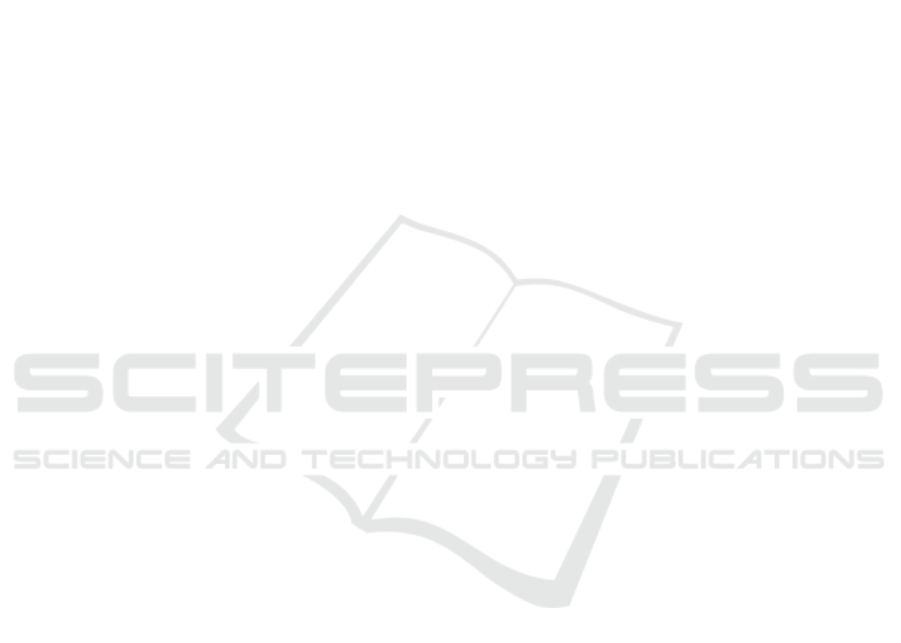
Prediction of the Impact of Physical Exercise on Knee Osteoarthritis
Patients using Kinematic Signal Analysis and Decision Trees
M. Mezghani
1,2
, N. Hagemeister
2
, M. Kouki
1
, Y. Ouakrim
2,4
, A. Fuentes
3
and N. Mezghani
2,4
1
´
Ecole Sup
´
erieure de la Statistique et de l’Analyse de l’Information, Universit
´
e de Carthage, Tunisia
2
Laboratoire de Recherche en Imagerie et Orthop
´
edie (LIO), Centre de Recherche du CHUM, Montreal, Canada
3
EMOVI Inc, Quebec, Canada
4
LICEF Reserach Center, TELUQ University, Montreal, Canada
Keywords:
3D Kinematics, Decision Trees, Knee Osteoarthritis, Physical Exercise, Knee Kinesiography.
Abstract:
The evaluation of knee biomechanics provides valuable clinical information. This can be done by means of a
knee kinesiography exam which measures the three-dimensional rotation angles during walking, thus provid-
ing objective knowledge about knee function (3D kinematics). 3D kinematic data is quantifiable information
that provides opportunities to develop automatic and objective methods for personalized computer-aided treat-
ment systems. The purpose of this study is to explore a decision tree based method for predicting the impact of
physical exercise on a knee osteoarthritis population. The prediction is based on 3D kinematic data i.e., flex-
ion/extension, abduction/adduction and internal/external rotation of the knee. Experiments were conducted on
a dataset of 309 patients who have engaged in physical exercise for 6 months and have been grouped into two
classes, Improved state (I) and not-Improved state (nI) based on their state before (t
0
) and after the exercise
(t
6
). The method developed was able to predict I and nI patien with knee osteoarthritis using 3D kinematic
data with an accuracy of 82%. Results show the effectiveness of 3D kinematic signal analysis and the decision
tree technique for predicting the impact of physical exercise based on patient knee osteoarthritis pain level.
1 INTRODUCTION
The knee is a joint of great anatomical and biome-
chanical complexity, and is the basis for the mobility
and stability of the human body. This joint under-
goes various static and dynamic stresses that make
it subject to several degenerative diseases, including
knee osteoarthritis (OA). The World Health Organi-
zation estimates that 10% of the adult population in
developed countries suffers from osteoarthritis, 6.1%
of which affects the knee (Woolf and Pfleger, 2003).
In Canada, hundreds of thousands of people suffer
from osteoarthritis of the knee, which affects their
functional abilities and undermines their quality of
life. The prevalence of osteoarthritis is increasing as
the population ages. Indeed, professionals estimate
that it will have doubled by the year 2020 (Creamer
and Hochberg, 1997). Knee osteoarthritis is also one
of the most important chronic diseases in terms of
the use of health services (Health Council of Canada,
2007).
Although there are protocol and practice guide-
lines for better management of osteoarthritis, several
studies show that the treatment of this pathology is
far from optimal and that significant deficiencies ex-
ist both for the diagnosis and for the therapeutic man-
agement of knee osteoarthritis.The diagnosis of os-
teoarthritis can be made either by a family doctor
(general practitioner) or by an orthopedic surgeon af-
ter a musculoskeletal evaluation that can be combined
with an imaging examination (X-ray). However, be-
cause radiological examinations are performed in a
static state, they collect data on the integrity of knee
structures but do not describe the functional aspects
of the knee. While such examinations allow us to de-
termine the impact of an injury on knee function, they
are not sensitive enough to allow clinicians to make an
informed choice about the treatment to be prescribed,
particularly when it comes to prescribing physical ex-
ercise. Properly formulated exercises are very im-
portant because they aim to delay the progression of
the disease while improving symptoms and joint func-
tion. In addition, exercise is among the non-surgical
treatments that have the most scientific evidence to
support their effectiveness (Fransen et al., 2015). De-
spite this, no studies have investigated the effect of
Mezghani, M., Hagemeister, N., Kouki, M., Ouakrim, Y., Fuentes, A. and Mezghani, N.
Prediction of the Impact of Physical Exercise on Knee Osteoarthritis Patients using Kinematic Signal Analysis and Decision Trees.
DOI: 10.5220/0009191401150120
In Proceedings of the 13th International Joint Conference on Biomedical Engineering Systems and Technologies (BIOSTEC 2020) - Volume 4: BIOSIGNALS, pages 115-120
ISBN: 978-989-758-398-8; ISSN: 2184-4305
Copyright
c
2022 by SCITEPRESS – Science and Technology Publications, Lda. All rights reserved
115