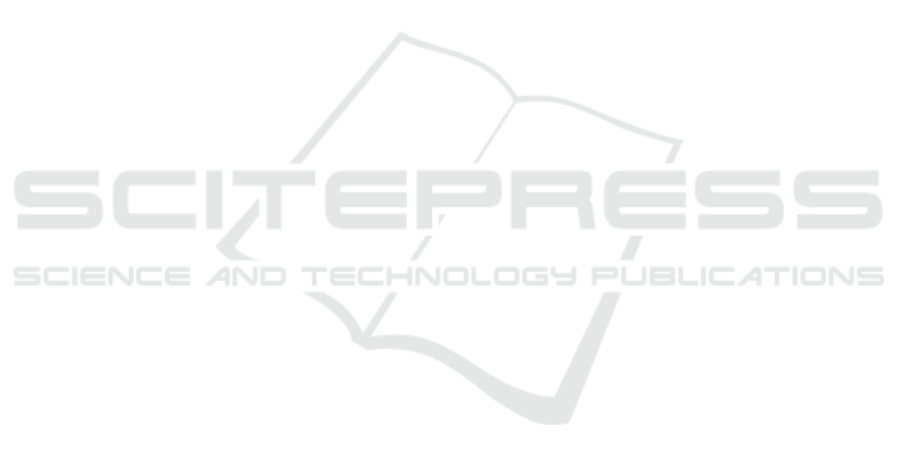
tion systems perspective*. Decision Sciences Journal
of Innovative Education, 4(1):67–85.
Islas, E., P
´
erez, M., Rodriguez, G., Paredes, I.,
´
Avila, I., and
Mendoza, M. (2007). E-learning tools evaluation and
roadmap development for an electrical utility. Jour-
nal of Theoretical and Applied Electronic Commerce
Research, 2(1):63–75.
Kangas, M., Siklander, P., Randolph, J., and Ruokamo, H.
(2017). Teachers’ engagement and students’ satisfac-
tion with a playful learning environment. Teaching
and Teacher Education, 63:274 – 284.
Kori, K., Pedaste, M., Altin, H., T
˜
onisson, E., and Palts,
T. (2016). Factors that influence students’ motiva-
tion to start and to continue studying information tech-
nology in estonia. IEEE Transactions on Education,
59(4):255–262.
Lee, J., Song, H.-D., and Hong, A. (2019). Exploring
factors, and indicators for measuring students’ sus-
tainable engagement in e-learning. Sustainability,
11(4):1–12.
Lerner, J. W. (1989). Educational interventions in learn-
ing disabilities. Journal of the American Academy of
Child & Adolescent Psychiatry, 28(3):326 – 331.
Li, Y., Duan, Y., Fu, Z., and Alford, P. (2012). An empiri-
cal study on behavioural intention to reuse e-learning
systems in rural china. British Journal of Educational
Technology, 43(6):933–948.
Liaw, S.-S., Huang, H.-M., and Chen, G.-D. (2007).
Surveying instructor and learner attitudes toward e-
learning. Computers & Education, 49(4):1066 – 1080.
Limayem, M. and Cheung, C. M. (2008). Understanding in-
formation systems continuance: The case of internet-
based learning technologies. Information & Manage-
ment, 45(4):227 – 232.
Lloyd, N. M., Heffernan, N. T., and Ruiz, C. (2007). Pre-
dicting student engagement in intelligent tutoring sys-
tems using teacher expert knowledge. In The Educa-
tional Data Mining Workshop held at the 13th Con-
ference on Artificial Intelligence in Education, pages
40–49.
Lo, Y.-y., Cooke, N. L., and Starling, A. L. P. (2011). Using
a repeated reading program to improve generalization
of oral reading fluency. Education and Treatment of
Children, 34(1):115–140.
Lyon, G. R., Shaywitz, S. E., and Shaywitz, B. A. (2003). A
definition of dyslexia. Annals of Dyslexia, 53(1):1–14.
