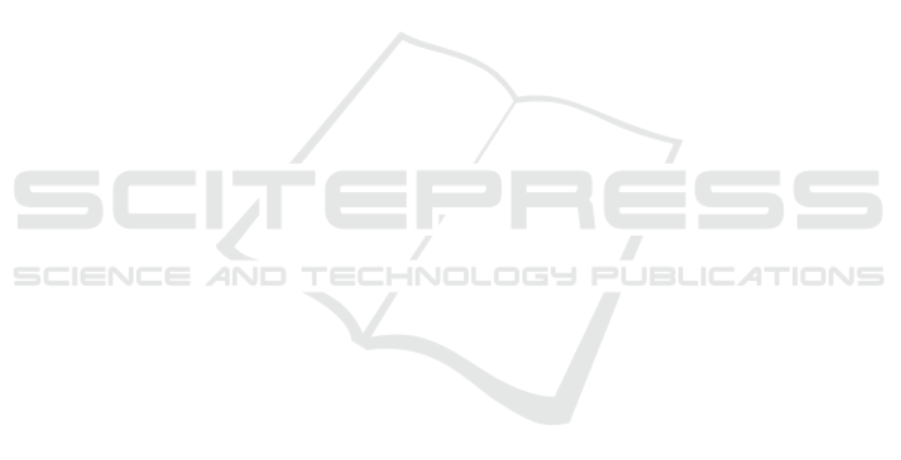
education?”.
Lavrov et al. (Lavrov et al., 2021) outlines ap-
proaches to the analysis of factors that affect the cog-
nitive independence of students. However, the ques-
tion remains: “How to implement such a study in
practice?”.
Consider the most well-known digital content
management systems designed to organize learning
processes using Internet technologies (table 1). For
almost all of these platforms, the main goal is to orga-
nize access to teaching materials, ensure interaction,
testing and reporting between teachers and students.
One of the most common distance learning sys-
tems in universities is the Moodle system, an educa-
tional platform that aims to connect teachers, admin-
istrators and students in a reliable, secure and inte-
grated system to create a personalized learning envi-
ronment (Moodle Docs, 2020; Abdula et al., 2020).
The technical aspects of the system can be de-
scribed as follows:
• Moodle is written in PHP using a SQL database,
• Moodle is installation packages and detailed in-
stallation files,
• Moodle represents different categories of users:
administrators, teachers-developers, teachers, stu-
dents, and guests.
Moodle has a wide range of features that are spe-
cific to e-learning platforms (Moodle Docs, 2020).
This allows you to organize all stages of the learning
process: diagnosis, planning, training, management
of educational activities, evaluation of results.
To diagnose and assess certain learning phenom-
ena in the Moodle system, the following technologies
are provided:
1. Questionnaire module is an activity that provides
many proven survey tools used to assess and
encourage learning in the Internet environment.
Teachers can use them to collect data from stu-
dents to help them understand the class and think
about their own teaching (Moodle Docs, 2020).
There are three types of questionnaires:
• ATTLS – Attitudes toThinkingandLearning
Survey a questionnaire containing 20 questions,
designed to determine the level of students’ at-
titudes to distance learning,
• COLLES – Constructivist On-Line Learning
Environment Survey (questionnaire “Learn-
ing Environment withEelements of Construc-
tivism”) a questionnaire containing 20 ques-
tions, designed to determine the level of stu-
dents’ attitudes to distance learning,
• Critical Incidents (questionnaire “Critical Inci-
dents”) is a questionnaire in which students are
given the opportunity to assess certain events
and their attitude to what is happening.
2. Survey module (choice) – in the classroom, you
can ask questions and set switches, and students
can press these switches to choose from a number
of possible answers. They can choose one or more
options, and if pre-settings allow, they can update
their selection. The options can be used as a quick
survey to stimulate reflection on the topic, to allow
the class to vote for the direction of the course, or
to assess progress (Moodle Docs, 2020).
3. Module test is an activity that allows teachers to
develop and build tests of knowledge, consisting
of many types of questions, including multiple
choice questions, right or wrong questions, short
answers and correspondence, and numerical ques-
tions (Moodle Docs, 2020).
4. The provided technologies can diversify distance
learning courses and make them “alive”.
5. Although these technologies have certain diag-
nostic capabilities, we believe that the main dis-
advantage of the questionnaire module is its static
nature:
• you cannot edit the questionnaire,
• other questions cannot be entered (they can
only be used in the same form as specified by
the developer).
Therefore, when teachers want to create their own
questionnaires to diagnose certain aspects of assess-
ment of learning phenomena, problems arise because
this is not provided in the system.
As the analysis of the literature shows, modern e-
learning systems do not allow to investigate the im-
portance of factors that affect cognitive independence
(in order to increase the effectiveness of learning).
Modern e-learning technologies have a wide range
of organizational capabilities at all stages of learning,
including diagnostic tools, but they are static in na-
ture, and the scope of assessment of learning phenom-
ena in them is limited.
Thus, despite the large number of studies in the
field of adaptive learning, including (Osadchyi et al.,
2020), the practice of most universities has shown a
lack of effective online learning in a pandemic. The
following facts have been recorded: decreased moti-
vation, decrease in the quality of the educational pro-
cess, refusal to study, stressful situations both among
students and teachers.
The main issues are related to the following dis-
abilities: operational research of motivational param-
AET 2020 - Symposium on Advances in Educational Technology
570