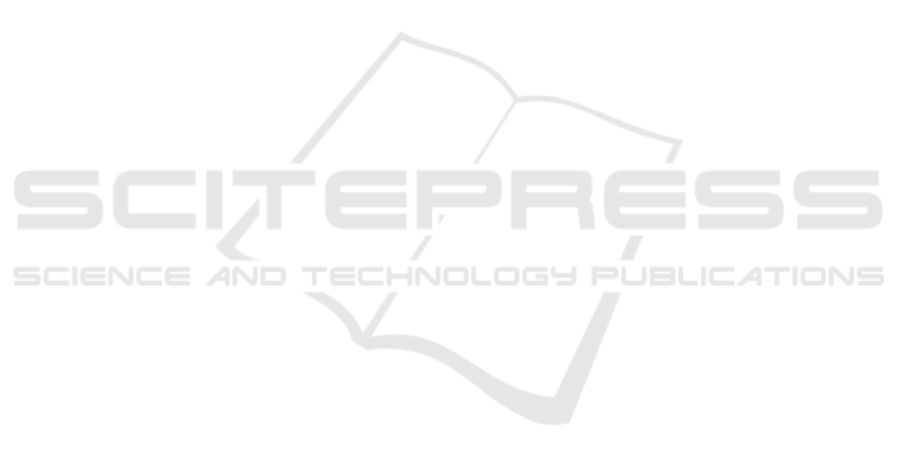
Barak, M., Hussein-Farraj, R., and Dori, Y. J. (2016).
On-campus or online: examining self-regulation and
cognitive transfer skills in different learning settings.
International Journal of Educational Technology in
Higher Education, 13(1):1–18.
Brockett, R. G. and Hiemstra, R. (1991). Self-direction in
adult learning: Perspectives on theory, research, and
practice. Routledge.
Deyo, Z. M., Huynh, D., Rochester, C., Sturpe, D. A., and
Kiser, K. (2011). Readiness for self-directed learning
and academic performance in an abilities laboratory
course. American Journal of Pharmaceutical Educa-
tion, 75(2).
Fisher, M., King, J., and Tague, G. (2001). Development
of a self-directed learning readiness scale for nursing
education. Nurse education today, 21(7):516–525.
Fisher, M. J. and King, J. (2010). The self-directed learn-
ing readiness scale for nursing education revisited: A
confirmatory factor analysis. Nurse education today,
30(1):44–48.
Garrison, D. R. (1997). Self-directed learning: Toward
a comprehensive model. Adult education quarterly,
48(1):18–33.
Garrison, D. R. (2003). Self-directed learning and distance
education. Handbook of distance education, pages
161–168.
Guglielmino, L. M. (1978). Development of the self-
directed learning readiness scale. PhD thesis, Pro-
Quest Information & Learning.
Guglielmino, L. M. (2013). The case for promoting self-
directed learning in formal educational institutions.
SA-eDUC, 10(2).
Guglielmino, L. M. and Guglielmino, P. J. (2001). Mov-
ing toward a distributed learning model based on
self-managed learning. S.A.M.Advanced Management
Journal, 66(3).
Guglielmino, L. M. and Guglielmino, P. J. (2004). Becom-
ing a more self-directed learner. Getting the most from
online learning: A learner’s guide, pages 25–38.
Guglielmino, L. M. and Guglielmino, P. J. (2013). Learning
preference assessment. www.lpasdlrs.com. accessed
3.3.2020.
Hakala, I., H
¨
arm
¨
anmaa, T., and Laine, S. (2016). Learn-
ing styles module as a part of a virtual campus. In
2016 IEEE Global Engineering Education Confer-
ence (EDUCON), pages 425–433.
Hakala, I. and Myllym
¨
aki, M. (2016). From face-to-face to
blended learning using ict. In 2016 IEEE Global En-
gineering Education Conference (EDUCON), pages
409–418.
Hassan, A. M. (1981). An investigation of the learning
projects among adults of high and low readiness for
self-direction in learning. PhD thesis, Iowa State Uni-
versity.
Heo, J. and Han, S. (2018). Effects of motivation, academic
stress and age in predicting self-directed learning
readiness (sdlr): Focused on online college students.
Education and Information Technologies, 23(1):61–
71.
Hiemstra, R. and Brockett, R. G. (2012). Reframing the
meaning of self-directed learning: An updated model.
In Adult Education Research Conference.
Jiusto, S. and DiBiasio, D. (2006). Experiential learning
environments: Do they prepare our students to be self-
directed, life-long learners? Journal of Engineering
Education, 95(3):195–204.
Kim, R., Olfman, L., Ryan, T., and Eryilmaz, E. (2014).
Leveraging a personalized system to improve self-
directed learning in online educational environments.
Computers & Education, 70:150–160.
Knowles, M. S. (1975). Self-Directed Learning: A Guide
for Learners and Teachers. Association Press, New
York, NY, USA.
Knowles, M. S. (1980). The Modern Practice of Adult Ed-
ucation: From Pedagogy to Andragogy: Revised and
Updates. Cambridge Adult Education, Prentice Hall
Regents.
Litzinger, T. A., Wise, J. C., and Lee, S. H. (2005). Self-
directed learning readiness among engineering under-
graduate students. Journal of Engineering Education,
94(2):215–221.
Loizzo, J., Ertmer, P. A., Watson, W. R., and Watson, S. L.
(2017). Adult mooc learners as self-directed: Percep-
tions of motivation, success, and completion. Online
Learning, 21(2).
Moore, M. (1986). Self-directed learning and distance ed-
ucation. International Journal of E-Learning & Dis-
tance Education/Revue internationale du e-learning et
la formation
`
a distance, 1(1):7–24.
Rashid, T. and Asghar, H. M. (2016). Technology use, self-
directed learning, student engagement and academic
performance: Examining the interrelations. Comput-
ers in Human Behavior, 63:604–612.
Song, L. and Hill, J. R. (2007). A conceptual model for
understanding self-directed learning in online envi-
ronments. Journal of Interactive Online Learning,
6(1):27–42.
Stewart, R. A. (2007). Investigating the link between self
directed learning readiness and project-based learning
outcomes: the case of international masters students in
an engineering management course. European Jour-
nal of Engineering Education, 32(4):453–465.
Sumuer, E. (2018). Factors related to college students’ self-
directed learning with technology. Australasian Jour-
nal of Educational Technology, 34(4).
Tang, Y. and Tseng, H. W. (2013). Distance learners’ self-
efficacy and information literacy skills. The journal of
academic librarianship, 39(6):517–521.
Tough, A. (1979). The adult’s learning projects: A fresh
approach to theory and practice in adult learning (2nd
ed.). Learning Concepts.
CSEDU 2021 - 13th International Conference on Computer Supported Education
446