Maravanyika, M., Dlodlo, N., and Jere, N. (2017). An
adaptive recommender-system based framework for
personalised teaching and learning on e-learning plat-
forms. In 2017 IST-Africa Week Conference (IST-
Africa), pages 1–9.
Moubayed, A., Injadat, M., Shami, A., and Lutfiyya, H.
(2020). Student engagement level in an e-learning en-
vironment: Clustering using k-means. American Jour-
nal of Distance Education, 34(2):137–156.
Nielsen, J. (1993). Usability Engineering. Academic Press.
Oga, C. and Haron, F. (2012). Life experiences of individ-
uals living with dyslexia in malaysia: A phenomeno-
logical study. Procedia - Social and Behavioral Sci-
ences, 46:1129 – 1133. 4th WORLD CONFERENCE
ON EDUCATIONAL SCIENCES (WCES-2012) 02-
05 February 2012 Barcelona, Spain.
Orfanou, K., Tselios, N., and Katsanos, C. (2015). Per-
ceived usability evaluation of learning management
systems: Empirical evaluation of the system usability
scale. The International Review of Research in Open
and Distributed Learning, 16(2):227–246.
Ozkan, S. and Koseler, R. (2009). Multi-dimensional stu-
dents’ evaluation of e-learning systems in the higher
education context: An empirical investigation. Com-
puters & Education, 53(4):1285 – 1296. Learning
with ICT: New perspectives on help seeking and in-
formation searching.
Pal, D. and Vanijja, V. (2020). Perceived usability evalua-
tion of microsoft teams as an online learning platform
during covid-19 using system usability scale and tech-
nology acceptance model in india. Children and Youth
Services Review, 119:1–12.
Pang, L. and Jen, C. C. (2018). Inclusive dyslexia-friendly
collaborative online learning environment: Malaysia
case study. Education and Information Technologies,
23:1023–1042.
Peres, S. C., Pham, T., and Phillips, R. (2013). Validation
of the system usability scale (sus): Sus in the wild.
Proceedings of the Human Factors and Ergonomics
Society Annual Meeting, 57(1):192–196.
Pickering, J. D. (2017). Measuring learning gain: Compar-
ing anatomy drawing screencasts and paper-based re-
sources. Anatomical Sciences Education, 10(4):307–
316.
Plata, I. T. and Alado, D. B. (2014). Evaluating the per-
ceived usability of virtual learning environment in
teaching ict courses. Globalilluminators. Org, 1:63–
76.
Putnam, C., Puthenmadom, M., Cuerdo, M. A., Wang, W.,
and Paul, N. (2020). Adaptation of the system usabil-
ity scale for user testing with children. CHI EA ’20,
page 1–7, New York, NY, USA. Association for Com-
puting Machinery.
Read, J. C. (2012). Evaluating artefacts with children: Age
and technology effects in the reporting of expected
and experienced fun. In Proceedings of the 14th ACM
International Conference on Multimodal Interaction,
ICMI ’12, page 241–248, New York, NY, USA. Asso-
ciation for Computing Machinery.
Rodrigues, H., Almeida, F., Figueiredo, V., and Lopes, S. L.
(2019). Tracking e-learning through published papers:
A systematic review. Computers & Education, 136:87
– 98.
Sahari, S. H. and Johari, A. (2012). Improvising reading
classes and classroom environment for children with
reading difficulties and dyslexia symptoms. Procedia
- Social and Behavioral Sciences, 38:100 – 107. ASIA
Pacific International Conference on Environment-
Behaviour Studies (AicE-Bs), Grand Margherita Ho-
tel, 7-9 December 2010, Kuching, Sarawak, Malaysia.
Sihombing, J. H., Laksitowening, K. A., and Darwiyanto,
E. (2020). Personalized e-learning content based on
felder-silverman learning style model. In 2020 8th In-
ternational Conference on Information and Commu-
nication Technology (ICoICT), pages 1–6.
CSEDU 2021 - 13th International Conference on Computer Supported Education
218