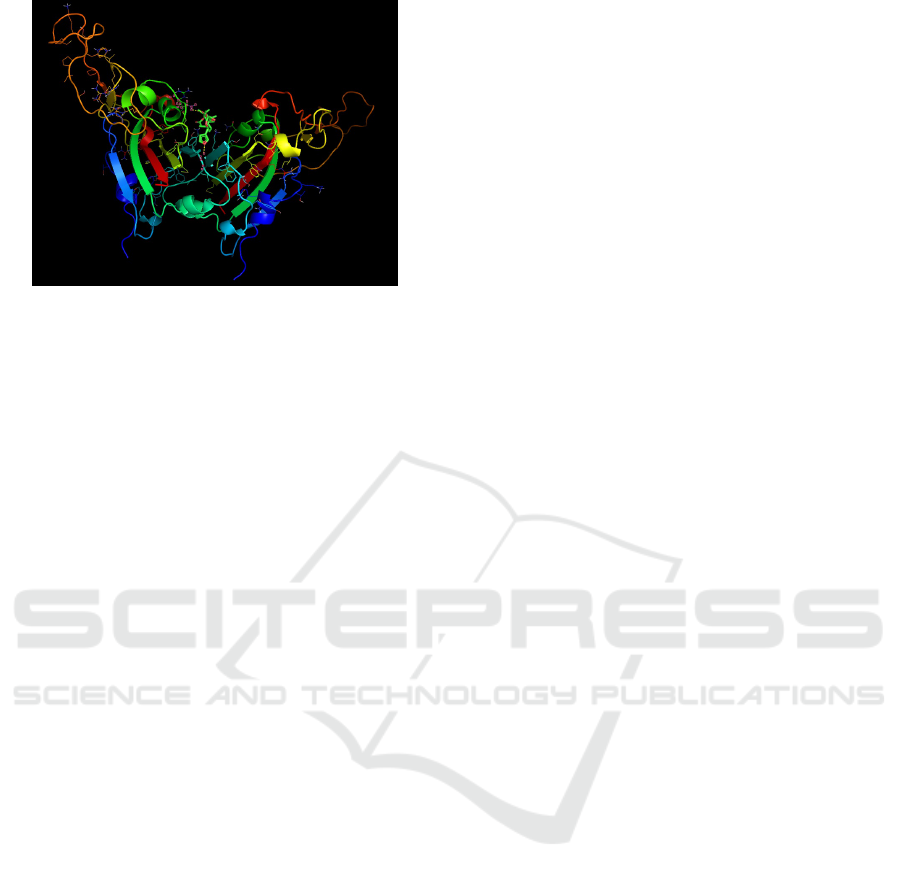
Figure 10: Complex of Spike protein with Obacunone with
selected ligand sites in PyMOL.
tested ligands showed higher dock score than remde-
sivir and dexamethasone, with the maximum dock
score shown by auranetin. Therefore, with the com-
bined docking results and the medical importance
of auranetin, we propose that auranetin and other
flavonoids can be further studied with the aim to get
suitable drugs against COVID-19.
REFERENCES
Aaftaab, S., Khusbhoo, J., Sasikala, K., and Mallika, A.
(2019). Molecular docking in modern drug discovery:
Principles and recent applications.
Cooperman, B. S. (2013). Allosteric regulation. Encyclo-
pedia Of Biological Chemistry, 71-74.
FDA (2020). Food and Drug Administration Agency.
Gheblawi, M., Wang, K., Viveiros, A., Nguyen, Q., Zhong,
J.-C., Turner, A. J., et al. (2020). Angiotensin-
converting enzyme 2: Sars-cov-2 receptor and regu-
lator of the renin-angiotensin system. Circ Res. 2020
May 8; 126(10).
Gruber, J. V., Holtz, R., Sikkink, S. K., and Tobin, D. J.
(2014). In vitro and ex vivo examination of topical
pomiferin treatments.
Huang, Y., Yang, C., Xu, X.-f., Xu, W., and Liu, S.-
w. (2020). Structural and functional properties of
sars-cov-2 spike protein: potential antivirus drug de-
velopment for covid-19. Acta Pharmacol Sin 41,
1141–1149 (2020).
