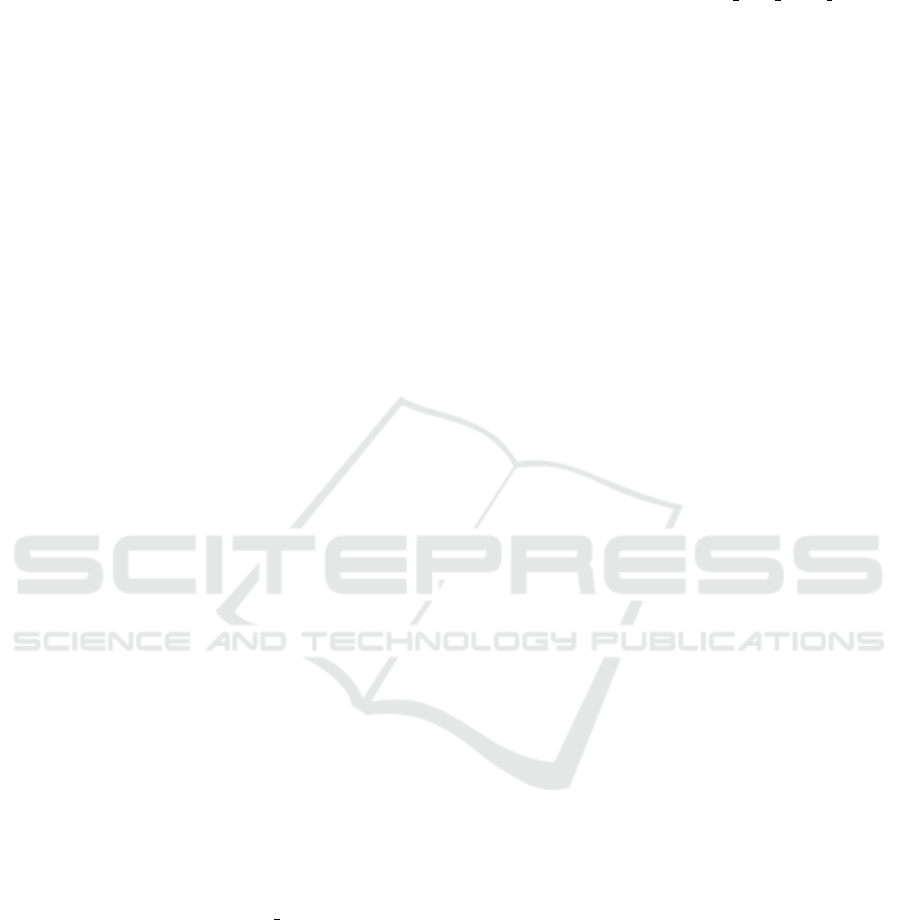
However, for those students and teachers who do not
like the Google Lens app, it is possible to use Seek or
Flora Incognita.
However, Google Lens provides only identifica-
tion without ecosystem. The Seek mobile application
can be used as a complex learning environment. It in-
cludes communications between naturalists, achieve-
ment system for motivation of the students and other
advantages.
In general, it is proven that using of AR-based
identification programs characterized by positive ef-
fect on education process and provides development
of the competencies.
REFERENCES
Agustina, W. W., Sumarto, S., and Trisno, B. (2019). Aug-
mented reality based on stem for supporting science
literacy in vocational education. Journal of Physics:
Conference Series, 1375:012088.
Antonietti, A., Imperio, E., Rasi, C., and Sacco, M. (2001).
Virtual reality and hypermedia in learning to use a
turning lathe. Journal of Computer Assisted Learn-
ing, 17(2):142–155.
Chorna, O., Hamaniuk, V. A., and Uchitel, A. (2019). Use
of YouTube on lessons of practical course of German
language as the first and second language at the ped-
agogical university. CEUR Workshop Proceedings,
2433:294–307.
Clark, A. C. and Ernst, J. V. (2008). STEM-Based Com-
putational Modeling for Technology Education. The
Journal of Technology Studies, 34(1):20–27.
de Jong, T. (2019). Moving towards engaged learning in
STEM domains; there is no simple answer, but clearly
a road ahead. Journal of Computer Assisted Learning,
35(2):153–167.
