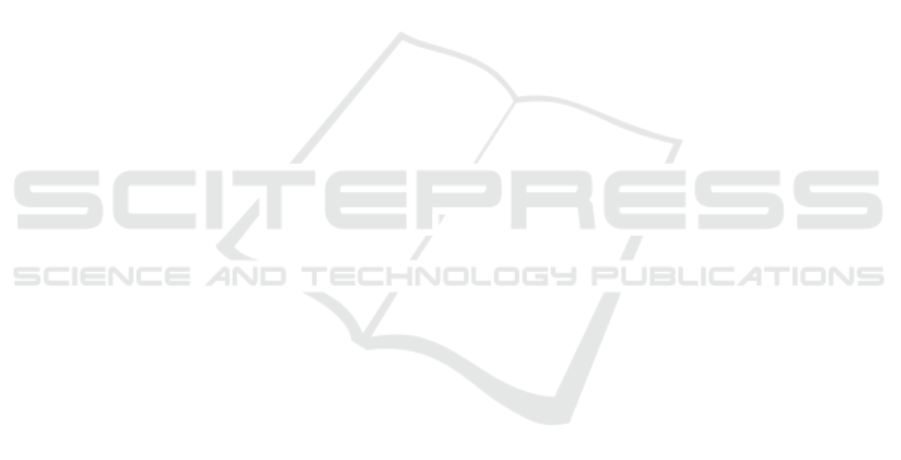
6 CONCLUSION
In this paper, we have considered additional fac-
tors like content delight, frequency of watching on-
line video content and user mood and their impact
on MOS for multimedia communication. The video
stimuli were streamed in different packet loss scenar-
ios, and we have used both binary and ordinal scale
to take account of the user delight. We have seen a
slight impact of both frequency of using online video
content or mood on MOS, but the results are not sta-
tistically significant. On the other hand, we have ob-
served a slight tendency to give higher MOS ratings to
stimuli where the user is delighted to watch content,
but the different is not too large. It is important to
mention that all subjects were technologically aware
of the field, and we might get more relevance from
the diverse set of users in an additional study. The
results establish the effectiveness of MOS ratings ob-
tained through subjective assessments for video clips.
Finally, we have benchmarked the subjective MOS
ratings with PEVQ MOS and observed the software
tendency to overestimate the quality of the streamed
videos. This paper motivates to test effectiveness of
the results by using latest codecs with high resolution
videos streamed over high-speed networks in future
work.
REFERENCES
Cisco (2018). Cisco Annual Internet Report - Cisco Annual
Internet Report (2018–2023) White Paper. Technical
report. Library Catalog: www.cisco.com.
Engelke, U., Maeder, A., and Zepernick, H. J. (2012). Hu-
man observer confidence in image quality assessment.
Signal Processing: Image Communication, 27:935–
947.
IBM Corp. (2019). IBM SPSS Statistics for Windows ver-
sion 22.
ITU-R Rec. BT.500-10 (2012). Methodology for the sub-
jective assessment of the quality of television pictures.
ITU-T Rec. J.247 (2008). Objective perceptual multimedia
video quality measurement in the presence of a full
reference.
ITU-T Rec. P.10/G.100 (2017). Vocabulary for perfor-
mance, quality of service and quality of experience.
Recommendation (11/2017), ITU-T.
ITU-T Rec. P.910 (2008). Subjective video quality assess-
ment methods for multimedia applications.
Johnston, K. (2018). Netflix and Video Streaming Widen
Lead over Subscription TV in Customer Satisfaction
American Customer Satisfaction Index.
Minhas, T. N. and Fiedler, M. (2013). Quality of experi-
ence hourglass model. In Computing, Management
and Telecommunications (ComManTel), 2013 Inter-
national Conference on, page 87–92. IEEE.
Minhas, T. N., Nawaz, O., Fiedler, M., and Khatibi, S.
(2019). The Effects of Additional Factors on Subjec-
tive Quality Assessments. In 2019 2nd International
Conference on Advancements in Computational Sci-
ences (ICACS), pages 1–5.
Nawaz, O., Minhas, T., and Fiedler, M. (2014). Optimal
MTU for realtime video broadcast with packet loss;
A QoE perspective. In The 9th International Con-
ference for Internet Technology and Secured Transac-
tions (ICITST-2014), London, pages 396–401.
Nawaz, O., Minhas, T. N., and Fiedler, M. (2017). QoE
based comparison of H.264/AVC and WebM/VP8 in
an error-prone wireless network. In Integrated Net-
work and Service Management (IM), 2017 IFIP/IEEE
Symposium, Lisbon, pages 1005–1010.
Ofcom (2019). Comparing service quality: Choosing the
best broadband, mobile and landline provider.
Opticom-GmbH (2016). PEVQ, advanced perceptual eval-
uation of video quality, White Paper. Technical report.
Library Catalog: www.opticom.de.
Pinson, M. H., Janowski, L., Pepion, R., Huynh-Thu, Q.,
Schmidmer, C., Corriveau, P., Younkin, A., Callet,
P. L., Barkowsky, M., and Ingram, W. (2012). The In-
fluence of Subjects and Environment on Audiovisual
Subjective Tests: An International Study. IEEE Jour-
nal of Selected Topics in Signal Processing, 6(6):640–
651.
Qualinet (2013). Qualinet White Paper on Definitions of
Quality of Experience. Technical report. Library Cat-
alog: www.qualinet.eu.
Reiter, U., Brunnstr
¨
om, K., De Moor, K., Larabi, M.-C.,
Pereira, M., Pinheiro, A., You, J., and Zgank, A.
(2014). Factors Influencing Quality of Experience. In
M
¨
oller, S. and Raake, A., editors, Quality of Experi-
ence: Advanced Concepts, Applications and Methods,
T-Labs Series in Telecommunication Services, pages
55–72. Springer International Publishing, Cham.
Robitza, W. and Hlavacs, H. (2014). Assessing the validity
of subjective qoe data through rating times and self-
reported confidence. In Quality of Multimedia Expe-
rience (QoMEX), 2014 Sixth International Workshop,
pages 297–302.
Russell, J. A. (1980). A Circumplex Model of Af-
fect. Journal of Personality and Social Psychology,
39(6):1161–1178.
Schleicher, R. and Antons, J.-N. (2014). Evoking Emo-
tions and Evaluating Emotional Impact. In M
¨
oller,
S. and Raake, A., editors, Quality of Experience: Ad-
vanced Concepts, Applications and Methods, T-Labs
Series in Telecommunication Services, pages 121–
132. Springer International Publishing, Cham.
Streijl, R. C., Winkler, S., and Hands, D. S. (2016). Mean
Opinion Score (MOS) Revisited: Methods and Ap-
plications, Limitations and Alternatives. Multimedia
Syst., 22(2):213–227.
Zhu, Y., Heynderickx, I., and Redi, J. A. (2015). Under-
standing the role of social context and user factors in
video Quality of Experience. Computers in Human
Behavior, 49:412–426.
DCNET 2020 - 11th International Conference on Data Communication Networking
66