Hung, C. and Chen, C. (2014). Computational approaches
for drug discovery. Drug development research, 75(6),
412–418.
Hwang, S.-L., Shih, P.-H., and Yen, G.-C. (2015). Chap-
ter 80 - citrus flavonoids and effects in dementia and
age-related cognitive decline. Diet and Nutrition in
Dementia and Cognitive Decline.
Jean, S.-S., Lee, P.-I., and Hsueh, P.-R. (2020). Treat-
ment options for covid-19: The reality and challenges.
Journal of Microbiology, Immunology and Infection
Volume 53, Issue 3, June 2020, Pages 436-443.
Jing, X., Ai-hua, Z., Shi, Q., Tian-lei, Z., Xian-na, L.,
Guang-li, Y., et al. (2019). Identification of the per-
turbed metabolic pathways associating with prostate
cancer cells and anticancer affects of obacunone.
Kouznetsova, V., Huang, D., and Tsigelny, I. F. (2020).
Potential covid-19 protease inhibitors: Repurposing
fdaapproved drugs.
MGLTools (2020). MGLTools - software developed at
the Molecular Graphics Laboratory (MGL) of The
Scripps Research Institute.
Narkhede, R. R., Cheke, R. S., Ambhore, J. P., and Shinde,
S. D. (2020). The molecular docking study of poten-
tial drug candidates showing anti-covid-19 activity by
exploring of therapeutic targets of sars-cov-2. screen-
ing, 5(8).
Nataraj, S., P., Khajamohiddin, S., and Jack, T. (2017).
Software for molecular docking: a review.
Oleg, T. and Arthur, J. O. (2010). Autodock vina: im-
proving the speed and accuracy of docking with a
new scoring function, efficient optimization and mul-
tithreading. Journal of computational chemistry vol.
31,2 (2010): 455-61.
Omar, S., Bouziane, I., Bouslama, Z., and Djemel, A.
(2020). In-silico identification of potent inhibitors
of covid-19 main protease (mpro) and angiotensin
converting enzyme 2 (ace2) from natural products:
Quercetin, hispidulin, and cirsimaritin exhibited better
potential inhibition than hydroxy-chloroquine against
covid-19 main protease active site and ace2.
Peter, H., Wei, S. L., Jonathan, E., Marion, M., Jennifer,
B., Louise, L., et al. (2020). Effect of dexamethasone
in hospitalized patients with covid-19: Preliminary re-
port. medRxiv.
Pham, M. Q., Vu, K. B., Pham, T. N. H., Tran, L. H., Tung,
N. T., Vu, V. V., Nguyen, T. H., Ngo, S. T., et al.
(2020). Rapid prediction of possible inhibitors for
sars-cov-2 main protease using docking and fpl sim-
ulations. RSC Advances, 10(53):31991–31996.
PubChem (2020). PubChem - open chemistry database at
the National Institutes of Health (NIH).
PyMOL (2020). PyMOL is a user-sponsored molecular
visualization system on an open-source foundation,
maintained and distributed by Schr
¨
odinger.
RCSB (2020). RCSB Protein Data Bank.
Singh, P., Sharma, A., and Nandi, S. P. (2020). Iden-
tification of potent inhibitors of covid-19 main pro-
tease enzyme by molecular docking study. ChemRxiv.
Preprint.
Sparks, D. (2020). Covid-19 (coronavirus) drugs: Are there
any that work? Mayo Clinic.
Ullrich, S. and Nitsche, C. (2020). The sars-cov-2 main pro-
tease as drug target. Bioorganic & Medicinal Chem-
istry Letters, Volume 30, Issue 17, 1 September 2020,
127377.
Vardhan, S. and Sahoo, S. K. (2020). Searching in-
hibitors for three important proteins of covid-19
through molecular docking studies. arXiv preprint
arXiv:2004.08095.
Wu, C., Liu, Y., Yang, Y., Zhang, P., Zhong, W., et al.
(2020). Analysis of therapeutic targets for sars-cov-
BIOINFORMATICS 2021 - 12th International Conference on Bioinformatics Models, Methods and Algorithms
116