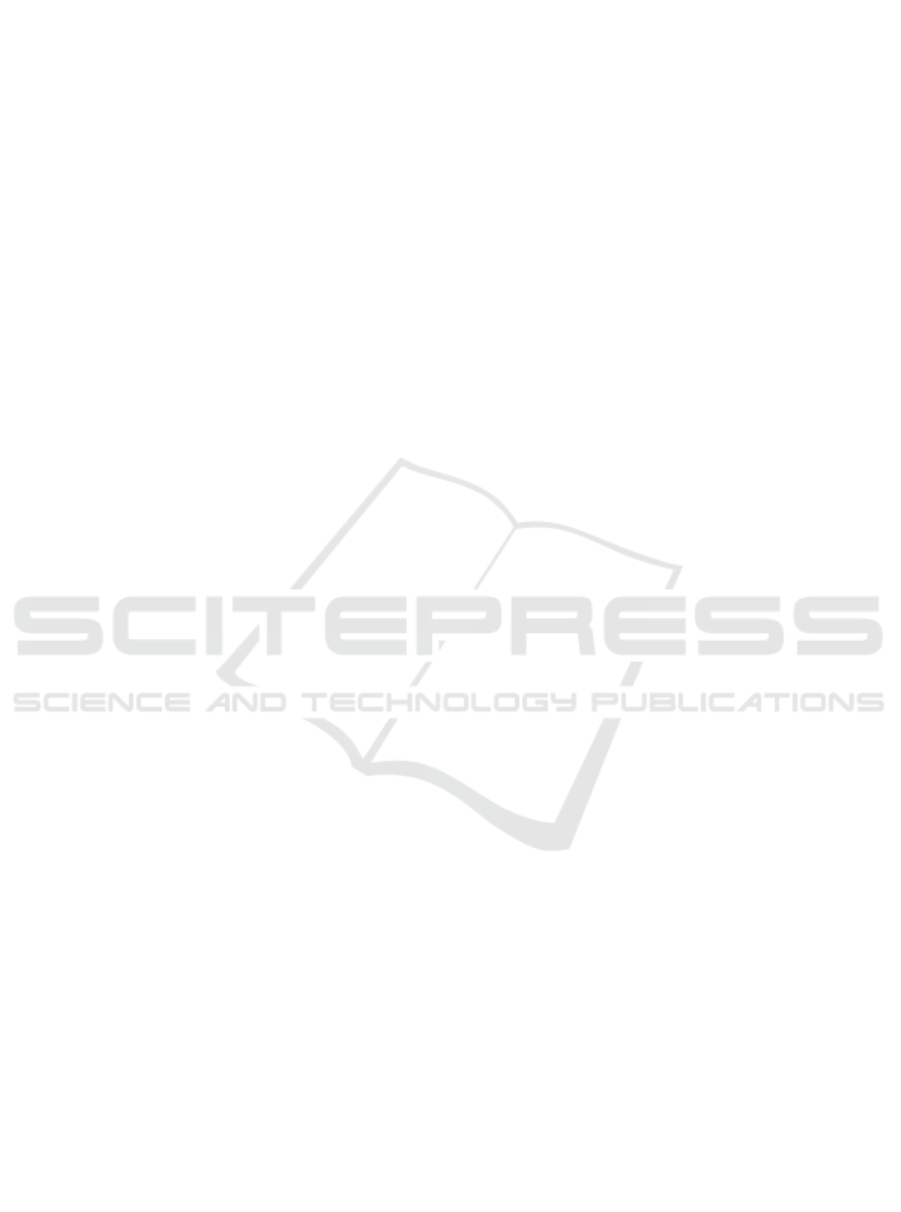
ACKNOWLEDGEMENTS
We thank all survey respondents for participation and
sharing their opinions on aspects referring to their ac-
ceptance of lifelogging technologies in their everyday
living. Kind thanks also to Linda Engelmann for the
assistance in data collection. This work resulted from
the project PAAL (Privacy Aware and Acceptable
Lifelogging services for older and frail people) and
was funded by the German Federal Ministry of Edu-
cation and Research (16SV7955).
REFERENCES
Ajzen, I., 1991. The theory of planned behavior. Organiza-
tional behavior and human decision processes, 50(2),
179–211.
Arber, S., Vandrevala, T., Daly, T., Hampson, S., 2008. Un-
derstanding gender differences in older people's atti-
tudes towards life-prolonging medical technologies.
Journal of Aging Studies, 22(4), 366–375.
Beier, G., 1999. Kontrollüberzeugungen im Umgang mit
Technik [Control convictions in dealing with
technology]. Report Psychologie, 9, 684–693.
Broos, A., 2005. Gender and information and communica-
tion technologies (ICT) anxiety: Male self-assurance
and female hesitation. CyberPsychology & Behavior,
8(1), 21–31.
