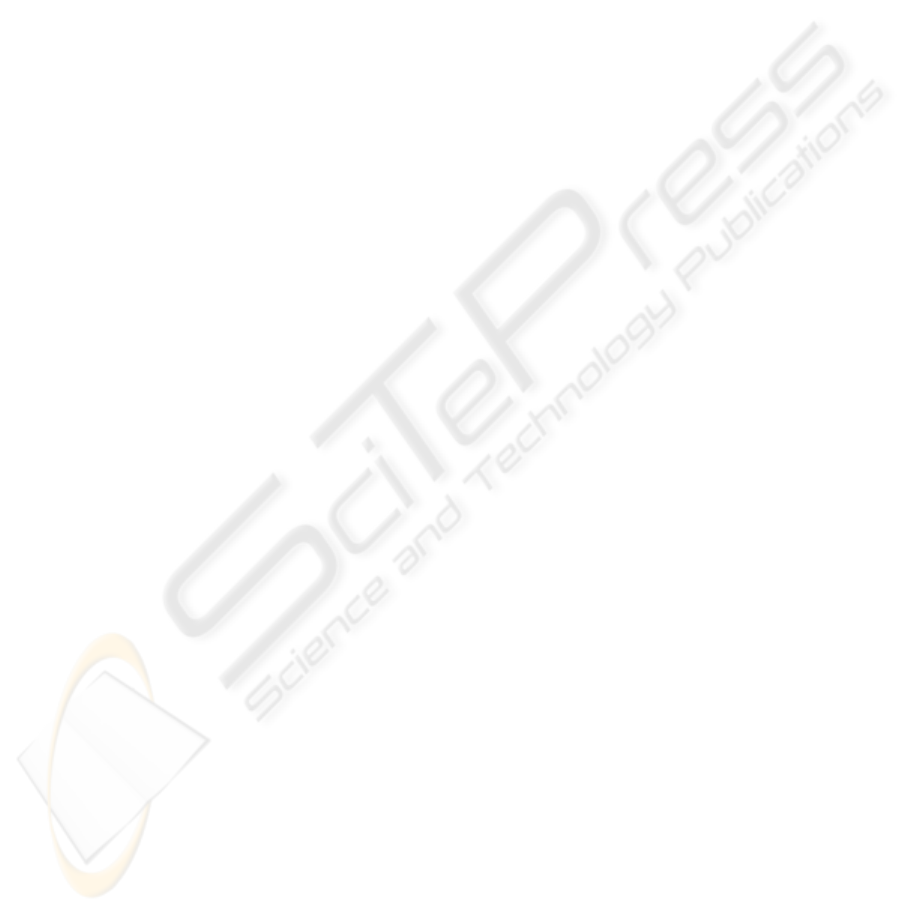
involves only limited expense and effort while
significantly increasing the profitability of the
company under consideration.
There is a large body of research around the
topic of price dispersion in the Internet but only a
limited amount of work has gone into studying what
are the resulting degrees of freedom for companies
and how they should use them. This paper opens
substantial opportunities for future studies on this
topic. Research questions include for example: (i)
How does the demand curve change over time? (ii)
What impact do the brand awareness of a company
and the uniqueness of its products have on the
demand curve? (iii) What kinds of reciprocal
dependencies are there between price components of
an order (Hamilton 2008)? (iv) Can user groups be
identified that demonstrate varying degrees of
willingness to pay? (v) Do we have to take customer
life time value into account when optimizing the
long-term profitability?
This paper is meant as a starting point for
discussion and further research related to optimizing
prices in e-commerce by determining the exact
demand curve for products and services in different
circumstances.
ACKNOWLEDGEMENTS
The comments from the unknown referees are
gratefully acknowledged.
REFERENCES
Baker, W. L., Lin, E., Marn, M. V., Zawada, C. C.,
Getting prices right on the Web, McKinsey
Quarterly, no. 2, p. 54-63, 2001
Baye, M. R., Morgan, J., Scholten, P., Price Dispersion in
the small and in the large: evidence from an internet
price comparison site, Journal of Industrial
Economics, vol. 51, no. 4, p. 463-496, 2004
Böhme, R., Koble, S., Pricing Strategies in Electronic
Marketplaces with Privacy-Enhancing Technologies,
Wirtschaftsinformatik, vol. 49, no. 1, p. 16-25, 2007
Bock, G. W., Lee, S.-Y. T., Li, H. Y., Price Comparison
and Price Dispersion: Products and Retailers at
Different Internet Maturity Stages, International
Journal of Electronic Commerce, vol. 11, no. 4, p.
101, 2007
Brynjolfsson, E., Smith, M. D., Frictionless Commerce? A
Comparison of Internet and Conventional Retailers,
Management Science, vol. 46, no. 4, p. 563-585, 2000
Chernev, A., Reverse Pricing and Online Price Elicitation
Strategies in Consumer Choice, Journal of Consumer
Psychology, vol. 13, no. 1, p. 51-62, 2003
Daripa, A., Kapur, S., Pricing on the Internet, Oxford
Review of Economic Policy, vol. 17, p. 202-216, 2001
Hamilton, R. W., Srivastava, J., When 2 + 2 Is Not the
Same as 1 + 3: Variations in Price Sensitivity Across
Components of Partitioned Prices, Journal of
Marketing Research, vol. 45, no. 4, p. 450-461, 2008
Hann, I.-H., Terwiesch, C., Measuring the Frictional Costs
of Online Transactions: The Case of a Name-Your-
Own-Price Channel, Management Science, vol. 49, no.
11, p. 1563-1579, 2003
Kannan, P.K., Kopalle, P., Dynamic Pricing on the
Internet: Importance and Implications for Consumer
Behavior, International Journal of Electronic
Commerce, vol. 5, no. 3, p. 63-83, 2001
Knoop, M., Effective pricing policies for E-Commerce
Applications – a field study, internal thesis, University
of Applied Science Lüneburg, 2004
Odlyzko, A., Privacy, economics, and price
discrimination on the Internet, in Proceedings of the
5th international conference on Electronic commerce,
ACM, p. 355-366, 2003
Patzer, G. L., Experiment-Research Methodology in
Marketing: Types and Applications. Westport,
Connecticut: Quorum/Greenwood, 1996
Roth, A. E., Ockenfels, A., Last-Minute Bidding and the
Rules for Ending Second-Price Auctions: Evidence
from eBay and Amazon Auctions on the Internet, The
American Economic Review, vol. 92, no. 4, p. 1093-
1103, 2002
Simon, H., Dolan, R. J., Power Pricing: How Managing
Price Transforms the Bottom Line, The Free Press,
New York, 1996
Spann, M., Skiera, B., Schäfers, B., Measuring individual
frictional costs and willingness-to-pay via name-your-
price mechanisms, Journal of Interactive Marketing,
vol. 18, no. 4, 2004
OPTIMIZING PRICE LEVELS IN E-COMMERCE APPLICATIONS - An Empirical Study
43