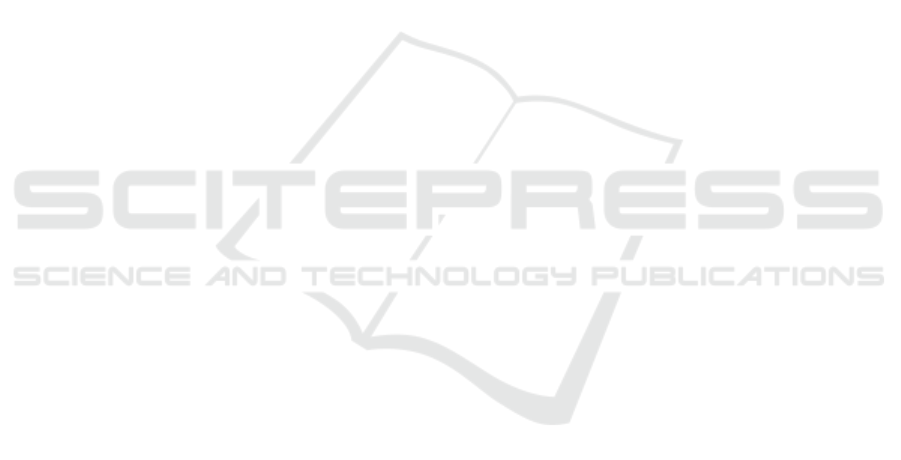
ieeexplore.ieee.org/stamp/stamp.jsp?tp=&arnumber=6
901413&isnumber=6901368
Cook, R., Kay, J., & Kummerfeld, B. (2015). MOOClm:
User Modelling for MOOCs. 9146, 80–91.
https://doi.org/10.1007/978-3-319-20267-9
Cropley, A.J. 1978. Some Guidelines for the Reform of
School Curricula in the Perspective of Lifelong
Education. International Review of Education, 24
(1):21–33.
Dimitrova, V., & Brna, P. (2016). From Interactive Open
Learner Modelling to Intelligent Mentoring: STyLE-
OLM and beyond. International Journal of Artificial
Intelligence in Education, 26(1), 332–349.
https://doi.org/10.1007/s40593-015-0087-3
El Mawas, N., Gilliot, J. M., Garlatti, S., Euler, R., &
Pascual, S. (2018). Towards personalized content in
massive open online courses. CSEDU 2018 -
Proceedings of the 10th International Conference on
Computer Supported Education, 2, 331–339.
https://doi.org/10.5220/0006816703310339
El Mawas N., Gilliot JM., Garlatti S., Euler R., Pascual S.
2019. As One Size Doesn’t Fit All, Personalized
Massive Open Online Courses Are Required. In:
McLaren B., Reilly R., Zvacek S., Uhomoibhi J. (eds)
Computer Supported Education. CSEDU 2018.
Communications in Computer and Information
Science, vol 1022. Springer, Cham
Giannandrea, L., & Sansoni, M. 2013. A literature review
on intelligent tutoring systems and on student profiling.
Learning & Teaching with Media & Technology, 287.
Harrathi, M., Touzani, N., & Braham, R. (2018). A hybrid
knowlegde-based approach for recommending massive
learning activities. Proceedings of IEEE/ACS
International Conference on Computer Systems and
Applications, AICCSA, 2017-Octob, 49–54. https://
doi.org/10.1109/AICCSA.2017.150
He, X., Liu, P., & Zhang, W. (2017). Design and
Implementation of a Unified Mooc Recommendation
System for Social Work Major: Experiences and
Lessons. Proceedings - 2017 IEEE International
Conference on Computational Science and Engineering
and IEEE/IFIP International Conference on Embedded
and Ubiquitous Computing, CSE and EUC 2017, 1,
219–223. https://doi.org/10.1109/CSE-EUC.2017.46
Herder, E. 2016. User Modeling and Personalization 3:
User Modeling – Techniques. https://
www.eelcoherder.com/images/teaching/usermodeling/
03_user_modeling_techniques.pdf
Iatrellis, O., Kameas, A., & Fitsilis, P. (2019). EDUC8
ontology: semantic modeling of multi-facet learning
pathways. Education and Information Technologies,
24(4), 2371–2390. https://doi.org/10.1007/s10639-019-
09877-4
Ishola, O. M., & McCalla, G. 2016. Tracking and Reacting
to the Evolving Knowledge Needs of Lifelong
Professional Learners. In UMAP (Extended
Proceedings).
