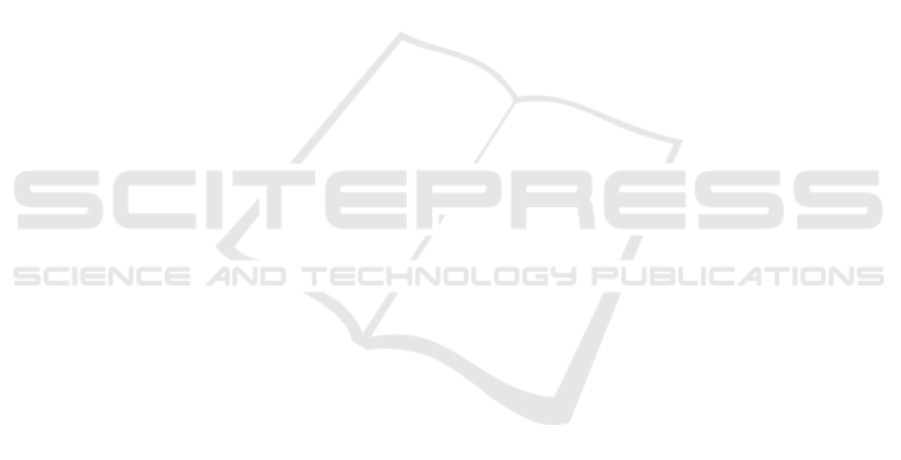
control of the ACC or CACC and not to vehicles capa-
ble of overtaking or lane changes. The impact of AVs
in merging roadways is investigated in (Rios-Torres
and Malikopoulos, 2017). The influence of AVs on
flow stability and throughput is studied in (Talebpour
and Mahmassani, 2016). The studies have shown that
AVs will affect traffic flows in different ways, both
improving or degrading traffic performance. Usu-
ally, it is assumed that all AVs will drive according to
the same driving logic (e.g. (Olia et al., 2018)), and
their impacts are commonly estimated by including
scenarios with increasing market penetration rates of
AVs. Moreover, the approaches taken to model AVs
or automated driving in simulation investigations vary
from study to study.
Microscopic simulation models have been devel-
oped aiming to describe human driving behavior. To
model the driving logic of AVs, these models need to
be modified, extended or replaced. Modifying param-
eters of existing car following models or lane chang-
ing models is a fast and simple approach to model
AV driving logics. This could for example be modi-
fications on distances kept between vehicles, reaction
times, accepted distances for lane changing, acceler-
ation and speed parameters. If a specific feature of
AVs can not be modeled by changing parameter val-
ues, then the models could be extended or replaced.
New or extended models could aim to simulate vehi-
cles sensors, control algorithms or safety features.
Nonetheless, regardless of the approach taken to
model AVs, the calibration of the models is based on
the available data, which currently is limited to exist-
ing partly automated vehicles, and not based on future
automated driving. Therefore, investigations based
on traffic simulation of AVs should consider a range
of possible driving logics for AVs (e.g (Olstam et al.,
2020; Mintsis et al., 2019)).
3 EXPERIMENT SETUP
The purpose of the simulation experiment done in this
study is to enhance the understanding of potential im-
pacts caused by AVs. The simulation experiment has
been delimited to a motorway environment due to the
less challenging conditions they present for first auto-
mated driving systems to consider them within their
operational design domain. The results present the
range of effects on traffic performance caused by dif-
ferent AV mixes, showing the impact of the coex-
istence of different types of AVs alongside human
driven vehicles.
The uncertainties related to the transition to full
vehicle automation and to the evolution of the capa-
bilities of AVs are addressed by including multiple
scenarios in the simulation experiment. The transi-
tion period to full vehicle automation is considered by
creating scenarios with increasing penetration rates
of AVs, that is, the proportion of AVs present in the
traffic flow. This represents the expected gradual in-
crease in the of number of AVs present in roads. Three
AV driving logics are used to represent the evolution
of AVs. These driving logics assume different lev-
els of cautiousness in their driving styles, recognizing
that as the technology that allows automated driving
advances, AVs will become more reliable in regards
to safety. However, the uncertainty does not relate
only on how the technologies will evolve but also on
how fast they will be implemented in vehicles. Thus,
scenarios with all possible combinations of the three
AV driving logics are included, each combination is
a specific AV mix. They represent both a faster and
a slower evolution of AVs as well as the coexistence
of different types of AVs. The results obtained regard-
ing the effects caused by this coexistence are the main
contribution of this simulation experiment.
The simulation experiment is conducted for a Ger-
man representative motorway stretch (Sonnleitner,
2018). The AVs in the simulation are assumed to have
perfect knowledge of the geometry of the motorway.
Some elements of the motorway, like the position of
lanes or traffic signs, are perceived in reality through
sensors on the vehicle, which is assumed to be per-
fect in the simulation. As seen in Table 1, AVs with
different driving logics interact with different number
of vehicles or objects. The accuracy, latency and re-
liability of the perception of other vehicles or objects
depend on whether the perception is done through on
board sensors or through connectivity features. This
perception is assumed to be perfect in the simulation
and with zero latency, and how it is accomplished is
implicitly included in the driving logics.
Future motorways might support automated driv-
ing by including digital infrastructure which will pro-
vide static and dynamic information to the AVs. The
infrastructure support for automated driving (ISAD)
(Carreras et al., 2018) considered in the simulation
experiment is ISAD-level D or E, which corresponds
to a conventional infrastructure with little support for
automated driving. The vehicles in the simulation per-
ceive different number of leading or trailing vehicles,
as well as immediate vehicles in target lanes for lane
changing maneuvers, they don’t have additional in-
formation about the traffic conditions elsewhere along
their routes. Since there is no support from the infras-
tructure included in the motorway model, all dynamic
driving tasks are handled by the driving logics.
VEHITS 2021 - 7th International Conference on Vehicle Technology and Intelligent Transport Systems
144