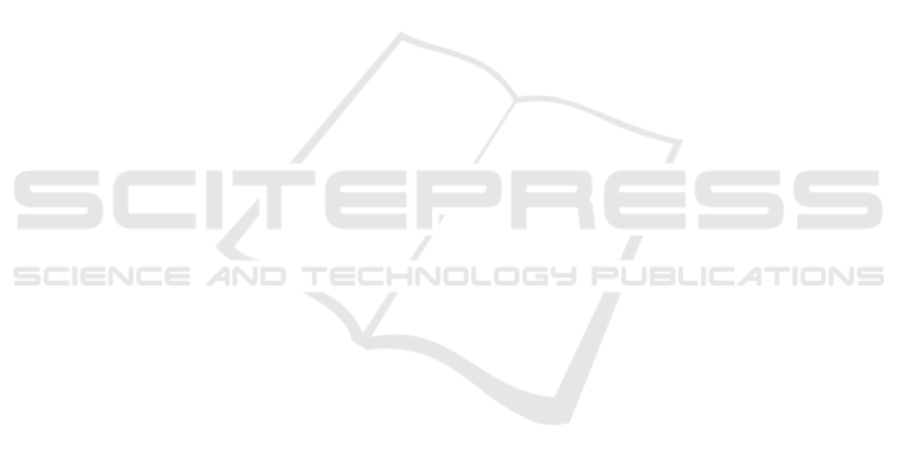
providers or caregivers. Data from the clinical app,
home app, as well as from the wearable device are
stored on the database to be made available to the
other applications in the system (Fig. 1). For in-
stance, plans generated on the clinical app by health-
care providers are available for the elderly to follow
in the mobile app. Cloud Firestore stores the profile
data, such as height or clinical condition, the answers
to the questionnaires for risk assessment, plans cre-
ated by the healthcare providers, and session and ex-
ercise data detailing the measurements of individual
exercises. Besides allowing developers to synchro-
nize data across applications, it enable us to monitor
the elderly during the trials, and to keep a detailed log
of participants measurements for further analysis of
the trials results.
2.5 Trial Monitor
Trial Monitor is a web application created with the
purpose of supporting researchers monitoring partici-
pants remotely during field trials (Vasconcelos et al.,
2019). The tool enables researchers to create per-
sonalized data visualization dashboards with the data
generated by participants during the trials (Fig. 7).
The web application connects to the data storage (i.e.
Cloud Firestore) to retrieve users data, and generates
visualizations for health providers to follow the el-
derly remotely.
The platform enables nursers to understand how
participants are progressing during the trial, by pro-
viding a system for monitoring the results of the
prescribed exercises and how they evolve over time.
Healthcare providers can use the platform to visual-
ize and analyse the results of the Otago exercises, or
the fall risk assessment questionnaires. The platform
displays the number of sessions completed and the re-
sults from individual exercises (e.g. number of repe-
titions, range of motion). Moreover, the platform al-
lows researchers to easily export data (i.e. CSV file)
from the system for further analysis after the end of
the trial.
3 VALIDATION TRIALS
We have produced 20 units of system to be used dur-
ing the validation trials. The participants are being
recruited. The trials to be conducted will allow to
evaluate the performance of the FD and to assess the
fall risk of the elder population in the North region of
Portugal. The trials will also provide insights on the
effectiveness of the FP exercises using this technolog-
ical platform.
The study will bring the objective measure of fall
risk factors and movement-based metrics extracted
during the Otago exercises. The technological plat-
form allows the centralization of all relevant variables
in a unified and secured database, that is accessed
through a web portal, that will be available for the
healthcare providers that will supervise the study. Be-
sides the aforementioned variables retrieved by the
Clinical app, i.e., personal profile, medical conditions,
medication, answer to the questionnaires, and scores
of the three functional tests, the Home app will allow
to measure range of motion, number of repetitions and
duration of ascending and descending movements for
eight exercises of the OEP, i.e., knee flexion, knee ex-
tension, hip abduction, knee bending, toe raises, calf
raises, sit to stand, and one leg standing.
4 CONCLUSION
The use of technological devices for early detection
and prevention of falls is a key strategy to minimize
the consequences of this event, namely the perma-
nence on the ground for long periods after the fall and
hypothermia, which often leads to the death of the el-
derly. This reality is verified not only in the elder’s
home but also in nursing homes. To respond to this,
the scientific production in this area has been prolifer-
ating, both in the technological sector and in the aca-
demic and clinical context. The synergies created by
the joint work of these areas, allow this project to con-
tribute to the development of more effective strategies
for prevention, reduction of falls and their physical,
psychological and economic consequences.
In technological terms, we consider that the tech-
nological literacy of each participant could impact the
way each one will use the platform and we are aware
that some limitations may raise during the course of
the eight weeks of intervention, considering that in
some of the sessions the elderly will perform the ex-
ercises alone at home. To try to overcome these
limitations, we foresee frequent contacts between the
healthcare providers and the elderly, as well as remote
guidance whenever possible.
At the end of the validation study, we will have
a system for fall management composed of a wear-
able device and a bundle of applications. The system
can be used to i) pervasively detect falls and assess
fall risk factors in daily life; ii) monitor movements
during fall risk assessment tests to estimate a risk of
falling using a desktop application, with the supervi-
sion of a healthcare provider; iii) monitor movements
during the execution of fall prevention exercises us-
ing by a tablet application. The trials conducted will
BIOSIGNALS 2021 - 14th International Conference on Bio-inspired Systems and Signal Processing
324