Jordan, K. 2014. Initial Trends in Enrolment and
Completion of Massive Open Online Courses. The
International Review of Research in Open and
Distributed Learning 15 (1)
Jung, Y., & Lee, J. (2018). Learning engagement and
persistence in massive open online courses (MOOCs).
Computers & Education, 122, 9–22. https://
doi.org/10.1016/j.compedu.2018.02.013
Karahoca, A., Yengin, I., & Karahoca, D. (2018). Cognitive
dialog games as cognitive assistants: Tracking and
adapting knowledge and interactions in student’s
dialogs. International Journal of Cognitive Research in
Science, Engineering and Education, 6(1), 45–52.
https://doi.org/10.5937/ijcrsee1801045K
Kay, J. 2012. AI and education: Grand challenges. IEEE
Intelligent Systems, 27(5), 66–69.
Kay, J. & Kummerfeld, B. 2011. Lifelong Learner
Modeling. In P. J. Durlach and A. Lesgold (Eds.),
Adaptive Technologies for Training and Education.
Cambridge University Press.
Kay, J., & Kummerfeld, B. 2019. From data to personal
user models for life‐long, life‐wide learners. British
Journal of Educational Technology, 50(6), 2871-2884.
Knapper, C., & Cropley, A. J. 2000. Lifelong learning in
higher education. Psychology Press.
Li, Y., Zheng, Y., Kang, J., & Bao, H. (2016). Designing a
Learning Recommender System by Incorporating
Resource Association Analysis and Social Interaction
Computing. https://doi.org/10.1007/978-981-287-868-
7_16
Liang-Zhong, C., Fu-Liang, G., and Ying-ji, L. 2018.
Research Overview of Educational Recommender
Systems. In Proceedings of the 2nd International
Conference on Computer Science and Application
Engineering (CSAE ’18). Association for Computing
Machinery, New York, NY, USA, Article 155, 1–7.
DOI: https://doi.org/10.1145/3207677.3278071
Lynda, H., & Bouarab-Dahmani, F. (2019). Gradual
learners’ assessment in massive open online courses
based on ODALA approach. Journal of Information
Technology Research, 12(3), 21–43. https://doi.org/
10.4018/JITR.2019070102
Maalej, W., Pernelle, P., Ben Amar, C., Carron, T., &
Kredens, E. (2016). Modeling Skills in a Learner-
Centred Approach Within MOOCs (D. K. W. Chiu, I.
Marenzi, U. Nanni, M. Spaniol, & M. Temperini, eds.).
In (pp. 102–111). https://doi.org/10.1007/978-3-319-
47440-3_11
Maravanyika, M., Dlodlo, N., & Jere, N. (2017). An
adaptive recommender-system based framework for
personalised teaching and learning on e-learning
platforms. 2017 IST-Africa Week Conference, IST-
Africa 2017, 1–9. Martins, C., Faria, L., De Carvalho,
C. V., & Carrapatoso, E. 2008. User modeling in
adaptive hypermedia educational systems. In
Educational Technology & Society, 11(1), 194–207.
https://doi.org/10.23919/ISTAFRICA.2017.8102297
Morales, R., Van Labeke, N., Brna, P., & Chan, M. E. 2009.
Open Learner Modelling as the Keystone of the Next
Generation of Adaptive Learning Environments. In C.
Mourlas, & P. Germanakos (Eds.), Intelligent User
Interfaces: Adaptation and Personalization Systems and
CSEDU 2020 - 12th International Conference on Computer Supported Education
538