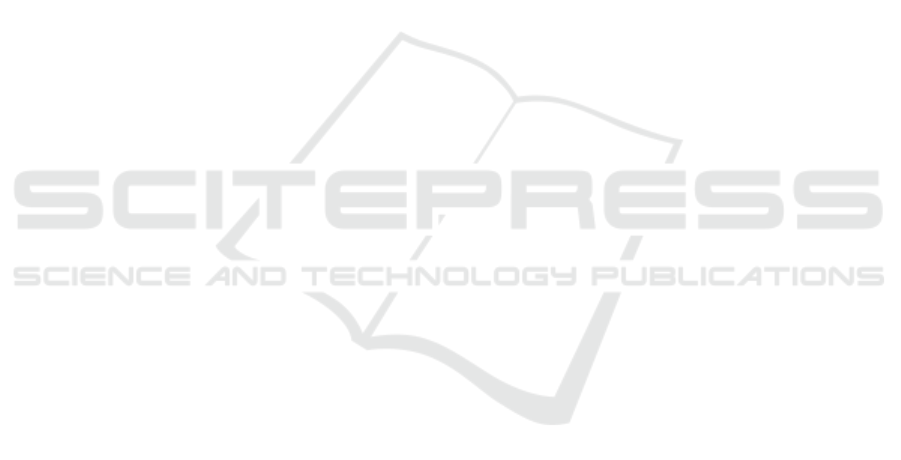
gnoses related national and international guidelines.
On the other hand one has to pay attention to aspects
of drug prescription efficiency is of crucial importan-
ce. Both result in management tools for the statutory
health insurance like regional target agreements. Mo-
dels should help to find optimal solutions with respect
to both aspects. The considered drugs are taken as no-
des of a graph and edges are given by the most equal
diagnostic structure. Starting with the local neighbor-
hood, we get a global graph structure and analyze cha-
racteristics such as graph center or graph periphery
and the graph community structure, cf. (Schuster and
Emcke, 2018). Multimorbidity and polypharmacy are
major problems in elderly patients. On average, pati-
ents above 70 years of age have drugs from more than
seven drug groups at 3rd ATC level (four digits) ad-
ministrated as a daily regime. We analyze the change
of the prescriptions from one quarter to the next by a
Markov Model in order get information about the sta-
bility of the present medication in 2018 and compare
the years 2013 and 2018 with respect to the patients
age and sex.
2 MATERIAL AND METHODS
We analyze all treatments and prescriptions of phy-
sicians for patients of the statutory health insurance
(SHI) by SHI physicians in Schleswig-Holstein, an
administration district in northern Germany (Bundes-
land). Thereby, we compare treatment and prescrip-
tion data from the first and second quarter of 2018
(two successive quarters with respect to the Markov
model) and the second quarter of 2013 in order to
get a five year comparison. The analysis is patient-
centered, meaning that the datasets of all treatments
and prescriptions of all physicians regarding an in-
dividual patient are used. The dataset of the second
quarter of 2018 covers 1,690,683 patients with dia-
gnoses and 1,383,489 patients with drug prescripti-
ons using a pseudonymized patient identity with age
and sex informations. We utilize the three-character
level of the International Statistical Classification of
Diseases and Related Health Problems [ICD]. The sa-
me diagnoses for the same patient by different phy-
sicians are not counted repeatedly. For the prescrip-
tion analysis the International Anatomic Therapeu-
tic Chemical (ATC) classification system with Ger-
man specifications provided by the German Institute
of Medical Documentation and Information (DIMDI)
is used. As antidiabetic drugs, we define the drugs of
the ATC drug groups A10A (insulins and analogues)
and A10B (blood glucose lowering drugs, excl. insu-
lins). There are 208,265 patients with diabetes E10-
E14 (Diabetes mellitus) or O24 (Diabetes mellitus in
pregnancy) and 131,296 patients of 65 years of age
and older among them. Thereby the diabetes preva-
lence regarding all patients within the statutory health
insurance and diagnosed with diabetes is 8.4 % and
the rate of persons insured benefitting from antidiabe-
tic drug therapy is 4.7 %, respectively. For persons of
65 years of age and older, we obtain prevalence values
of 23.5 % regarding diagnoses and 18.3 % regarding
antidiabetic drug therapy. For each drug d by ATC 5th
level we consider which fraction of patients has cer-
tain diagnoses (ICD at the three digit level). Thereby
we get an n-dimensional vector v
d
(n the number of
diagnoses) and a Manhatten distance of two such vec-
tors. We use drugs d by ATC 5th level(7 digits) as
vertices of a graph. With respect to each drug d we
can select m=1, 2, 3 other drug(s) at that ATC 5th le-
vel with smallest distance and get the top 1 to top 3
directed graphs with edges determined by that distan-
ce neighborhood and the induced undirected graphs
G. For these graphs we construct graph communities
using the modularity method. This is done by an ILP
(integer linear program) which is NP-hard. A related
LP (linear program) can be solved in polynomial time
and finally we have to apply a post-processing step:
the rounding of the LP to an ILP result, cf. (Shinano
et al., 2003), (Newman, 2006), (Agarwal and Kem-
pe, 2008). Further graph characteristics are the ver-
tex eccentricity which gives the length of the longest
shortest path from the source u to every other vertex
v in the graph G, the graph periphery gives vertices
that are maximally distant to at least one vertex in the
graph G and the graph center gives the set of verti-
ces with minimum eccentricity in the graph G. If we
use transition coefficients for the change of antidia-
betic drugs of patients from one quarter the next, we
obtain a Markov model. We solve the related eigenva-
lue problem for the resulting 32-dimensional matrix.
We look for an eigenvector of a maximal eigenvalue
with components of the same sign in order to get a
stable solution, which we can compare with the pre-
sent fractions of antidiabetic drugs. These drugs will
be considered in relation to patients’ diagnoses, age,
multimorbidity and polypharmacy.
3 RESULTS
36.8 % of the diabetes patients with drug therapy are
treated with metformin having average drug costs of
18.73 Euro per quarter (2nd quarter of 2018). 41.6
% of the patients are treated with cost rising up to
50.00 Euros using glibenclamide (A10BB01), metfor-
min (A10BA02), glimepiride (A10BB12), gliquidone
HEALTHINF 2020 - 13th International Conference on Health Informatics
346