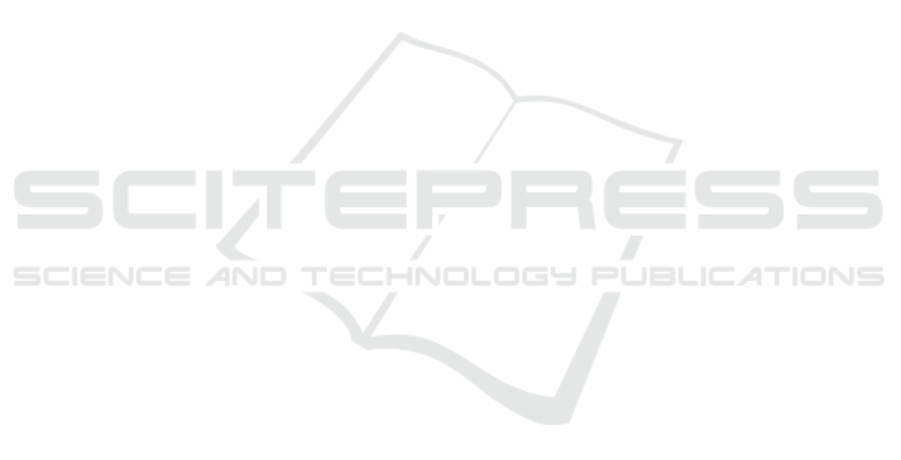
gration. Secondly, there is a requirement of efficient
government, which fosters such an integration, from
planning a survey to publishing its outcomes.
That said, integrating multiple surveys at the data
level is more promising, and integrating disparate data
sources has been widely practiced. For example, var-
ious sources of data, such as geographic information,
can be integrated with surveys (Cooper, 2020). One
can also link spatial data from surveys and databases
for the integration, e.g., health surveys and health
facility databases (Dotse-Gborgbortsi et al., 2020).
Since spatial and temporal information are essential
to population survey data, they are used for testing
the feasibility of direct integration of surveys. They
further provide the mappings between the surveys for
the implementation of the integration.
It is recommended that the data collection and
the reporting systems enable data sharing to improve
the adaptation of integrated surveys (Jacobson and
Teutsch, 2012). As an example, in India, the avail-
ability of raw data and reports of the National Family
Health Survey (NFHS) in the public domain, has im-
proved the uptake of several researchers working with
the data, compared to similar national surveys (Dan-
dona et al., 2016). The NFHS is favorably imple-
mented at the national scale at a higher frequency,
i.e., roughly once in 5 years, aligned with the world-
wide data collection efforts. The NFHS data can
be strategically used with other national and local
surveys to infer health and related socio-economic
factors, even though its focus is on maternal-child
health indicators. Hence, we choose to integrate
the NFHS-4 during 2015-16, the fourth edition of
NFHS (IIPS and MoHFW, 2016), and the Compre-
hensive National Nutrition Survey (CNNS) during
2016-18 (MoHFW, UNICEF and Population Council,
2019). These surveys are conducted by the Ministry
of Health and Family Welfare (MoHFW), Govern-
ment of India (GoI), and implemented by the Ministry
of Statistics and Programme Implementation (Mo-
SPI), GoI. MoSPI provides access to the demographic
survey outcomes. However, studies using the open
data have examined these surveys in a silo, based on
their specific individual goals. There is also prior
work on comparing these surveys, specifically (Rathi
et al., 2018), but not integrating them. An integrated
analysis of pertinent surveys can effectively reduce
the burden of conducting numerous surveys in a pop-
ulous middle-income country like India. Hence, our
goal is to demonstrate a proof-of-concept of a cross-
analysis. Our challenge here lies in the difference in
the granularity of the open data available in the two
chosen surveys, limiting our scope of directly inte-
grating them at the data level. We address this by us-
ing spatial statistics and visualizations.
We focus on mining information on various as-
pects of malnutrition for children under five, in In-
dia, through this integrated study. Under-five stud-
ies are concluding spatial heterogeneity in various
health indicators on malnutrition (Khan and Mohanty,
2018; Puri et al., 2020; Sharma et al., 2020), which
can be exploited. The interest in under-five stud-
ies is due to the persistence of childhood morbid-
ity and mortality in India, as per NFHS-4 (Dhirar
et al., 2018). Wasting has not reduced as much
between NFHS-3 and NFHS-4 findings as stunting.
In the weighted sample taken in CNNS, the preva-
lence of anemia is 40.5% amongst children under five,
with iron-deficiency anemia being the most preva-
lent type (Sarna et al., 2020). The nutritional de-
ficiency affects all age groups, but children under
five, particularly those with severe acute malnutrition
(SAM), have a higher mortality risk from common
childhood illnesses such as diarrhea, pneumonia, and
malaria (UNICEF, 2019). While the infant mortal-
ity rate (IMR) is at 41 per thousand live births, the
under-5-mortality rate (U5MR) is at 50. Childhood
undernutrition accounts for 45% of U5MR alone and
is a crucial public health issue in India. Dietary di-
versification is an additional solution apart from the
focus on infrastructure for food distribution and de-
livery by the government (Dhirar et al., 2018). There
is an emphatic call for more frequent health surveys
to be conducted to continuously monitor the progress
due to such nutrition programs and infrastructural im-
provement, motivating our integrated study.
A fine-grained analysis has been done on the oc-
currence of anemia, stunting, and incomplete immu-
nization in children aged 12-59 months, at district
and individual levels, using NFHS-4 data (Puri et al.,
2020). This study also showed the influence of mater-
nal education on the aforementioned outcomes at the
district level. There is also evidence that there is spa-
tial influence on poor sanitation, which is one of the
causes of stunting in India, where the extreme tem-
perature is a contextual correlate (Bharti et al., 2019).
We use these analyses of the concerned surveys for
identifying contextual factors of malnutrition.
Our novel contribution is in using visual analytics
with spatial context for integrating surveys, namely
NFHS-4 and CNNS in India, for under-five child
malnutrition study. Visual analytics is a data anal-
ysis workflow where one uses visualization to pro-
vide the feedback loop along with other data mining
methods (Keim et al., 2008). We propose a three-
step workflow of (i) using state-wise differences for
determining the feasibility of survey integration, (ii)
a region-based study to identify variables for inte-
GISTAM 2021 - 7th International Conference on Geographical Information Systems Theory, Applications and Management
204