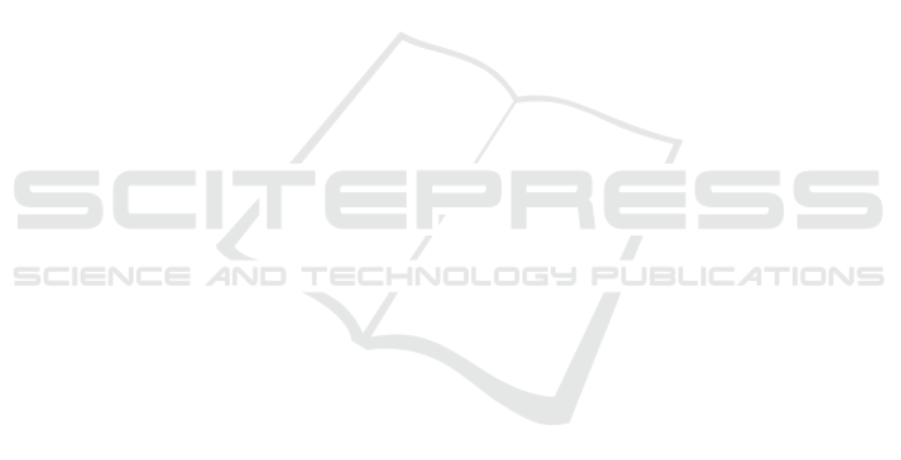
The temporal-spatial coordination, as a combina-
tion of coordination metrics, gives a clear picture of
the convergence between eye and mouse movements.
The level of correlation is somewhat lower in less un-
certain conditions. Nevertheless, the findings support
the idea that pointer tracking may be useful as an al-
ternative to eye-tracking.
REFERENCES
Annett, M. (1970). A classification of hand preference by
association analysis. Br. J. Psychol., 61:303–321.
Bechara, A. (2007). Iowa Gambling Task: Professional
Manual. Lutz, FL: Psychological Assessment Re-
sources, Inc.
Bechara, A., Damasio, A. R., Damasio, H., and Anderson,
S. W. (1994). Insensitivity to future consequences fol-
lowing damage to human prefrontal cortex. Cognition,
50:7–15.
Bieg, H.-J., Chuang, L. L., Fleming, R. W., Reiterer, H., and
Bülthoff, H. H. (2010). Eye and pointer coordination
in search and selection tasks. In Proceedings of the
2010 Symposium on Eye-Tracking Research & Appli-
cations - ETRA ’10, page 89, New York, New York,
USA. ACM Press.
Blignaut, P., Holmqvist, K., Nyström, M., and Dewhurst,
R. (2014). Improving the accuracy of video-based
eye tracking in real time through post-calibration re-
gression. In Current Trends in Eye Tracking Re-
search, pages 77–100. Springer International Publish-
ing, Cham.
Buelow, M. T. and Suhr, J. A. (2009). Construct validity of
the Iowa gambling task.
Chen, F. and Fischbacher, U. (2016). Response time and
click position: cheap indicators of preferences. Jour-
nal of the Economic Science Association, 2(2):109–
126.
Chen, M. C., Anderson, J. R., and Sohn, M. H. (2001).
What can a mouse cursor tell us more? In CHI ’01 ex-
tended abstracts on Human factors in computing sys-
tems - CHI ’01, page 281, New York, New York, USA.
ACM Press.
Cooke, L. (2006). Is the Mouse a "Poor Man’s Eye
Tracker"? In Proceeding of the 53rd Annual Con-
ference of the Society for Technical Communication,
pages 252–255.
Dillon, A. and Watson, C. (1996). User analysis in HCI -
The historical lessons from individual differences re-
search. International Journal of Human Computer
Studies, 45(6):619–637.
Djamasbi, S., Tulu, B., Loiacono, E., and Shitefleet-Smith,
J. (2008). Can a Reasonable Time Limit Improve
the Effective Usage of a Computerized Decision Aid?
Communications of the Association for Information
Systems, 23(22):393–408.
Fiedler, S. and Glöckner, A. (2012). The dynamics of deci-
sion making in risky choice: An eye-tracking analysis.
Frontiers in Psychology, 3(OCT):335.
Franco-Watkins, A. M. and Johnson, J. G. (2011). Decision
moving window: using interactive eye tracking to ex-
amine decision processes. Behavior Research Meth-
ods, 43(3):853–863.
Freeman, J. B., Dale, R., and Farmer, T. A. (2011). Hand in
motion reveals mind in motion.
Guo, Q., Jin, H., Lagun, D., Yuan, S., and Agichtein, E.
(2013). Mining touch interaction data on mobile de-
vices to predict web search result relevance. In Pro-
ceedings of the 36th international ACM SIGIR con-
ference on Research and development in information
retrieval - SIGIR ’13, page 153.
Hornof, A. J. and Halverson, T. (2002). Cleaning up sys-
tematic error in eye-tracking data by using required
fixation locations. Behavior Research Methods, In-
struments, and Computers, 34(4):592–604.
Huang, J., White, R., and Buscher, G. (2012). User see,
user point. In Proceedings of the 2012 ACM annual
conference on Human Factors in Computing Systems
- CHI ’12, page 1341.
Huang, J., White, R. W., and Dumais, S. (2011). No clicks,
no problem. In Proceedings of the 2011 annual con-
ference on Human factors in computing systems - CHI
’11, page 1225, New York, New York, USA. ACM
Press.
Just, M. and Carpenter, P. (1976). Eye fixations and cogni-
tive processes. Cognitive Psychology, 8(4):441–48.
Koop, G. J. and Johnson, J. G. (2013). The response dy-
namics of preferential choice. Cognitive Psychology,
67(4):151–185.
Lavín, C., San Martín, R., and Rosales Jubal, E. (2014).
Pupil dilation signals uncertainty and surprise in a
learning gambling task. Frontiers in Behavioral Neu-
roscience, 7:218.
Liebling, D. J. and Dumais, S. T. (2014). Gaze and mouse
coordination in everyday work. In Proceedings of the
2014 ACM International Joint Conference on Perva-
sive and Ubiquitous Computing Adjunct Publication -
UbiComp ’14 Adjunct, pages 1141–1150, New York,
New York, USA. ACM Press.
Marchionini, G. (2006). Exploratory search. Communica-
tions of the ACM, 49(4):41.
Milisavljevic, A., Hamard, K., Petermann, C., Gosselin, B.,
Doré-Mazars, K., and Mancas, M. (2018). Eye and
Mouse Coordination During Task: From Behaviour to
Prediction. In Proceedings of the 13th International
Joint Conference on Computer Vision, Imaging and
Computer Graphics Theory and Applications, pages
86–93. SCITEPRESS - Science and Technology Pub-
lications.
Navalpakkam, V., Jentzsch, L., Sayres, R., Ravi, S., Ahmed,
A., and Smola, A. (2013). Measurement and model-
ing of eye-mouse behavior in the presence of nonlin-
ear page layouts. In Proceedings of the 22nd inter-
national conference on World Wide Web - WWW ’13,
pages 953–964.
Olk, B. and Kappas, A. (2011). Eyetracking as a Tool
for Visual Research. In Margolis, E., Pauwels, L.
(eds) The Sage Handbook of Visual Research Meth-
ods, pages 433–51. Los Angeles: Sage.
BIOSIGNALS 2020 - 13th International Conference on Bio-inspired Systems and Signal Processing
36