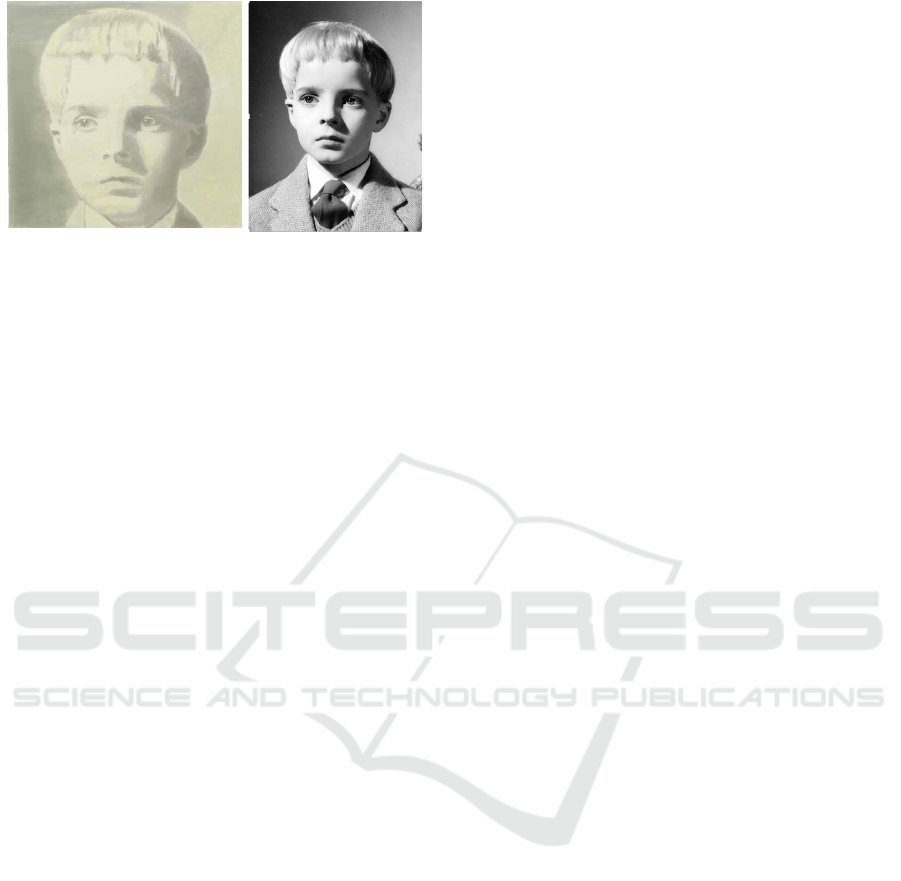
1312
LTP 386
The Valley, 2007
Oil on canvas
106.5 × 109.5 cm | 41 ⅞ × 43 ⅛ inches
Signed and dated on verso, top right: “Luc Tuymans 007”
Pinault Collection
PROVENANCE
Zeno X Gallery, Antwerp
SOLO EXHIBITIONS
Les Revenants, Zeno X Storage, Antwerp, 2007.
Wenn der Frühling kommt, Haus der Kunst, Munich, 2008.
La Pelle, Palazzo Grassi, Venice, 2019–20.
MONOGRAPHS AND SOLO EXHIBITION CATALOGUES
Norio Sugawara, Luc Tuymans: Beyond Schwarzheide, ill. iii. Tokyo:
Wako Works of Art, 2007.
Pablo Sigg and Tommy Simoens, ed., Luc Tuymans: Is It Safe?, 69,
127–28; cover, ill. 71. London: Phaidon, 2010.
Patrizia Dander and Donna Wingate, ed., Luc Tuymans: Wenn der
Frühling kommt, 9, 14; ill. 14. Ed. cat. Haus der Kunst, Munich,
2008. Antwerp: Ludion, 2014.
Frank Demaegd, ed., Luc Tuymans: Zeno X Gallery, 25 Years of
Collaboration, ill. 117, 124, 269. Exh. cat. Antwerp: Zeno X Books,
2016.
SELECTED LITERATURE
Hanno Rauterberg, “Schwach gemalt, schwach gedacht.” Die Zeit,
April 24, 2003.
Eric Rinckhout, “Tuymans schildert de macht der jezuïeten.” De
Morgen, April 21, 2007.
Frank Heirman, “Uitverkocht voor opening.” Gazet van Antwerpen,
April 25, 2007.
Jan Van Hove, “De jezuïetenstreken van Luc Tuymans.” De Standaard,
April 25, 2007.
Wim Daneels, “De jezuïetenstreken van Luc Tuymans.” Het Nieuwsblad,
April 26, 2007.
Peter van Dyck, “Luc Tuymans: De duurste Belg.” Gentleman,
April 2007, ill. 52.
Hans Theys, “Van oude spoken en dingen die niet voorbijgaan.”
H Art, May 2007, 3.
Dorine Esser, “‘Ik slaag er niet in iets vrolijks te schilderen.’” Isel,
May/June 2007, 25; ill. 25.
Jeroen Laureyns, “Geschilderde geruchten.” Knack, June 6, 2007.
Jan Koenot, “De macht van de jezuïeten en de onmacht van beelden:
Terugblik op Luc Tuymans’ serie ‘Les Revenants.’” Streven,
November 2007, 870.
“Luc Tuymans on the Failure of Utopias.” Art World, February/March
2008, ill. 16.
Rüdiger Heinze, “Malen auf des Messers Schneide.” Augsburger
Allgemeine, March 1, 2008.
Swantje Karich, “Wieviel Rätsel braucht die Geschichte?” Frankfurter
Allgemeine Zeitung, March 12, 2008.
