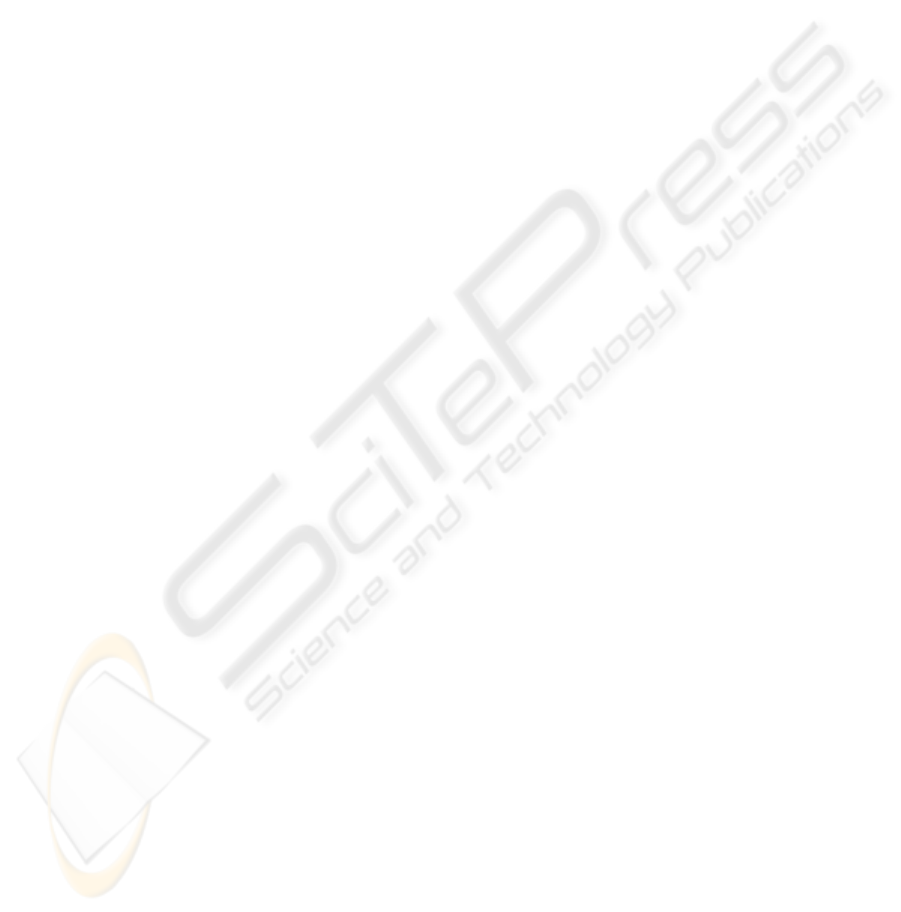
REFERENCES
Schwartz P.J., Malliani A., 1975. Electrical alternation of
T wave: clinical and experimental evidence of its
relationship with the sympathetic nervous system and
with the Long QT Syndrome. Am Heart J,; 89(1): 45-
50.
Zareba W., Moss A.J., Le Cessie S., Hall W.J., 1994. T
wave Alternans in idiopathic Long QT Syndrome. J
Am Coll Cardiol, 23(7): 1541-1546.
Adam D.R., Smith J.M., Akselrod S., Nyberg S., Powell
A.O., Cohen R.J., 1984. Fluctuations in T-wave
morphology and susceptibility to ventricular
fibrillation. J Electrocardiol, 17(3): 209-218.
Smith J.M., Clancy E.A., Valeri C.R., Ruskin J.N., Cohen
R.J., 1988. Electrical alternans and cardiac electrical
instability. Circulation, 77: 110-121.
Rosenbaum D.S., Jackson L.E., Smith J.M., Garan H.,
Ruskin J.N., Cohen R.J., 1994. Electrical alternans and
vulnerability to ventricular arrhythmias. N Engl J Med,
330(4): 235-241.
Kusmirek S.L., Gold M.R., 2007. Dynamic changes of T-
wave alternans: does it predict short-term arrhythmia
vulnerability?. J Cardiovasc Electrophysiol, 18(5):
518-519.
Klingenheben T., Ptaszynski P., 2007. Clinical
significance of microvolt T-wave alternans.
Herzschrittmacherther Elektrophysiol, 18(1):39-44.
Narayan S.M., 2007. T-wave alternans and human
ventricular arrhythmias: what is the link?. J Am Coll
Cardiol, 49(3): 347-349.
