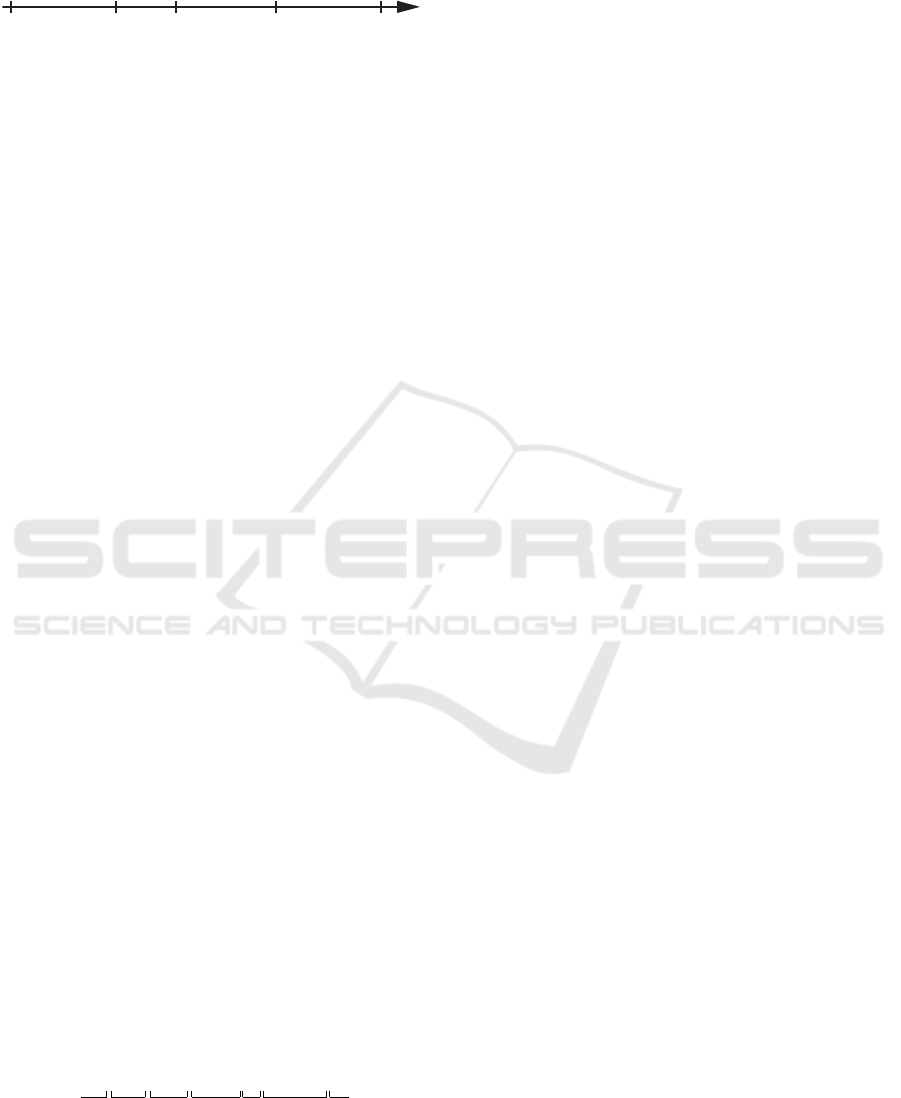
time (in s)
buyers
service
free time
of
cash register
free time
of
cash register
buyers
service
Figure 4: Interplay of buyer free time intervals and buyer
service times at cash register.
parameters such as the buyers’ concentration and the
buyers’ probability allow a good analysis of the flow
of shop customers. The validation of the obtained re-
sults will be carried out using the data of two super-
markets of different sizes in Lithuania.
