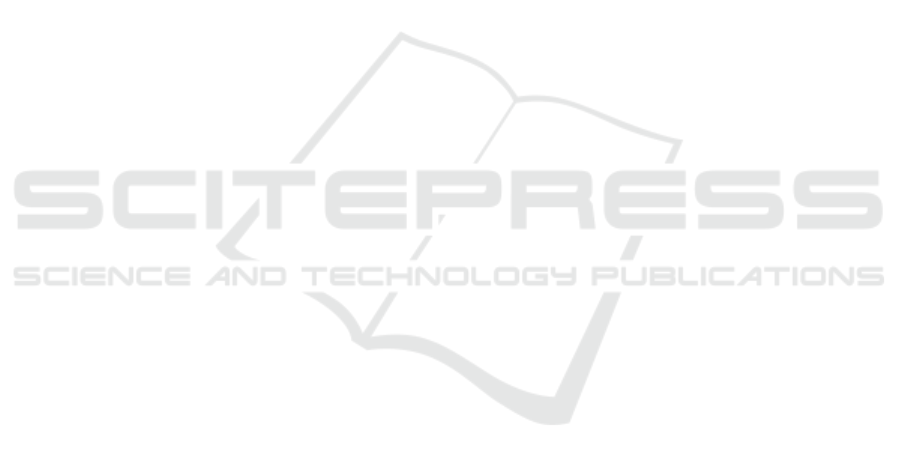
4.2 A Framework for the Combined
Use of Human and Data Centric
Complexity Assessment
The authors have examined the literature and to date
they have been unable to identify works that appreci-
ate the need to assess and manage complexity. The
authors therefore believe there is a significant gap in
the literature that needs to be addressed to i) extend
the use of data models to assess complexity in in-
dustrial settings and ii) combine the use of data mo-
dels and questionnaires/surveys to assess and there-
fore manage complexity through multiple levels of
an organisation. To address this gap, a framework
is proposed (Figure 4) that combines human-centric
and data-centric complexity assessment in combina-
tion with complexity management.
The framework consists of three layers, with a sin-
gle research pillar spanning all layers. The lowest
layer of the framework is the Data Layer. This layer
represents the core data that exists in both the minds
of humans as well as that which exists in engineering
data models. This data could exist either internal or
external to the organisation as well as at almost any
level i.e. from management down to operators. As a
result of the myriad of data sources, it is necessary to
determine what data models and which people should
be interviewed in order to get the right data. For this
reason, the research pillar exists to help identify the
relevant sources or drivers of complexity. At the data
layer there exists the information necessary to assess
complexity, but at its most raw, unprocessed form. It
is necessary for some perspective to be added to the
data in order for it to be used to assess complexity.
Note that given a different perspective, a different cri-
teria concerning an enterprise could be synthesised.
Above the Data Layer there is the Assessment
Layer. At this layer the data sources are processed
so that complexity can be assessed. If the complexity
data source is a data model, then a complexity model
is used. On the other hand, if the perceptions of hu-
mans need to be used as a complexity assessor, then
the surveys or questionnaires are used on the relevant
stakeholders. At this layer again the research pillar
is fundamental in either applying or developing the
right complexity model for the relevant data source or
asking the right questions of the given stakeholder.
Based on the results of the assessment it is neces-
sary to apply the right management strategy. This is
carried out in the Management Layer. Note that at the
Data and Assessment Layers there is a distinction be-
tween the data sources and the assessment methods.
However, at the Management Layer the framework
aims to combine the complexity assessment to un-
derstand i) how they interact, and ii) to therefore im-
plement a management strategy that complements the
assessment. The research pillar is essential in deter-
mining what management strategies exist and which
are most relevant to the results derived from the com-
plexity assessment. Once the management strategy
has been identified it is implemented which closes the
loop and allows further data to be taken from the Data
Layer to understand the impact of the management
strategy. This impact is carried out through the evalu-
ation link from the Assessment layer to the Manage-
ment layer.
The framework brings together only the ele-
ments necessary to begin to combine data-centric and
human-centric complexity assessment methods. Ho-
wever, considerable work needs to be done to deter-
mine how the data should be combined i.e. the tri-
angle spanning the left and right columns in Figure 4
representing the two complexity assessment methods.
The authors’ are still investigating a robust method to
address this problem
5 CONCLUSION
This paper has described two of the key methods as-
sociated with deriving an assessment of complexity,
namely data-centric and human centric approaches.
These two classifications have not, to the best of the
knowledge of the authors, been used to categorise
complexity assessment methods previously. The re-
spective approaches are discussed and compared with
the strengths and weaknesses being identified. This
evaluation leads to the formation of an entirely no-
vel framework that combines the two assessment met-
hods into one. As well as assessing complexity, the
framework is holistic in that it appreciates the need
to manage complexity, close the loop between asses-
sment and management, and for continuously resear-
ching to identifying new complexity sources, asses-
sment methods, and management methods. The aut-
hors strongly believe that the combination of the two
assessment methods are complementary and funda-
mental to complexity management. This idea is to
be tested as part of the case study with the industrial
partner and is on-going work.
ACKNOWLEDGEMENT
The authors gratefully acknowledge the supports of
the Commission for Technology and Innovation in
Switzerland (CTI) through the project Complexity 4.0
(ref: 18993.1), the UK EPSRC through the KDCM
COMPLEXIS 2018 - 3rd International Conference on Complexity, Future Information Systems and Risk
92