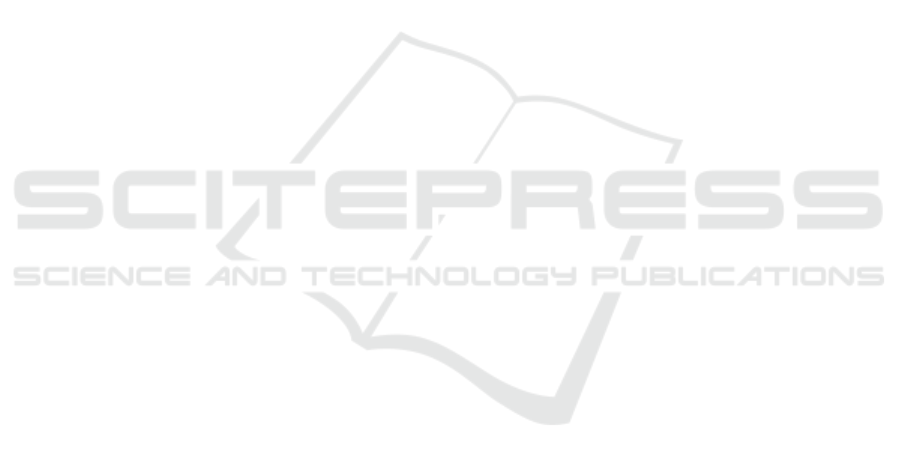
als’ mobility should go along with more clinical
testings to reach better predictions. In opposite
of current strategies applied in Kedougou, which
do not include human mobility details and figure
out the overall disease trends weeks or months
later, our model instantly plots detailed informa-
tion about malaria dispersal. Hence, policy mak-
ers may foresee the right actions to do in each
patch even though the disease has not happened
yet.
• What-if Analysis Boost. Last but not the least,
our solution can be used to calibrate the overall
actions against malaria. In fact, we can model the
disease spread while asking or supposing a spe-
cific pattern. For instance, we can suppose (or
eventually suggest) that people have to stay home
during their incubation period in order to reduce
the global evolution of the disease. Likewise,
we can suggest them following a specific mobil-
ity pattern based on the FoI of different patches.
In other words, we offer policy maker a tool that
can be used as dashboard to evaluate different sce-
nario and their effects.
Even if the experimental results we got are in-
teresting and rather intuitive sometime, they could
be more accurate with real mobility data. In ongo-
ing work, we plan to acquire those data and conduct
deeper experimental validation.
6 CONCLUSION
In this article we proposed a malaria model that takes
into account individual information such as mobility
patterns, health statuses, and so on. The model is a
discrete SEIR-SIS approach and differs mainly from
existing models by the fact that global details about
the disease are obtained by gathering the health sta-
tus of each individual among a given population. Our
approach is more accurate in terms of estimating the
disease level, and affords the possibility to setup more
efficient vector control strategies aiming to eliminate
malaria in Africa. Actually, our model is an early ex-
planation of Kedougou case where the malaria inci-
dence is still high despite the strategies of the Senegal
national program. In this respect, it unveils kind of
solutions that decision makers can use with respect to
individuals movement in order to eliminate the dis-
ease. Moreover, the results from our model match
real observations in Kedougou with a mean absolute
error of 0.001 while using synthetic data. Such a re-
sult is promising and let us believe that with real data,
our approach will behave efficiently. Thus, ongoing
works are conducted to collect on-line mobility data
obtained, for instance, through mobile networks. We
are aware that the results presented in this paper are
mainly based on synthetic data and thus cannot be in-
terpreted as realistic results. However, as we obtain
expected values we believe that our model behaves
correctly and should give useful information when ap-
plied to real data in the future.
REFERENCES
ANSD (2013). Projection de la populatiion de
la région de kedougou - 2013-2025. http:
//www.ansd.sn/ressources/publications/indicateurs/
Projections-demographiques-2013-2025+.htm.
Accessed: 2019-05-02.
Arthur, S. (2017). Malaria incubation period. http://malaria.
emedtv.com/malaria/malaria-incubation-period.html.
Accessed: 2017-03-29.
Chitnis, N., Cushing, J., and Hyman, J. (2006). Bifur-
cation analysis of a mathematical model for malaria
transmission. SIAM Journal on Applied Mathematics,
67(1):24–45.
Chitnis, N., Hyman, J. M., and Cushing, J. M. (2008).
Determining Important Parameters in the Spread of
Malaria Through the Sensitivity Analysis of a Math-
ematical Model. Bulletin of Mathematical Biology,
70(5):1272–1296.
Dharmawardena, P., Rodrigo, C., Mendis, K., de A. W. Gu-
nasekera, W. M. K. T., Premaratne, R., Ringwald,
P., and Fernando, D. (2017). Response of imported
malaria patients to antimalarial medicines in sri lanka
following malaria elimination. PLOS ONE, 12(11):1–
14.
Dimitrov, N. B. and Meyers, L. A. (2010). Mathematical
Approaches to Infectious Disease Prediction and Con-
trol, chapter Chapter 1, pages 1–25. INFORMS.
du Sénégal, G. (2017). National malaria control program.
http://www.pnlp.sn/. Accessed: 2019-01-25.
Filipe, J. A., Riley, E. M., Drakeley, C. J., Sutherland, C. J.,
and Ghani, A. C. (2007). Determination of the pro-
cesses driving the acquisition of immunity to malaria
using a mathematical transmission model. PLoS com-
putational biology, 3(12):1–11.
for Disease Control, C. and Prevention (2015). https://www.
cdc.gov/malaria/about/disease.html. Accessed: 2017-
05-29.
Gharbi, M., Flegg, J. A., Pradines, B., Berenger, A., Ndi-
aye, M., Djimdé, A. A., Roper, C., Hubert, V., Kendjo,
E., Venkatesan, M., Brasseur, P., Gaye, O., Offianan,
A. T., Penali, L., Le Bras, J., Guérin, P. J., and Study,
M. o. t. F. N. R. C. f. I. M. (2013). Surveillance of
travellers: An additional tool for tracking antimalar-
ial drug resistance in endemic countries. PLOS ONE,
8(10):1–11.
Greenwood, B., Marsh, K., and Snow, R. (1991). Why do
some african children develop severe malaria? Para-
sitology today, 7(10):277–281.
A Malaria Control Model using Mobility Data: An Early Explanation of Kedougou Case in Senegal
45