The novelty of this paper is given by the analy-
sis of internal factors in bottleneck detection through
a comparison of different approaches for measuring
burstiness in real shop processes. Internal factors are
investigated through the waiting time in the queue to
the cash register as well as the payment processing
time at the cash register. It is worth noting that this pa-
per is dedicated to the payment process by jointly de-
scribing and modelling the waiting in the queue to the
cash register as well as the payment processing time
at the cash register by gap processes. Describing each
process by gaps individually, the payment process can
be modelled by two independent gap-processes with
different parameters. Exemplary, gap parameters for
modelling and simulation are found by analyzing the
payment process of two supermarkets in Lithuania.
The remaining part of this paper is organized as
follows: Section 2 introduces the theoretical basis for
internal factors in bottleneck detection. The distribu-
tion of buyers within bursty environments for bottle-
neck analysis is given in Section 3 followed by ap-
proaches for measuring burstiness in bursty business
processes in Section 4. The associated results of an
empirical study of different grocery shops in Lithua-
nia are discussed in Section 5. Finally, some conclud-
ing remarks are provided in Section 6.
2 BURSTY BUSINESS
PROCESSES
In general, any process including the process of buy-
ing in which binary decisions are made can be de-
scribed by gaps as illustrated in Fig. 5 (Ahrens et al.,
2019b).
- x - - x - - x - - - x x - - - - x -
2 2 3 40
Figure 5: Modelling of the buying process by gaps (a buyer
(represented by ”x”) within a sequence of non-buying visi-
tors (represented by ”-”)).
When neglecting the payment processing times
(also referred as buyers’ service times), gap processes
appear when describing the free time intervals be-
tween buyers at the cash register as highlighted in
Fig. 5. The process of buying can be defined by buyer
probability p
e
as well as buyer concentration (1 − α)
(Wilhelm, 1976; Ahrens, 2000). A customer becomes
with the probability p
e
a buyer and remains with the
probability (1 − p
e
) a visitor. On the other hand, the
payment processing time at the cash register can be
modelled by gaps as well. Therefore the payment
process can be modelled by two independent gap pro-
cesses, namely the description of the waiting time in
the queue to the cash register and the payment pro-
cessing time at the cash register.
The distribution of the gaps (e. g. describing free
time intervals between two buyers) can be described
by a gap distribution function u(k) defining the prob-
ability that a gap Y between two buyers is greater than
or at least equal to a given number k, i. e.
u(k) = P(Y ≥ k) . (1)
A bursty behaviour could emerge, if u(k) is dif-
ferent from the exponential distribution function
(Kessler et al., 2003; Weisstein, 1999). Next to gap-
distributions described by one parameter such as the
beforehand mentioned exponential, distribution func-
tions such as Weibull (Weisstein, 1999; Kessler et al.,
2003) or Wilhelm (Wilhelm, 1976), which depend on
two parameters, allow a greater precision when de-
scribing bursty business processes known from bit-
errors in telecommunications (Ahrens, 2000) as well
as the characteristic of transmission control protocol
(TCP) connection arrivals (Feldmann, 2000; Kessler
et al., 2003) or when analyzing the internet traffic
(Zukerman et al., 2003; Kresch and Kulkarni, 2011).
The distribution function u(k), published by Wil-
helm (Wilhelm, 1976), is defined by the parameters,
namely buyer probability p
e
and buyer concentration
(1 − α ), and results for bursty business processes (in-
cluding shop sales) in
u(k) = [(k + 1)
α
− k
α
] · e
−β·k
. (2)
with the parameter β defined by
p
e
≈ β
α
. (3)
For independent buyers, the buyers’ concentration re-
sults in (1 − α) = 0, whereas for practically relevant
bursty buyers’ processes, a buyer concentration in the
range of 0 < (1 − α) ≤ 0,5 can be expected. The
gap distribution function defined in (2) is of a high
level of practical relevance as the function was found
by analysing bit-errors in short-wave communication
channels (Wilhelm, 1976) and confirmed by analyz-
ing packet arrivals in TCP connections (Kessler et al.,
2003).
Internal and External Factor Analysis in Bottleneck Detection in Shop Sales: The Case of Grocery Shops in Lithuania
55