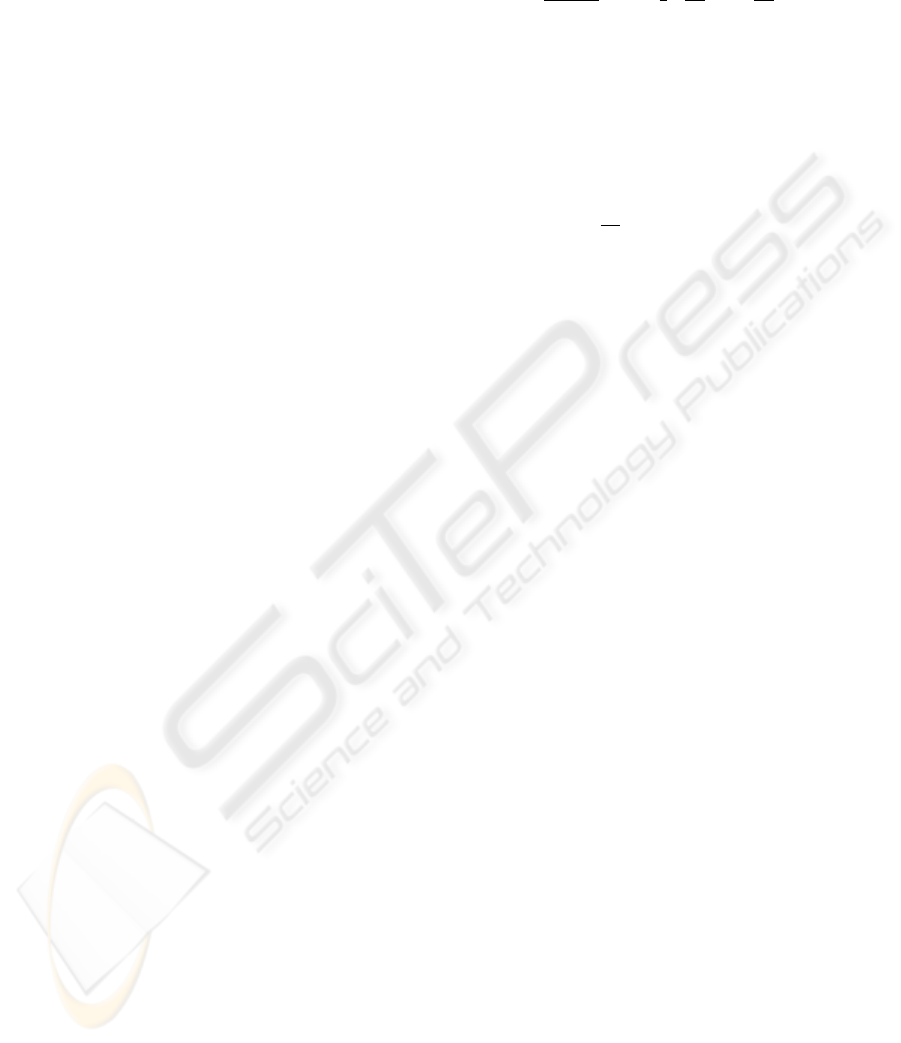
selected three families of general texture descriptors,
Co-occurrence matrix measures, Local Binary Pat-
terns and Gabor Filter Banks. Additionally, we have
computed the presence of shading as a complemen-
tary feature.
4.1 Co-occurrence Matrix
The co-occurrence matrix can be defined as an esti-
mation of the joint probality density function of gray
level pairs in a image (P. Ohanian, 1992). The ele-
ment values in a matrix are bounded from 0 to 1 and
the sum of all element values is:
P(i, j,D,θ) = P(I(l,m) =
i⊗ I(l + Dcos(θ),m+ Dsin(θ)) = j),
where I(l,m) is the gray value at the pixel(l,m), D is
the distance among pixels and θ is the angle of each of
neighbors. The angle orientation θ has been fixed to
be [0
o
,45
o
,90
o
,135
o
], because, according to (Husoy,
1999; P. Ohanian, 1992), it is the minimum set of ori-
entations needed to describe a second-order statistic
measures of texture. After computing this matrix, six
characterizing measures, energy, entropy, the Inverse
Difference Moment, shade, inertia and Promenance
are extracted as defined in (P. Ohanian, 1992). Thus,
a 48 feature space is built for each pixel, since we are
estimating 6 different measures at 4 orientations and
two distances D = [5,8].
