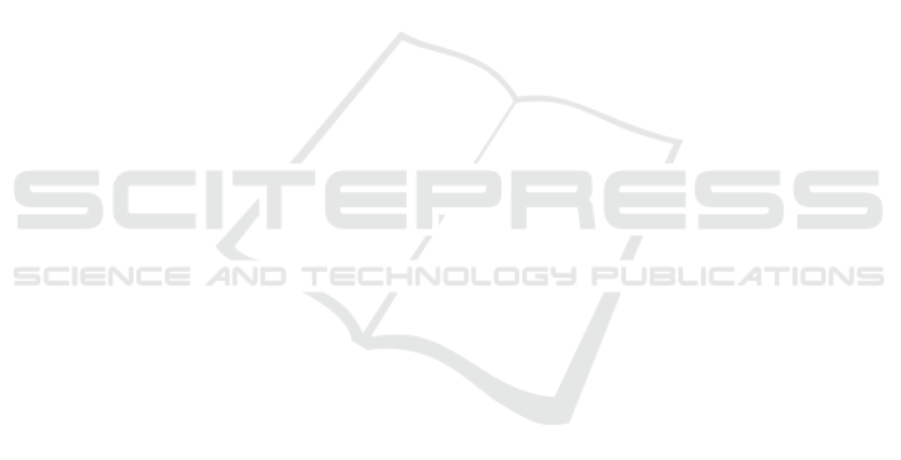
REFERENCES
Activ8 (2016). Activ8 Professional validation - Ac-
tiv8all.com.
A&DCompany (2016). Clinical Validation | Medical |
Products | A&D.
Baumeister, H., Nowoczin, L., Lin, J., Seifferth, H., Seufert,
J., Laubner, K., and Ebert, D. D. (2014). Impact of
an acceptance facilitating intervention on diabetes pa-
tients’ acceptance of internet-based interventions for
depression: a randomized controlled trial. Diabetes
research and clinical practice, 105(1):30–39.
Beddit (2016). Beddit Sleep Tracker.
Biernacki, P. and Waldorf, D. (1981). Snowball sampling:
Problems and techniques of chain referral sampling.
Sociological methods & research, 10(2):141–163.
Buckingham, C. D. (2016). GRiST - Galatean Risk and
Safety Tool.
Champion, V. L., Skinner, C. S., et al. (2008). The health
belief model. Health behavior and health education:
Theory, research, and practice, 4:45–65.
Cimperman, M., Bren
ˇ
ci
ˇ
c, M. M., and Trkman, P. (2016).
Analyzing older users’ home telehealth services
acceptance behavior—applying an extended utaut
model. International journal of medical informatics,
90:22–31.
Davis, F. D. (1985). A Technology Acceptance Model
for Empirically Testing New End-user Information
Systems: Theory and Results. Massachusetts Insti-
tute of Technology, Sloan School of Management,
https://dspace.mit.edu/handle/1721.1/15192. Google-
Books-ID: hbx8NwAACAAJ.
De Witte, N. and Van Daele, T. (2017). Vlaamse UTAUT-
vragenlijst.
Deci, E. L. and Ryan, R. M. (2012). Self-determination
theory. Handbook of theories of social psychology.
D’Haeseleer, I., Gerling, K., Schreurs, D., Buckingham,
C., Abeele, V., et al. (2019a). Uses and attitudes of
old and oldest adults towards self-monitoring health
systems. In Pervasive Health, Date: 2019/05/20-
2019/05/23, Location: Trento, Italy.
D’Haeseleer, I., Gerling, K., Schreurs, D., Vanrumste, B.,
and Vanden Abeele, V. (2019b). Ageing is not a dis-
ease: Pitfalls for the acceptance of self-management
health systems supporting healthy ageing. In The 21st
International ACM SIGACCESS Conference on Com-
puters and Accessibility, pages 286–298. ACM.
Doyle, J., Walsh, L., Sassu, A., and McDonagh, T.
