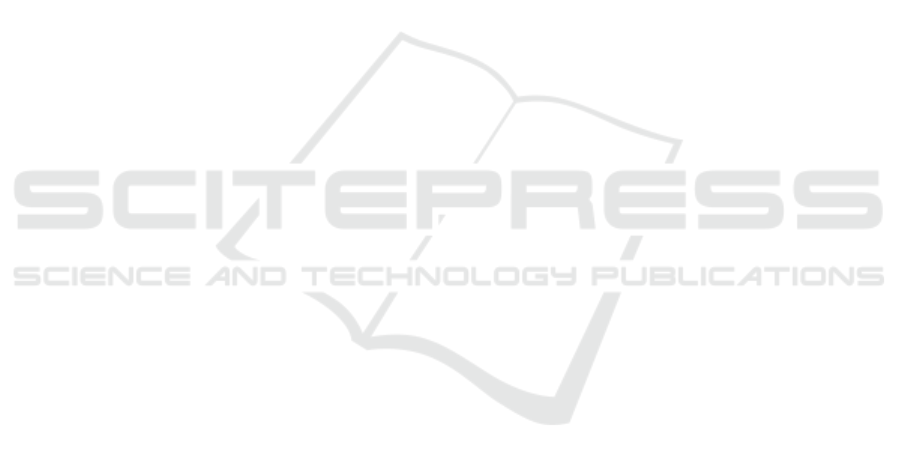
ACKNOWLEDGEMENTS
Intracranial data was recorded at the Queen Eliza-
beth University Hospital Birmingham and provided
by Simon Hanslmayr, Bernhard Staresina, Maria
Wimber, George Parish, Marije Ter Wal, Frederic
Roux, Ramesh Chelvarajah, David Rollings and Vi-
jay Sawlani.
REFERENCES
Buzs
´
aki, G., Anastassiou, C. A., and Koch, C. (2012).
The origin of extracellular fields and currents - EEG,
ECoG, LFP and spikes. Nature Reviews, Neuro-
science, 13:407–420.
Einevoll, G. T., Kayser, C., Logothetis, N. K., and Panzeri,
S. (2013). Modelling and analysis of local field poten-
tials for studying the function of cortical circuits. Nat
Rev Neurosci, 14(11):770–785.
Eleftheriou, E. and Falconer, D. (1986). Tracking proper-
ties and steady-state performance of rls adaptive filter
algorithms. IEEE Transactions on Acoustics, Speech,
and Signal Processing, 34(5):1097–1110.
Hagen, E., Dahmen, D., Stavrinou, M. L., Lind
´
en, H., Tet-
zlaff, T., van Albada, S. J., Gr
¨
un, S., Diesmann, M.,
and Einevoll, G. T. (2016). Hybrid scheme for model-
ing local field potentials from point-neuron networks.
Cerebral Cortex, 26(12):4461–4496.
Hjorth, B. (1975). An on-line transformation of EEG
scalp potentials into orthogonal source derivations.
Electroencephalography and Clinical Neurophysiol-
ogy, 39(5):526 – 530.
Hofmanis, J., Caspary, O., Louis-Dorr, V., and Maillard, L.
(2011). Automatic depth electrode localization in in-
tracranial space. In 4th International Conference on
Bio-inspired Systems and Signal Processing, Biosig-
nals 2011, Rome, Italie.
Hu, S., Stead, M., and Worrel, G. (2007). Automatic iden-
tification and removal of scalp reference signal for
intracranial EEGs based on Independent Component
Analysis. IEEE Transactions on Biomedical Engi-
neering, 54(9):1560–1572.
Hu, S., Stead, M., and Worrell, G. (2008). Removal of
scalp reference signal and line noise for intracranial
EEGs. In Networking, Sensing and Control, 2008. IC-
NSC 2008. IEEE International Conference on, pages
1486–1491.
Kajikawa, Y. and Schroeder, C. E. (2011). How local is the
local field potential? Neuron, (72).
Le Cam, S., Tran, H., Ranta, R., and Louis-Dorr, V. (2019).
Simultaneous separation and sorting of extra-cellular
spikes. In 9th International IEEE/EMBS Conference
on Neural Engineering, NER 2019, San Francisco,
USA.
Libenson, M. (2012). Practical approach to electroen-
cephalography. Elsevier Health Sciences.
Lind
´
en, H., Pettersen, K. H., and Einevoll, G. T. (2010). In-
trinsic dendritic filtering gives low-pass power spec-
tra of local field potentials. Journal of computational
neuroscience, 29(3):423–444.
Madhu, N., Ranta, R., Maillard, L., and Koessler, L. (2012).
A unified treatment of the reference estimation prob-
lem in depth EEG recordings. Med. Biol. Eng. Com-
put., 50(10):1003–1015.
Mitzdorf, U. (1985). Current source-density method and
application in cat cerebral cortex: investigation of
evoked potentials and EEG phenomena. Physiologi-
cal reviews, 65(1):37–100.
Nelson, M. J., Pouget, P., Nilsen, E. A., Patten, C. D.,
and Schall, J. D. (2008). Review of signal distortion
through metal microelectrode recording circuits and
filters. Journal of Neuroscience Methods, 169:141–
157.
Quian Quiroga, R., Nadasdy, Z., and Ben-Shaul, Y.
(2004). Unsupervised spike detection and sorting with
wavelets and superparamagnetic clustering. Neural
computation, 16(8):1661–1687.
Ranta, R., Salido-Ruiz, R., and Louis-Dorr, V. (2010).
Reference estimation in EEG recordings. In 32nd
Ann. Int. Conf. of the IEEE-EMBS, Buenos Aires, Ar-
gentina.
Rossion, B., Retter, T., and Liu-Shuang, J. (2020). Under-
standing human individuation of unfamiliar faces with
oddball fast periodic visual stimulation and electroen-
cephalography. European Journal of Neuroscience
(review).
Rossion, B., Torfs, K., Jacques, C., and Liu-Shuang, J.
(2015). Fast periodic presentation of natural im-
ages reveals a robust face-selective electrophysiolog-
ical response in the human brain. Journal of Vision,
15(1):18–18.
Salido-Ruiz, R. A., Ranta, R., Korats, G., Le Cam, S.,
Koessler, L., and Louis-Dorr, V. (2019). A unified
weighted minimum norm solution for the reference
inverse problem in eeg. Computers in Biology and
Medicine, 115:103510.
Tran, H., Ranta, R., Le Cam, S., and Louis-Dorr, V. (2020).
Fast simulation of extracellular action potential signa-
tures based on a morphological filtering approxima-
tion. Journal of Computational Neuroscience, 48.
Yao, D. (2001). A method to standardize a reference of
scalp EEG recordings to a point at infinity. Physiolog-
ical Measurement, 22:693–711.
Zanos, T. P., Mineault, P. J., and Pack, C. C. (2011). Re-
moval of spurious correlations between spikes and lo-
cal field potentials. J Neurophysiology, 105:474–486.
BIOSIGNALS 2021 - 14th International Conference on Bio-inspired Systems and Signal Processing
350