Rosenbaum D.S., Albrecht P., Cohen R.J., 1996.
Predicting sudden cardiac death from T wave alternans
from the surface electrocardiogram: promises and
pitfalls. J Cardiovasc Electrophysiol, 7(11): 1095-
1111.
Klingenheben T., Zabel M., D’Agostino R.B., Cohen R.J.,
Hohnloser S.H., 2000. Predictive value of T-wave
alternans for arrhythmic events in patients with
congestive heart failure. Lancet, 356(9230): 651-652.
Ikeda T., Saito H., Tanno K., Shimizu H., Watanabe J.,
Ohnishi Y., Kasamaki Y., Ozawa Y., 2002. T-wave
alternans as predictor for sudden death after
myocardial infarction. Am J Cardiol, 89(1): 79-82.
Tapanainen J.M., Still A.M., Airaksinen K.E., Huikuri
H.V., 2001. Prognostic significance of risk stratifiers
of mortality, including T wave alternans, after acute
myocardial infarction: results of prospective follow-up
study. J Cardiovasc Electrophysiol, 12(6): 645-652.
Bigger J.T., Bloomfield D.M., 2007. Microvolt T-wave
alternans: an effective approach to risk stratification in
ischemic cardiomyopathy?. Nat Clin Pract Cardiovasc
Med, 4(6):300-301.
Ikeda T., Yoshino H., Sugi K., Tanno K., Shimizu H.,
Watanabe J., Kasamaki Y., Yoshida A., Kato T., 2006.
Predictive value of microvolt T-wave alternans for
sudden cardiac death in patients with preserved
cardiac function after acute myocardial infarction:
results of a collaborative cohort study. J Am Coll
Cardiol, 48(11): 2268:2274.
Burattini L., Zareba W., Burattini R., 2006. Automatic
detection of microvolt T-wave alternans in Holter
recordings: Effect of baseline wandering. Biomedical
Signal Processing and Control, 1(2): 162-168.
Burattini L., Zareba W., Burattini R., 2007. Heart-rate
adapting match filter detection of T-wave alternans in
experimental Holter ECG recordings. In: Proceeding
of the Fifth IASTED International Conference on
Biomedical Engineering, Gardner J.W. Ed., ACTA
Press, Zurich: 346-351.
Burattini L., 1998. Electrocardigraphic T-wave alternans
detection and significance. Doctoral thesis. University
of Rochester, Rochester NY.
Burattini L., Zareba W., Moss A.J., 1999. Correlation
method for detection of transient T-wave alternans in
digital ECG recordings. Ann Noninvasive
Electrocardiol, 4(4): 416-424.
Pelicano N., Oliveira M., da Silva N., Anao A., Feliciano
J., Fiarresga A., Alves S., Silva S., Quininha J., 2006.
Assessment of T-wave alternans after acute
myocardial infarction: influence of timing of PTCA on
cardiac electrical stabilization. Rev Port Cardiol,
25(4): 379-387.
Ikeda T., Sakata T., Takami M., Kondo N., Tezuka N.,
Nakae T., Noro M., Enjoji Y., Abe R., Sugi K.,
Yamaguchi T., 2000. Combined assessment of T-wave
alternans and late potentials used to predict arrhythmic
events after myocardial infarction. A prospective
study. J Am Coll Cardiol, 35(3): 722-730.
Puletti M., Curione M., Righetti G., Jacobellis G., 1980.
Alternans of the ST segment and T wave in acute
myocardial infarction. J Electrocardiol, 13(3): 279-
300.
Lilliefors H.W., 1967. On the Kolmogorov-Smirnov test
for normality with men and variance unknown. J Am
Stat Assoc, 62:399-402.
Cox V., Patel M., Kim J., Liu T., Sivaraman G., Narayan
S.M., 2007. Predicting arrhythmia-free survival using
spectral and modified-moving average analyses of T-
wave alternans. Pacin Clin Electrophysiol, 30(3):352-
358.
Richter S., Duray G., Hohnloser S.H., 2005. How to
analyze T-wave alternans. Heart Rhythm, 2(11):1268-
1271.
Nearing B.D., Huang A.H., Verrier R.L., 1991. Dynamic
tracking of cardiac vulnerability by complex
demodulation of the T wave. Science, 252(5004): 437-
440.
BIOSIGNALS 2008 - International Conference on Bio-inspired Systems and Signal Processing
192