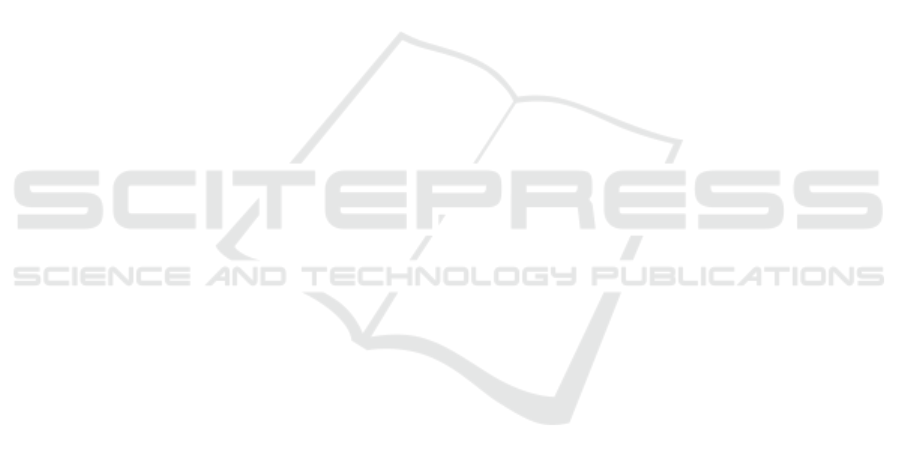
social interaction level of aging people, from multi-
modal sources of information in an ecological set-
ting. Meanwhile, monitoring activity will be paired
with strategic interventions deployed from the envi-
ronment in order to induce positive emotions, in case
of lowmood detection, and to improve the quality of
social interactions.
ACKNOWLEDGEMENTS
This work has been supported by Fondazione Cariplo,
through project Stairway to elders: bridging space,
time and emotions in their social environment for
wellbeing, grant no. 2018-0858.
REFERENCES
Anderson, D. J. and Adolphs, R. (2014). A framework for
studying emotions across species. Cell, 157(1):187–
200.
Anttonen, J. and Surakka, V. (2007). Music, heart rate, and
emotions in the context of stimulating technologies. In
International Conference on Affective Computing and
Intelligent Interaction, pages 290–301. Springer.
Boccignone, G., Conte, D., Cuculo, V., DAmelio, A.,
Grossi, G., and Lanzarotti, R. (2018). Deep construc-
tion of an affective latent space via multimodal enact-
ment. IEEE Transactions on Cognitive and Develop-
mental Systems, 10(4):865–880.
Boccignone, G., Conte, D., Cuculo, V., and Lanzarotti, R.
(2017). Amhuse: a multimodal dataset for humour
sensing. In Proceedings of the 19th ACM Interna-
tional Conference on Multimodal Interaction, pages
438–445. ACM.
Bruno, N., Uccelli, S., Viviani, E., and de’Sperati, C.
(2016). Both vision-for-perception and vision-for-
action follow weber’s law at small object sizes, but
violate it at larger sizes. Neuropsychologia, 91:327–
334.
Cao, Z., Hidalgo, G., Simon, T., Wei, S.-E., and Sheikh,
Y. (2018). Openpose: realtime multi-person 2d pose
estimation using part affinity fields. arXiv preprint
arXiv:1812.08008.
Cuculo, V., DAmelio, A., Lanzarotti, R., and Boccignone,
G. (2018). Personality gaze patterns unveiled via auto-
matic relevance determination. In Federation of Inter-
national Conferences on Software Technologies: Ap-
plications and Foundations, pages 171–184. Springer.
Cuculo, V., Lanzarotti, R., and Boccignone, G. (2015). The
color of smiling: computational synaesthesia of facial
expressions. In International Conference on Image
Analysis and Processing, pages 203–214. Springer.
de’Sperati, C. (2020). Cortical resonance to visible and in-
visible visual rhythms. Brain Sciences, Submitted.
deSperati, C. and Baud-Bovy, G. (2017). Low perceptual
sensitivity to altered video speed in viewing a soccer
match. Scientific reports, 7(1):15379.
Dias, P. A., Malafronte, D., Medeiros, H., and Odone, F.
(2019). Gaze estimation for assisted living environ-
ments. In WACV.
Ekman, P. and Friesen, W. V. (1971). Constants across cul-
tures in the face and emotion. Journal of personality
and social psychology, 17(2):124.
Fern
´
andez-Caballero, A., Mart
´
ınez-Rodrigo, A., Pastor,
J. M., Castillo, J. C., Lozano-Monasor, E., L
´
opez,
M. T., Zangr
´
oniz, R., Latorre, J. M., and Fern
´
andez-
Sotos, A. (2016). Smart environment architecture for
emotion detection and regulation. J. Biomed. Inform.,
64:55–73.
Fried, L. P., Tangen, C. M., Walston, J., Newman, A. B.,
Hirsch, C., Gottdiener, J., Seeman, T., Tracy, R., Kop,
W. J., Burke, G., et al. (2001). Frailty in older adults:
evidence for a phenotype. The Journals of Gerontol-
ogy Series A: Biological Sciences and Medical Sci-
ences, 56(3):M146–M157.
Giannakouris, K. et al. (2008). Ageing characterises the
demographic perspectives of the european societies.
Statistics in focus, 72:2008.
Grondin, S. (2010). Timing and time perception: a re-
view of recent behavioral and neuroscience findings
and theoretical directions. Attention, Perception, &
Psychophysics, 72(3):561–582.
Grossi, G., Lanzarotti, R., Napoletano, P., Noceti, N., and
Odone, F. (2019). Positive technology for elderly
well-being: A review. Pattern Recognition Letters.
Hawkins, B. A. (2005). Aging well: toward a way of life
for all people. Preventing chronic disease, 2(3).
Heitz, R. P. (2014). The speed-accuracy tradeoff: history,
physiology, methodology, and behavior. Frontiers in
neuroscience, 8:150.
Jallais, C. and Gilet, A.-L. (2010). Inducing changes
in arousal and valence: Comparison of two mood
induction procedures. Behavior research methods,
42(1):318–325.
Jones, S. R. (1992). Was there a hawthorne effect? Ameri-
can Journal of sociology, 98(3):451–468.
Mackersie, C. L. and Kearney, L. (2017). Autonomic ner-
vous system responses to hearing-related demand and
evaluative threat. American journal of audiology,
26(3S):373–377.
Mano, L. Y., Faic¸al, B. S., Nakamura, L. H., Gomes, P. H.,
Libralon, G. L., Meneguete, R. I., Filho, G. P., Gi-
ancristofaro, G. T., Pessin, G., Krishnamachari, B.,
and Ueyama, J. (2016). Exploiting IoT technologies
for enhancing Health Smart Homes through patient
identification and emotion recognition. Comput. Com-
mun., 89:178–190.
Martini, C., Barla, A., Odone, F., Verri, A., Cella, A., Rol-
landi, G. A., and Pilotto, A. (2018). Data-driven con-
tinuous assessment of frailty in older people. Frontiers
in Digital Humanities, 5:6.
Noceti, N., Odone, F., Sciutti, A., and Sandini, G. (2017).
Exploring biological motion regularities of human ac-
Stairway to Elders: Bridging Space, Time and Emotions in Their Social Environment for Wellbeing
553