Devi, A. and Rav, G. (2018). Reviews on Augmented Real-
ity: Google Lens. International Journal of Computer
Trends and Technology, 58:94–97.
du Plessis, L. K. (2015). Through the Google Lens: Devel-
opment of lecturing practice in Photography. Master
of technology in photography, Durban University of
Technology. https://openscholar.dut.ac.za/bitstream/
10321/1437/1/DU%20PLESSIS 2015.pdf.
Dziabenko, O. and Budnyk, O. (2019). Go-Lab ecosystem:
Using online laboratories in a primary school. In ED-
ULEARN19 Proceedings, 11th International Confer-
ence on Education and New Learning Technologies,
pages 9276–9285. IATED.
Gil-Quintana, J., Malvasi, V., Castillo-Abdul, B., and
Romero-Rodr
´
ıguez, L. (2020). Learning leaders:
Teachers or youtubers? participatory culture and stem
competencies in italian secondary school students.
Sustainability (Switzerland), 12(18).
Hussein, M. and N
¨
atterdal, C. (2015). The Benefits of
Virtual Reality in Education: A Comparison Study.
Bachelor of science thesis in software engineering and
management, University of Gothenburg, Chalmers
University of Technology. https://gupea.ub.gu.se/
bitstream/2077/39977/1/gupea 2077 39977 1.pdf.
Joiner, I. A. (2018). Chapter 6 - virtual reality and aug-
mented reality: What is your reality? In Joiner, I. A.,
editor, Emerging Library Technologies, Chandos In-
formation Professional Series, pages 111–128. Chan-
dos Publishing.
Jong, T. D., Sotiriou, S., and Gillet, D. (2014). Innovations
in STEM education: the Go-Lab federation of online
labs. Smart Learning Environments, 1(3):1–16.
Kapici, H. O., Akcay, H., and de Jong, T. (2019). Us-
ing Hands-On and Virtual Laboratories Alone or To-
gether—Which Works Better for Acquiring Knowl-
edge and Skills? Journal of Science Education and
Technology, 28(3):231–250.
Khine, M. S., editor (2018). Computational Thinking in the
STEM Disciplines: Foundations and Research High-
lights. Springer, Cham.
Kinateder, M., Ronchi, E., Nilsson, D., Kobes, M., M
¨
uller,
M., Pauli, P., and M
¨
uhlberger, A. (2014). Virtual Re-
ality for Fire Evacuation Research. In Proceedings of
the 2014 Federated Conference on Computer Science
and Information Systems, volume 2, pages 313–321.
Kuzminska, O., Mazorchuk, M., Morze, N., Pavlenko, V.,
and Prokhorov, A. (2019). Study of digital compe-
tence of the students and teachers in ukraine. Com-
munications in Computer and Information Science,
1007:148–169.
Lee, E. A. L. and Wong, K. W. (2014). Learning with
desktop virtual reality: Low spatial ability learners are
more positively affected. Computers and Education,
79:49–58.
Marienko, M. V., Nosenko, Y. H., and Shyshkina, M. P.
(2020). Personalization of learning using adaptive
technologies and augmented reality. CEUR Workshop
Proceedings, 2731:341–356.
Mart
´
ın-Guti
´
errez, J., Fabiani, P., Benesova, W., Meneses,
M. D., and Mora, C. E. (2015). Augmented real-
ity to promote collaborative and autonomous learning
in higher education. Computers in Human Behavior,
51:752–761.
Modlo, Y. O., Semerikov, S. O., Nechypurenko, P. P., Bon-
darevskyi, S. L., Bondarevska, O. M., and Tolmachev,
S. T. (2019). The use of mobile Internet devices
in the formation of ICT component of bachelors in
electromechanics competency in modeling of techni-
cal objects. CEUR Workshop Proceedings, 2433:413–
428.
Morkun, V., Semerikov, S. O., Morkun, N. V.,
Hryshchenko, S., and Kiv, A. E. (2018). Defining
the structure of environmental competence of future
mining engineers: ICT approach. CEUR Workshop
Proceedings, 2257:198–203.
Park, N. (2011). The Development of STEAM Career
Education Program using Virtual Reality Technology.
Journal of Physics A: Mathematical and Theoretical,
44(8):085201.
Potkonjak, V., Gardner, M., Callaghan, V., Mattila, P.,
Guetl, C., Petrovi
´
c, V. M., and Jovanovi
´
c, K. (2016).
AET 2020 - Symposium on Advances in Educational Technology
32