4.2 Local Binary Patterns
These feature extractor operators are used to detect
uniform texture patterns in circular neighborhoods
with any quantization of angular space and spatial res-
olution (Ojala and Maenpaa, 2002). They are based
on a circular symmetric neighborhood of P members
of a circle with radius R. Gray level invariance is
achieved when the central pixel g
c
is subtracted to
each neighbor g
p
, assigning to the result 1 if the dif-
ference is positive and 0 if it is negative. Each neigh-
bor is weighted with a 2
p
value. Then, the neighbors
are added, and the result is assigned to the central
pixel.
LBP
R,P
=
P
∑
p=0
s(g
p
− g
c
) · 2
p
With these operators we generate a 3 dimensional
space, by applying a radius of R = [1,2,3] and a
neighborhood of P = [8, 16,24].
4.3 Gabor Filters Bank
The Gabor Filters is an special case of wavelets
(Daugman, 1985; Feichtinger and Strohmer, 1998).
It is a Gaussian g modulated by a complex sinusoid
s. In 2D, a Gabor filter has the following form in the
spatial domain:
h(x,y) =
1
2πσ
x
′
σ
y
′
exp{−
1
2
[(
x
′
σ
x
′
)
2
+ (
y
′
σ
y
′
)
2
]} · s(x, y),
where s(x,y) and the Gaussian rotation are defined as:
s(x,y) = exp[−i2π(Ux+Vy)]
x
′
= xcosθ+ ysinθ, y
′
= −xsinθ+ ycosθ.
x
′
and y
′
represent the spatial coordinates rotated by
an angle θ. σ
x
′
and σ
y
′
are the standard deviations
for the Gaussian envelope. An aspect ratio λ and its
orientation are defined as:
λ =
σ
x
′
σ
y
′
, φ = arctanV/U
where U and V represent the 2D frequencies of the
complex sinusoid.
λ has been fixed to 1 in order to create isotropic
gaussian envelopes, that is, both σ
x
′
and σ
y
′
are equal,
and θ is discarded, θ = 0. The 2D frequency, (U,V)
have been changed to its polar representation F,φ.
Thus, we have created a filter bank using the follow-
ing parameters:
σ
x
= σ
y
= [12.7205,6.3602,3.1801, 1.5901],
φ = [0
o
,45
o
,90
o
,135
o
],
F = [0.0442,0.0884,0.1768, 0.3536],
yielding a 16 dimensional space for each pixel.
4.4 Shading
According to (Gil et al., 2006), one of the main dif-
ferences in the calcified tissue is the shadow which is
appreciated behind it. In order to detect this shadow,
we have performed an accumulative mean on a polar
image. This measure is performed by calculating the
mean, from one pixel to the end of the column. Be-
fore that, we have chosen a threshold to give the same
weight to the tissue and a smaller one to the shadows.
This differs from (Gil et al., 2006), since they want to
achieve a rough classification. This operation gener-
ates one value for each pixel.
As a result of feature extraction process, we ob-
tain a vector of 68 dimensions for each pixel, which
will be used to train the classifier. The main goal of
this is to extract the best of each technique in order to
improve the classification performance.
5 CLASSIFICATION
Once we have reconstructed and characterized the im-
age, we proceed to the classification in order to iden-
tify the different types of plaque. We have estab-
lished 3 classes of tissue: fibrotic plaque, lipid or
VISAPP 2007 - International Conference on Computer Vision Theory and Applications
116