Caprani, N., Piasek, P., Gurrin, C., O'Connor, N. E., Irving,
K., Smeaton, A. F., 2014. Life-long collections: moti-
vations and the implications for lifelogging with mobile
devices. International Journal of Mobile Human Com-
puter Interaction (IJMHCI), 6(1), 15–36.
Chang, Y. S., Yang, C., 2013. Why do we blog? From the
perspectives of technology acceptance and media
choice factors. Behaviour & Information Technology,
32(4), 371–386.
Chen, Y., Jones, G. J.F., 2012. What do people want from
their lifelogs? In: 6th Irish Human Computer Interac-
tion Conference (iHCI2012), 20-21 June 2012, Galway,
Ireland.
Cho, Y., Park, J., Park, S., Jung, E. S. (2017). Technology
acceptance modeling based on user experience for au-
tonomous vehicles. Journal of the Ergonomics Society
of Korea, 36(2), 87–108.
Climent-Pérez, P., Spinsante, S., Mihailidis, A., Florez-Re-
vuelta, F., 2020. A review on video-based active and
assisted living technologies for automated lifelogging.
Expert Systems with Applications, 139, 112847.
Cohen, J. (1988). Statistical power analysis for the behav-
ioral sciences, Lawrence Erlbaum Associates. New
York, 2
nd
edition.
Davis, F. D., 1989. Perceived Usefulness, Perceived Ease
of Use, and User Acceptance of Information Technol-
ogy. MIS Quarterly, 13(3), 319–340.
Durndell, A., Haag, Z., 2002. Computer self-efficacy, com-
puter anxiety, attitudes towards the Internet and re-
ported experience with the Internet, by gender, in an
East European sample. Computers in Human Behavior,
18(5), 521–535.
Fishbein, M. and Ajzen, I., 1975. Belief, Attitude, Intention
and Behavior: An Introduction to Theory and Research.
Addison-Wesley Publishing Company, Inc., Reading,
MA, USA.
Gurrin, C., Smeaton, A. F., Doherty, A. R., 2014. Lifelog-
ging: Personal big data. Foundations and Trends in In-
formation Retrieval, 8(1), 1–125.
Harvey, M., Langheinrich, M., Ward, G., 2016. Remember-
ing through lifelogging: A survey of human memory
augmentation. Pervasive and Mobile Computing, 27,
14–26.
Hayes, T. L., Abendroth, F., Adami, A., Pavel, M.,
Zitzelberger, T. A., Kaye, J. A., 2008. Unobtrusive as-
sessment of activity patterns associated with mild cog-
nitive impairment. Alzheimer's & Dementia, 4(6), 395–
405.
Heerink, M., 2011. Exploring the influence of age, gender,
education and computer experience on robot ac-
ceptance by older adults. In 2011 6th ACM/IEEE Inter-
national Conference on Human-Robot Interaction
(HRI), pp. 147-148. IEEE.
Jalal, A., Kamal, S., Kim, D., 2014. A depth video sensor-
based life-logging human activity recognition system
for elderly care in smart indoor environments. Sensors,
14(7), 11735–11759.
Kelly, P., Marshall, S. J., Badland, H., Kerr, J., Oliver, M.,
Doherty, A. R., Foster, C., 2013. An ethical framework
for automated, wearable cameras in health behavior re-
search. American journal of preventive medicine, 44(3),
314–319.
Lidynia C., Schomakers EM., Ziefle M., 2019. What Are
You Waiting for? – Perceived Barriers to the Adoption
of Fitness-Applications and Wearables. In: Ahram T.
(eds) Advances in Human Factors in Wearable Tech-
nologies and Game Design. AHFE 2018. Advances in
Intelligent Systems and Computing, vol 795. Springer,
Cham.
McAdams E., Krupaviciute A., Gehin C., Dittmar A., Del-
homme G., Rubel P., Fayn J., McLaughlin J., 2011.
Wearable Electronic Systems: Applications to Medical
Diagnostics/Monitoring. In: Bonfiglio A., De Rossi D.
(eds.) Wearable Monitoring Systems. Springer, Boston.
Miller, L. M. S., Bell, R. A., 2012. Online health infor-
mation seeking: the influence of age, information trust-
worthiness, and search challenges. Journal of aging and
health, 24(3), 525–541.
Morganti, L., Riva, G., Bonfiglio, S., Gaggioli, A., 2013.
Building collective memories on the web: the Nostalgia
Bits project. International Journal of Web Based Com-
munities, 9(1), 83–104.
Mubashir, M., Shao, L., Seed, L., 2013. A survey on fall
detection: Principles and approaches. Neurocomputing,
100, 144–152.
ICT4AWE 2021 - 7th International Conference on Information and Communication Technologies for Ageing Well and e-Health
34