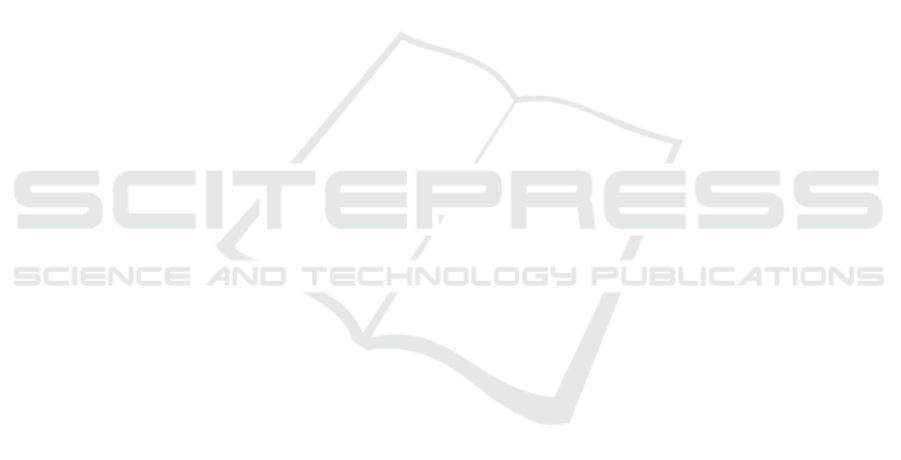
• Incorporate external impacts on ROCI such as cy-
ber insurance;
• Forecasting long-term investment impacts which
plan beyond the first year of cyber solution adop-
tion, including equipment failure rates, annual
maintenance, degradation of performance and
technological upgrades;
• Model attack diversity and intended consequences
that may map to military or government sites (do
attack statistics show that different exploits are
utilized for target-specific campaigns, such as data
exfiltration compared to reduced mission effec-
tiveness?);
Evaluating risk and risk management is another
complex field of study. In the traditional two-axis
management view of risk, risk calculation depends
on two independent variables: consequence (the im-
pact of a successful attack), and probability (the like-
lihood that the attack will be successfully executed).
Currently, the ROCI model conflates these two vari-
ables, an action that may reduce the granularity of the
model’s analysis. In the future, ROCI may be im-
proved by separating these axes, according to the tra-
ditional model of risk estimation. Furthermore, While
cybersecurity risk has been modeled in the traditional
risk management matrix (Collard et al., 2016), other
research shows that these risk matrices are not effec-
tive (Thomas, 2013). Various research methods also
exist to show that decisions can be optimized by in-
corporating risk (Hubbard and Seiersen, 2016). By
capturing the nuances of risk and the methods used
to manage the risk, the Return-on-Investment mod-
els and recommendations would be even more robust,
giving system managers a greater amount of knowl-
edge with where and how to improve their system
from cyber attacks in a cost-efficient and effective
manner.
ACKNOWLEDGEMENTS
Roger A. Hallman is supported by the United States
Department of Defense SMART Scholarship for Ser-
vice Program, funded by USD/R&E (The Under Sec-
retary of Defense-Research and Engineering), Na-
tional Defense Education Program (NDEP) / BA-1,
Basic Research.
REFERENCES
Bergner., S. and Lechner., U. (2017). Cybersecurity ontol-
ogy for critical infrastructures. In Proceedings of the
9th International Joint Conference on Knowledge Dis-
covery, Knowledge Engineering and Knowledge Man-
agement - Volume 2: KEOD,, pages 80–85. INSTICC,
SciTePress.
Bodin, L. D., Gordon, L. A., Loeb, M. P., and Wang,
A. (2018). Cybersecurity insurance and risk-sharing.
Journal of Accounting and Public Policy, 37(6):527–
544.
Carin, L., Cybenko, G., and Hughes, J. (2008). Cybersecu-
rity strategies: The queries methodology. Computer,
41(8):20–26.
Cavusoglu, H., Mishra, B., and Raghunathan, S. (2004). A
model for evaluating it security investments. Commu-
nications of the ACM, 47(7):87–92.
Cieply, M. and Barnes, B. (2015). Sony at-
tack, first a nuisance, swiftly grew into a
firestorm. The New York Times, page A1.
https://www.nytimes.com/2014/12/31/business/media
/sony-attack-first-a-nuisance-swiftly-grew-into-a-
firestorm-.html.
Collard, G., Disson, E., Talens, G., and Ducroquet, S.
(2016). Proposition of a method to aid security clas-
sification in cybersecurity context. In 2016 14th An-
nual Conference on Privacy, Security and Trust (PST),
pages 88–95. IEEE.
Davis, J. H. (2015). Hacking exposed 21 million in u.s.,
government says. The New York Times, page A1.
https://www.nytimes.com/2015/07/10/us/office-
of-personnel-management-hackers-got-data-of-
millions.html.
Gordon, L. A. and Loeb, M. P. (2002). The economics of in-
formation security investment. ACM Transactions on
Information and System Security (TISSEC), 5(4):438–
457.
Gordon, L. A., Loeb, M. P., and Lucyshyn, W. (2014a). Cy-
bersecurity investments in the private sector: the role
of governments. Geo. J. Int’l Aff., 15:79.
Gordon, L. A., Loeb, M. P., Lucyshyn, W., and Zhou, L.
(2015). The impact of information sharing on cyber-
security underinvestment: A real options perspective.
Journal of Accounting and Public Policy, 34(5):509–
519.
Gordon, L. A., Loeb, M. P., Lucyshyn, W., Zhou, L., et al.
(2014b). Externalities and the magnitude of cyber se-
curity underinvestment by private sector firms: a mod-
ification of the gordon-loeb model. Journal of Infor-
mation Security, 6(01):24.
Huang, C. D., Hu, Q., and Behara, R. S. (2008). An eco-
nomic analysis of the optimal information security in-
vestment in the case of a risk-averse firm. Interna-
tional journal of production economics, 114(2):793–
804.
Hubbard, D. W. and Seiersen, R. (2016). How to measure
anything in cybersecurity risk. John Wiley & Sons.
Jajodia, S., Noel, S., Kalapa, P., Albanese, M., and
Williams, J. (2011). Cauldron mission-centric cy-
ber situational awareness with defense in depth. In
2011-MILCOM 2011 Military Communications Con-
ference, pages 1339–1344. IEEE.
Return on Cybersecurity Investment in Operational Technology Systems: Quantifying the Value That Cybersecurity Technologies Provide
after Integration
51