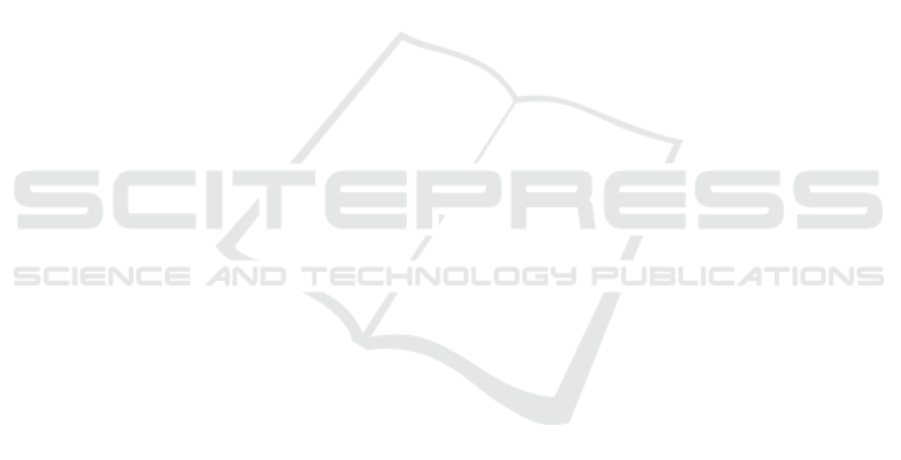
reflected in the SCR. By estimating the causal rela-
tionships between the variables that serve as clues to
inferring the changes in a person’s mental state, we
may be able to compare the inferred changes while
eliminating various convoluted factors in future work.
5 CONCLUSION
The aim of this study was to investigate whether an
active attitude can be induced in a person by apply-
ing the framework of work engagement enhancement
to a cooperative decision-making task with agents.
We conducted experiments to evaluate the effect of
an agent behavior directed toward increasing personal
resources for improving the active attitude of the par-
ticipants. In the experiment, the participants were
asked to plan a two-day trip to a fictitious city with
two agents (an expert agent and a mediator agent).
There were three groups; PR-group: the mediator
agent serves to increase personal resources, JR-group:
the mediator agent serves to increase job resources,
and NA-group: no mediator agent is involved. From
the results, we suggest that the mediator agent can in-
duce and maintain the participant’s active attitude to-
ward the task and the agents by encouraging the par-
ticipant to increase his/her personal resources. In fu-
ture work, we will consider a method for analyzing
the detailed inner state changes of the participants in-
volved in the task.
ACKNOWLEDGEMENTS
This research is supported by Grant-in-Aid for Young
Scientists (B) (KAKENHI No. 16K21113), and
Grant-in-Aid for Scientific Research on Innovative
Areas (KAKENHI No. 26118002) from the Ministry
of Education, Culture, Sports, Science and Technol-
ogy of Japan.
REFERENCES
Andr
´
e, E., Rist, T., Van Mulken, S., Klesen, M., and Baldes,
S. (2000). The automated design of believable di-
alogues for animated presentation teams. Embodied
conversational agents, pages 220–255.
Bakker, A. B., Demerouti, E., and Verbeke, W. (2004).
Using the job demands-resources model to predict
burnout and performance. Human Resource Manage-
ment: Published in Cooperation with the School of
Business Administration, The University of Michigan
and in alliance with the Society of Human Resources
Management, 43(1):83–104.
Bechade, L., Dubuisson Duplessis, G., Sehili, M., and Dev-
illers, L. (2015). Behavioral and emotional spoken
cues related to mental states in human-robot social in-
teraction. In Proceedings of the 2015 ACM on Interna-
tional Conference on Multimodal Interaction, pages
347–350. ACM.
Chi, M. T. and Wylie, R. (2014). The icap framework: Link-
ing cognitive engagement to active learning outcomes.
Educational psychologist, 49(4):219–243.
Dennett, D. C. (1988). The intentional stance in theory and
practice.
Gratch, J., Wang, N., Gerten, J., Fast, E., and Duffy, R.
(2007). Creating rapport with virtual agents. In In-
ternational Workshop on Intelligent Virtual Agents,
pages 125–138. Springer.
Kotze, M. (2018). How job resources and personal
resources influence work engagement and burnout.
African Journal of Economic and Management Stud-
ies, 9(2):148–164.
Misu, T., Sugiura, K., Kawahara, T., Ohtake, K., Hori, C.,
Kashioka, H., Kawai, H., and Nakamura, S. (2011).
Modeling spoken decision support dialogue and opti-
mization of its dialogue strategy. ACM Transactions
on Speech and Language Processing (TSLP), 7(3):10.
Ohmoto, Y., Kataoka, M., and Nishida, T. (2014). The ef-
fect of convergent interaction using subjective opin-
ions in the decision-making process. In Proceedings
of the Annual Meeting of the Cognitive Science Soci-
ety, volume 36.
Ohmoto, Y., Ueno, S., and Nishida, T. (2017). Effect of an
agent’s contingent responses on maintaining an inten-
tional stance. In Proceedings of the 5th International
Conference on Human Agent Interaction, pages 277–
285. ACM.
Ono, T., Ichijo, T., and Munekata, N. (2016). Emergence
of joint attention between two robots and human us-
ing communication activity caused by synchronous
behaviors. In Robot and Human Interactive Com-
munication (RO-MAN), 2016 25th IEEE International
Symposium on, pages 1187–1190. IEEE.
Raux, A., Langner, B., Bohus, D., Black, A. W., and Es-
kenazi, M. (2005). Let’s go public! taking a spoken
dialog system to the real world. In Ninth European
Conference on Speech Communication and Technol-
ogy.
Russell, S. and Norvig, P. (2002). Artificial intelligence: a
modern approach.
Schaufeli, W. B. and Bakker, A. B. (2004). Job demands,
job resources, and their relationship with burnout and
engagement: A multi-sample study. Journal of Or-
ganizational Behavior: The International Journal of
Industrial, Occupational and Organizational Psychol-
ogy and Behavior, 25(3):293–315.
Walster, E. and Festinger, L. (1962). The effectiveness of”
overheard” persuasive communications. The Journal
of Abnormal and Social Psychology, 65(6):395.
Wooldridge, M. (2009). An introduction to multiagent sys-
tems. John Wiley & Sons.
Xanthopoulou, D., Bakker, A. B., Demerouti, E., and
Schaufeli, W. B. (2009). Reciprocal relation-
ships between job resources, personal resources, and
work engagement. Journal of Vocational behavior,
74(3):235–244.
ICAART 2021 - 13th International Conference on Agents and Artificial Intelligence
336