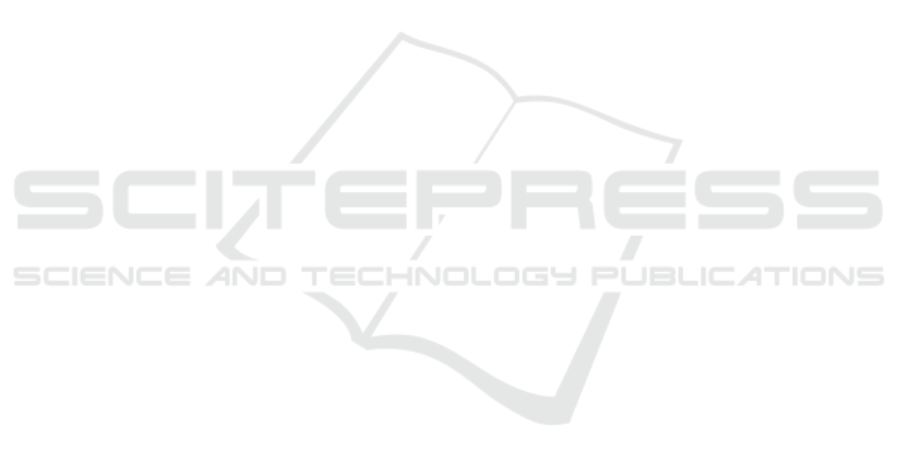
test this, a study was designed that records the indi-
vidual subjects in different sweat status using a ther-
mal imaging camera. For evaluation a forehead area
is used to find out the difference between the maxi-
mum and minimum temperature. Due to the set deci-
sion thresholds, 78% of the images can be classified
correctly. This means that then the status not sweat-
ing was distinguished from sweating. The result also
shows that there is a significant difference between
the sweating and the non-sweating subjects based on
a temperature difference in a forehead area. With this
result, an important step in the direction of climate
automation is done. For this automation, in addi-
tion to already known interior data and personal data,
data about the sweat status of the various occupants
is required. So far, there was no way to detect this
status without contact. Only humidity sensors and
thermocouples were previously used for sweat detec-
tion. With the non-contact sweat detection by thermal
imaging cameras, it can be easily detected in the vehi-
cle, which climate setting is suitable for the individual
occupants.
Since the presented method found only a recog-
nition accuracy of 78%, further alternative methods
are examined to increase the accuracy. Other alterna-
tives of sweat detection without thermal camera are
currently under investigation.
REFERENCES
ASHRAE (2004). ASHRAE handbook fundamentals: At-
lanta, american society of heating. Refrigeration, and
Air Conditioning Engineers, (ANSI/ASHRAE Stan-
dard 55-2004).
Bricout, V.-A., DeChenaud, S., and Favre-Juvin, A. (2010).
Analyses of heart rate variability in young soccer play-
ers: the effects of sport activity. Autonomic Neuro-
science, 154(1-2):112–116.
De Dear, R. (2004). Thermal comfort in practice. Indoor
air, 14(7):32–39.
Dentel, A. and Dietrich, U. (2013). Thermische Be-
haglichkeit – Komfort in Geb
¨
auden. Dokumentation
Primero–Komfort. Hamburg, HafenCity Universit
¨
at,
Institut f
¨
ur Energie und Geb
¨
aude.
Djongyang, N., Tchinda, R., and Njomo, D. (2010). Ther-
mal comfort: A review paper. Renewable and sustain-
able energy reviews, 14(9):2626–2640.
Ebisch, S. J., Aureli, T., Bafunno, D., Cardone, D., Romani,
G. L., and Merla, A. (2012). Mother and child in syn-
chrony: thermal facial imprints of autonomic conta-
gion. Biological psychology, 89(1):123–129.
Epstein, Y. and Moran, D. S. (2006). Thermal comfort and
the heat stress indices. Industrial health, 44(3):388–
398.
Fanger, P. O. (1970). Thermal comfort. Analysis and appli-
cations in environmental engineering. Copenhagen,
Danish Technical Press.
Fern
´
andez-Cuevas, I., Marins, J. C. B., Lastras, J. A., Car-
mona, P. M. G., Cano, S. P., Garc
´
ıa-Concepci
´
on,
M.
´
A., and Sillero-Quintana, M. (2015). Classifica-
tion of factors influencing the use of infrared thermog-
raphy in humans: A review. Infrared Physics & Tech-
nology, 71:28–55.
Fiala, D., Lomas, K. J., and Stohrer, M. (2001). Com-
puter prediction of human thermoregulatory and tem-
perature responses to a wide range of environmental
conditions. International journal of biometeorology,
45(3):143–159.
Karjalainen, S. (2007). Gender differences in thermal com-
fort and use of thermostats in everyday thermal en-
vironments. Building and environment, 42(4):1594–
1603.
Kuno, Y. (1934). Physiology of human perspiration. J. And
A. Churchill: London.
Liu, W., Lian, Z., Deng, Q., and Liu, Y. (2011). Evaluation
of calculation methods of mean skin temperature for
use in thermal comfort study. Building and Environ-
ment, 46(2):478–488.
Liu, W., Lian, Z., and Liu, Y. (2008). Heart rate variability
at different thermal comfort levels. European journal
of applied physiology, 103(3):361–366.
Machado-Moreira, C. A., Wilmink, F., Meijer, A., Mek-
javic, I. B., and Taylor, N. A. (2008). Local differ-
ences in sweat secretion from the head during rest
and exercise in the heat. European journal of applied
physiology, 104(2):257–264.
Merla, A. and Romani, G. L. (2007). Thermal signatures
of emotional arousal: a functional infrared imaging
study. In 2007 29th Annual International Conference
of the IEEE Engineering in Medicine and Biology So-
ciety, pages 247–249. IEEE.
Nakano, J., Tanabe, S.-i., and Kimura, K.-i. (2002). Dif-
ferences in perception of indoor environment between
japanese and non-japanese workers. Energy and
Buildings, 34(6):615–621.
Nielsen, R., Gavhed, D. c., and Nilsson, H. (1989). Ther-
mal function of a clothing ensemble during work: de-
pendency on inner clothing layer fit. Ergonomics,
32(12):1581–1594.
Nkurikiyeyezu, K. N., Suzuki, Y., and Lopez, G. F. (2018).
Heart rate variability as a predictive biomarker of ther-
mal comfort. Journal of Ambient Intelligence and Hu-
manized Computing, 9(5):1465–1477.
Pomeranz, B., Macaulay, R., Caudill, M. A., Kutz, I.,
Adam, D., Gordon, D., Kilborn, K. M., Barger, A. C.,
Shannon, D. C., and Cohen, R. J. (1985). Assessment
of autonomic function in humans by heart rate spec-
tral analysis. American Journal of Physiology-Heart
and Circulatory Physiology, 248(1):H151–H153.
Randall, W. C. (1946). Quantitation and regional distribu-
tion of sweat glands in man. The Journal of clinical
investigation, 25(5):761–767.
Sammito, S. and B
¨
ockelmann, I. (2015). Analyse der
Herzfrequenzvariabilit
¨
at. Herz, 40(1):76–84.
VEHITS 2020 - 6th International Conference on Vehicle Technology and Intelligent Transport Systems
430