Thomas Schönberger, “Leerstellen der Monstrosität.” Spex,
March 2008, ill. 126.
Gesine Borcherdt, “‘Wenn der Frühling kommt’ ist der Titel einer
grossen Werkschau im
Münchner Haus der Kunst.” Monopol, April 2008, 115; ill. 115.
Susanna C. Ott, “Geronnene Erinnerung.” Applaus, April 2008.
Morgan Falconer, “Luc Tuymans: Agent Provocateur.” Art World,
April/May 2008, ill. 43.
Hanno Rauterberg, “Was bedeuten diese Bilder?” Die Zeit, May 8,
2008.
Stefanie de Jonge, “De 7 hoofdzonden volgens Luc Tuymans.” Humo,
February 14, 2011, ill. 128.
Elisabeth Vedrenne, “Le monde mental de Luc Tuymans.”
Connaissance des arts, October 2016, ill. 58.
David Castenfors, “Målar Mästare Tuymans.” Artlover, no. 30, 2016,
ill. 5.
NOTES
Part of the group Les Revenants.
Figure 4: Left: ‘The Valley’ by Luc Tuymans, 2007. Oil
on canvas. 106.5 X 109.5 cm. Pinault Collection. Right:
Source image, a still from the Film ’The Valley of the
damned’ directed by Wolf Rilla, 1960. Blurring or lack
of contrast makes low-level image processing and image
recognition very challenging.
a Brazilian dystopian Netflix series called 3 % where
only few people can get to an offshore heaven-like is-
land, where the elite lives, by winning in a game. But
if they lose the game they are killed. This woman just
heard that she lost and will die by poisoning, hence
the expression of shock and dispair. The boy depicted
in Fig. 4 is from a 1960s movie based on the book
’Village of the damned’ by John Wyndham. It is an-
other dystopian story: “All the residents of the village
of Midwich become suddenly unconscious for several
hours. Months later, twelve local women and girls
give birth the same day to albino children with phos-
phorescent eyes. Precocious and able to communi-
cate by telepathy they will quickly reveal hostile in-
tentions.” (Donnadieu, 2019) The boy shown in the
painting is one of these children.
Panofsky calls this the level of conventional mean-
ing because it rests on knowing conventions in society
and knowing about historical events, well known fig-
ures, and cultural artefacts, like books or films. This
kind of meaning is imposed on the image by calling
on episodic and semantic memory or consulting ex-
ternal knowledge sources such as found on the web.
The AI methods that now come into play are based
on knowledge representation, reasoning, and seman-
tic memory, such as knowledge graphs that store vast
amounts of facts (more than 7 billion for the Google
knowledge graph). No efforts have been made so far
to model interpretation of conventional meanings in
paintings using AI, but it is not excluded to start tack-
ling this issue with current semantic technologies.
5. Finally there is the highest layer which Panof-
sky calls the intrinsic meaning or content of an art
work. Here we address the ultimate motives of the
artist, which could be political, psychological, histor-
ical, or mere story telling.
For example, the paintings shown in Figures
1 and 4 are both coming from an exhibition Les
Revenants about the enduring influence of religious
power, against which the painter wants to rebel, in
particular the influence of the Jesuits. The link to the
Jesuits is straightforward for Fig.1 because it depicts
a Jesuit church. The intrinsic meaning is established
by the distortion and the choice of “yellow, earthy and
greyish white, slightly fuzzy hues, (...) which disrupts
the splendor and magnificence of the place. In so
doing, Luc Tuymans inverts the illusionist character
of religious architecture by blurring the sculpted and
painted representations meant to inspire the faithful
and strengthen their faith.” (Donnadieu, 2019).
And even though the boy in Fig. 4 comes from a
totally different context, namely the movie ‘The Val-
ley of the damned’, it still makes a link to the Jesuits.
“The stern, stubborn gaze of the portrayed child, his
strict haircut and clothes signal harsh educational and
social norms or quasi-military upbringing.” (Don-
nadieu, 2019). This is indirectly associated with the
Jesuit educational system that wanted to form ‘sol-
diers of Christ’.
In general, the intrinsic meanings conjured up by
Tuymans create feelings of uneasiness and fear by
referring to antagonistic themes, such as the Nazi
regime, racism, child abuse, religious power, crime,
etc., and it is achieved by the selection of source im-
ages, the very constrained use of colors, image dis-
tortions, and close-ups. For example, the face in
Fig. 2 is actually the projection of a face on a doll’s
head, in order to evoke a feeling of alienation and
dystopia. Building AI systems that can handle this
level of meaning is currently totally beyond the state
of the art. Indeed, the more we get to the level of
intrinsic meaning, the more helpless current AI tech-
niques become.
The different processes going from form to mean-
ing through these five levels are not only supported by
the visual aspects of the work which, partially uncon-
sciously, affect the psychological state of the viewer.
But the title of each work and the explanations in the
catalog are also important factors that influence mean-
ing making. The painting shown in Fig.4 is called
‘The Valley’ giving a clue about the source of this
image, namely a movie with the same name. The
painting in Fig.1 is called ‘The book’, thus giving an
important cue that we are looking at an image of a
book, whereas at first one sees a scrambled interior of
a church. The title ‘twentyseventeen’ (Fig.2) refers to
the year 2017 in which the painting (and the movie)
were made. It is a dark year according to Tuymans
with the rise of populism, Brexit, and disinformation
on social media through companies like Cambridge
Analytica, so that fear and dystopia are appropriate
themes to be evoked in that year.
ICAART 2020 - 12th International Conference on Agents and Artificial Intelligence
898