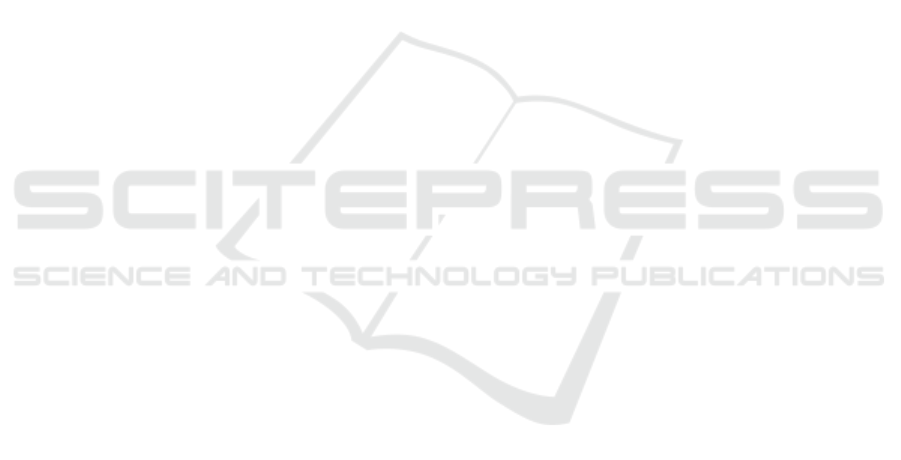
adults: Evidence from world health organization study
on global ageing and adult health. Social Science &
Medicine, 247:112821.
Levy, E., L
´
evy, P., Le, C., et al. (1995). The economic cost
of obesity: the French situation. International Journal
of Obesity and Related Metabolic Disorders: Journal
of the International Association for the Study of Obe-
sity, 19(11):788–792.
Manson, J., Skerrett, P., Greenland, P., et al. (2004). The es-
calating pandemics of obesity and sedentary lifestyle:
a call to action for clinicians. Archives of Internal
Medicine, 164(3):249–258.
Ministerio de Salud, Chile (2017). Base de datos. http://
epi.minsal.cl/bases-de-datos/. Online; accessed 3 oct
2020.
Ministerio de Salud, Colombia (2015). Gesti
´
on
del conocimiento y fuentes de informaci
´
on.
https://www.minsalud.gov.co/salud/publica/
epidemiologia/Paginas/gestion-del-conocimiento-y-
fuentes-de-informacion.aspx. Online; accessed 3 oct
2020.
Mitchell, N., Catenacci, V., Wyatt, H., et al. (2011). Obe-
sity: overview of an epidemic. Psychiatric Clinics,
34(4):717–732.
National Institutes of Health (1998). Clinical guidelines for
the identification, evaluation, and treatment of over-
weight and obesity in adults - the evidence report.
Obesity Reviews, 6(2):51S–209S.
NCD Risk Factor Collaboration (NCD-RisC) (2016).
Trends in adult body-mass index in 200 countries from
1975 to 2014: a pooled analysis of 1698 population-
based measurement studies with 19.2 million partici-
pants. The Lancet, 387(10026):1377–1396.
NCD Risk Factor Collaboration (NCD-RisC) (2017).
Worldwide trends in body-mass index, underweight,
overweight, and obesity from 1975 to 2016: a pooled
analysis of 2416 population-based measurement stud-
ies in 128.9 million children, adolescents, and adults.
The Lancet, 390(10113):2627–2642.
Ogden, C., Carroll, M., Curtin, L., et al. (2006). Prevalence
of overweight and obesity in the United States, 1999-
2004. JAMA, 295(13):1549–55.
Okorodudu, D., Jumean, M., Montori, V., et al. (2010). Di-
agnostic performance of body mass index to identify
obesity as defined by body adiposity: a systematic re-
view and meta-analysis. International Journal of Obe-
sity, 34(5):791–799.
Olariu, E., Cadwell, K., Hancock, E., et al. (2017). Current
recommendations on the estimation of transition prob-
abilities in markov cohort models for use in health
care decision-making: a targeted literature review.
ClinicoEconomics and Outcomes Research: CEOR,
9:537–546.
Orpana, H., Tremblay, M., and Fin
`
es, P. (2006). Trends
in Weight Change Among Canadian Adults: Evidence
from 1996/1997 to 2004/2005 National Population
Health Survey. Citeseer.
Penman, A. and Johnson, W. (2006). The changing shape
of the body mass index distribution curve in the popu-
lation: implications for public health policy to reduce
the prevalence of adult obesity. Preventing Chronic
Disease, 3(3).
Power, C., Lake, J., and Cole, T. (1997). Body mass index
and height from childhood to adulthood in the 1958
british born cohort. The American Journal of Clinical
Nutrition, 66(5):1094–1101.
Secretar
´
ıa de Gobierno de Salud, Argentina
(2019). 2° Encuesta Nacional de Nutrici
´
on
y Salud ENNYS 2. Resumen ejecutivo.
https://cesni-biblioteca.org/2-encuesta-nacional-
de-nutricion-y-salud-ennys-2-resumen-ejecutivo/.
Online; accessed 3 oct 2020.
Srinivasan, S., Bao, W., Wattigney, W., et al. (1996). Ado-
lescent overweight is associated with adult overweight
and related multiple cardiovascular risk factors: the
bogalusa heart study. Metabolism, 45(2):235–240.
Sturm, R. (2002). The effects of obesity, smoking, and
drinking on medical problems and costs. Health Af-
fairs, 21(2):245–254.
Talukdar, D., Seenivasan, S., Cameron, A., et al. (2020).
The association between national income and adult
obesity prevalence: Empirical insights into tempo-
ral patterns and moderators of the association using
40 years of data across 147 countries. PloS One,
15(5):e0232236.
Tan, R. and Pu, S. (2002). Impact of obesity on hypogo-
nadism in the andropause. International Journal of
Andrology, 25(4):195–201.
Thomas, D., Weedermann, M., Fuemmeler, B., et al.
(2014). Dynamic model predicting overweight, obe-
sity, and extreme obesity prevalence trends. Obesity,
22(2):590–597.
Van de Kassteele, J., Hoogenveen, R., Engelfriet, P., et al.
(2012). Estimating net transition probabilities from
cross-sectional data with application to risk factors
in chronic disease modeling. Statistics in Medicine,
31(6):533–543.
Van Hooff, M., Voorhorst, F., Kaptein, M., et al. (2004).
Predictive value of menstrual cycle pattern, body mass
index, hormone levels and polycystic ovaries at age 15
years for oligo-amenorrhoea at age 18 years. Human
Reproduction, 19(2):383–392.
Wang, L., Denniston, M., Lee, S., et al. (2010). Long-term
health and economic impact of preventing and reduc-
ing overweight and obesity in adolescence. Journal of
Adolescent Health, 46(5):467–473.
Ward, Z. J., Long, M. W., Resch, S. C., et al. (2017). Simu-
lation of growth trajectories of childhood obesity into
adulthood. The New England Journal of Medicine,
377:2145–2153.
World Health Organization (2003). Controlling the global
obesity epidemic. https://www.who.int/nutrition/
topics/obesity/en/. Online; accessed 9 sep 2020.
World Health Organization (2016). Obesity and over-
weight. https://www.who.int/news-room/fact-sheets/
detail/obesity-and-overweight. Online; accessed 9 sep
2020.
Xue, H., Slivka, L., Igusa, T., et al. (2018). Applications
of systems modelling in obesity research. Obesity Re-
views, 19(9):1293–1308.
Mathematical Model for Estimating Nutritional Status of the Population with Poor Data Quality in Developing Countries: The Case of Chile
415