(2014). Designing a Wellness Self-management Tool
for Older Adults: Results from a Field Trial of
YourWellness. In Proceedings of the 8th Interna-
tional Conference on Pervasive Computing Technolo-
gies for Healthcare, PervasiveHealth ’14, pages 134–
141, ICST, Brussels, Belgium, Belgium. ICST (In-
stitute for Computer Sciences, Social-Informatics and
Telecommunications Engineering).
Ebert, D. D., Berking, M., Cuijpers, P., Lehr, D., P
¨
ortner,
M., and Baumeister, H. (2015). Increasing the ac-
ceptance of internet-based mental health interventions
in primary care patients with depressive symptoms. a
randomized controlled trial. Journal of affective dis-
orders, 176:9–17.
Fishbein, M. (1979). A theory of reasoned action: some ap-
plications and implications. In Nebraska Symposium
on Motivation. University of Nebraska Press.
Fougeyrollas, P. and Noreau, L. (2001). Life Habits.
Heart, T. and Kalderon, E. (2013). Older adults: Are they
ready to adopt health-related ICT? International Jour-
nal of Medical Informatics, 82(11):e209–e231.
IBM (2017). Ibm spss statistics.
iHealth Labs Inc. (2019). ihealth. (Accessed on
01/03/2020).
Innovations, B. (2016). BeWell Innovations.
Jaschinski, C. (2014). Ambient Assisted Living: Towards
a Model of Technology Adoption and Use Among El-
derly Users. In Proceedings of the 2014 ACM Interna-
tional Joint Conference on Pervasive and Ubiquitous
Computing: Adjunct Publication, UbiComp ’14 Ad-
junct, pages 319–324, New York, NY, USA. ACM.
Jaschinski, C. and Allouch, S. B. (2015). Understanding the
user’s acceptance of a sensor-based ambient assisted
living application. In Human Behavior Understand-
ing, pages 13–25. Springer.
Jim
´
enez-Mixco, V., Cabrera-Umpi
´
errez, M. F., Arren-
dondo, M. T., Panou, M., Struck, M., and Bonfiglio,
S. (2013). Feasibility of a wireless health monitoring
system for prevention and health assessment of elderly
people. In 2013 35th Annual International Conference
of the IEEE Engineering in Medicine and Biology So-
ciety (EMBC), pages 7306–7309, Osaka, Japan. IEEE.
Khechine, H., Lakhal, S., and Ndjambou, P. (2016).
A meta-analysis of the utaut model: Eleven
years later. Canadian Journal of Administra-
tive Sciences/Revue Canadienne des Sciences de
l’Administration, 33(2):138–152.
Kononova, A., Li, L., Kamp, K., Bowen, M., Rikard, R.,
Cotten, S., and Peng, W. (2019). The use of wear-
able activity trackers among older adults: Focus group
study of tracker perceptions, motivators, and barriers
in the maintenance stage of behavior change. JMIR
mHealth and uHealth, 7(4):e9832.
Lemmens, J., Ism van Engelen, E., Post, M. W., de Witte,
L. P., Beurskens, A., Wolters, P. M., and de Witte, L. P.
(2007). Reproducibility and validity of the dutch life
habits questionnaire (life-h 3.0) in older adults. Clini-
cal rehabilitation, 21(9):853–862.
Lumos Labs, I. (2020). Lumosity brain training. (Accessed
on 01/03/2020).
McMahon, S. K., Lewis, B., Oakes, M., Guan, W., Wyman,
J. F., and Rothman, A. J. (2016). Older adults’ ex-
periences using a commercially available monitor to
self-track their physical activity. JMIR mHealth and
uHealth, 4(2):e35.
Nations, U. (2015). World Population Prospects - Popula-
tion Division - United Nations.
Nunes, F., Verdezoto, N., Fitzpatrick, G., Kyng, M.,
Gr
¨
onvall, E., and Storni, C. (2015). Self-care tech-
nologies in hci: Trends, tensions, and opportunities.
ACM Transactions on Computer-Human Interaction
(TOCHI), 22(6):1–45.
The Unacceptance of a Self-Management Health System by Healthy Older Adults
279