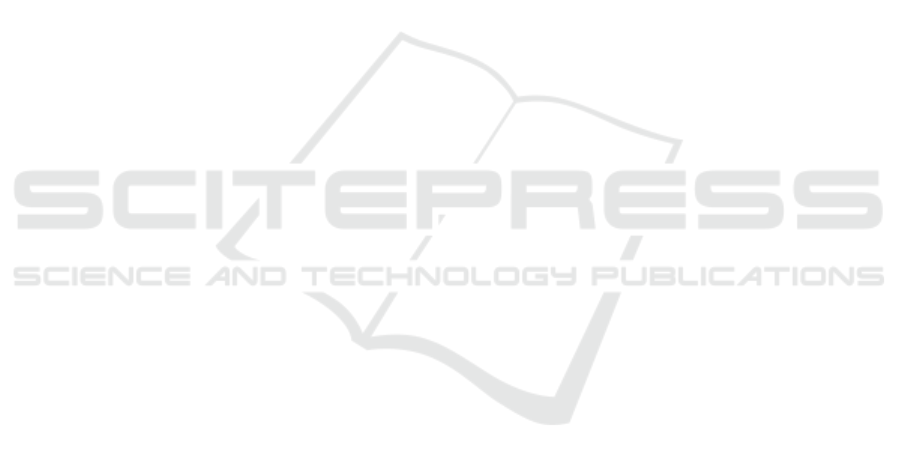
Annual Review of Information Science and Technol-
ogy Volume 31, Vol. 31:3–32.
Fishbein, M. and Ajzen, I. (1975). Belief, Attitude, Inten-
tion and Behavior: An Introduction to Theory and Re-
search. Addison-Wesley, Reading, MA, USA.
Ghyas, Q. M., Sugiura, H., and Kondo, F. N. (2012). Ex-
amining User Intention Behaviour Towards e-Readers
in Japan Using the Decomposed Theory of Planned
Behaviour. In MOBILITY 2012, The Second Interna-
tional Conference on Mobile Services, Resources, and
Users, pages 38–45, Venice, Italy. IARIA XPS Press.
Glass, R. and Li, S. (2010). Social Influence and Instant
Messaging Adoption. Journal of Computer Informa-
tion Systems, 51(2):24–30.
Godoe, P. and Johansen, T. S. (2012). Understanding adop-
tion of new technologies: Technology readiness and
technoloy acceptance as an integrated concept. Jour-
nal of European Psychology Students, 3(1):38–53.
Gombachika, H. S. and Khangamwa, G. (2013). ICT readi-
ness and acceptance among TEVT students in Univer-
sity of Malawi. Campus-Wide Information Systems,
30(1):35–43.
Guhr, N., Loi, T., Wiegard, R., and Breitner, M. H.
(2013). Technology Readiness in Customers ’ Per-
ception and Acceptance of M(obile ) -Payment : An
Empirical Study in Finland , Germany , the USA and
Japan. International Conference on Wirtschaftsinfor-
matik, March:119–133.
Huang, A. H., Yen, D. C., and Zhang, X. (2008). Explor-
ing the potential effects of emoticons. Information &
Management, 45(7):466–473.
Hyde, K. F. (2000). Recognising deductive processes in
qualitative research. Qualitative Market Research: An
International Journal, 3(2):82–90.
Jin, C. H. (2014). Adoption of e-book among college stu-
dents: The perspective of an integrated TAM. Com-
puters in Human Behavior, 41(1):471–477.
Kaiser, H. F. and Rice, J. (1974). Little Jiffy, Mark IV. Edu-
cational and psychological measurement, 34(1):111–
117.
Lancaster, S., Yen, D. C., Huang, A. H., and Hung, S.-Y.
(2007). The selection of instant messaging or e-mail:
College students’ perspective for computer communi-
cation. Information Management & Computer Secu-
rity, 15(1):5–22.
Li, D., Chau, P. Y., and Lou, H. (2005). Understanding In-
dividual Adoption of Instant Messaging: An Emprical
Investigation. Journal of the Association for Informa-
tion Systems, 6(4):102 – 129.
Lin, C.-H., Shih, H.-Y., and Sher, P. J. (2007). Integrat-
ing Technology Readiness into Technology Accep-
tance: The TRAM Model. Psychology & Marketing,
24(7):641–657.
Lu, Y., Zhou, T., and Wang, B. (2009). Exploring Chinese
users’ acceptance of instant messaging using the the-
ory of planned behavior, the technology acceptance
model, and the flow theory. Computers in Human Be-
havior, 25(1):29–39.
Mick, D. G. and Fournier, S. (1998). Paradoxes of
Technology: Consumer Cognizance, Emotions, and
Coping Strategies. Journal of Consumer Research,
25(2):123–143.
Nor, A. S. A.-A. K. M. (2015). Challenges of adoption
of internet banking service in Yemen. International
Journal of Bank Marketing, 33(2):178–194.
Parasuraman, A. (2000). Technology Readiness Index
(TRI) - A Multiple-Item Scale to Embrace New Tech-
nologies. Journal of Service Research, 2(4):307–320.
Parasuraman, A. and Colby, C. L. (2014). An updated
and streamlined technology readiness index: TRI 2.0.
Journal of service research, 18(1):1–16.
P
¨
uschel, J., Mazzon, J. A., and Hernandez, J. M. C. (2010).
Mobile banking: proposition of an integrated adoption
intention framework. International Journal of Bank
Marketing, 28:389–409.
Rennecker, J. and Godwin, L. (2003). Theorizing the Un-
intended Consequences of Instant Messaging (IM) for
Worker Productivity. Sprouts: Working Papers on In-
formation Environments, Systems and Organizations,
3(3):137–168.
Shih, Y.-C. and Fan, S.-t. (2013). Adoption of Instant
Messaging By Travel Agency Workers in Taiwan: In-
tegrating Technology Readiness with the Theory of
Planned Behavior. International Journal of Business
and Information, 8(1):120–136.
Shih, Y.-Y. and Fang, K. (2004). The use of a decomposed
theory of planned behavior to study Internet banking
in Taiwan. Internet Research, 14(3):213–223.
Shin, S. and Lee, W. J. (2014). The effects of technology
readiness and technology acceptance on NFC mobile
payment services in Korea. Journal of Applied Busi-
ness Research, 30(6):1615–1626.
Taylor, S. and Todd, P. A. (1995). Understanding informa-
tion technology usage: A test of competing models.
Teo, T. S. H. and Pok, S. H. (2003). Adoption of WAP-
enabled mobile phones among Internet users. Omega,
31(6):483–498.
Venkatesh, V., Morris, M. G., Davis, G. B., and Davis, F. D.
(2003). User Acceptance of Information Technology:
Toward a Unified View. MIS Quarterly, 27(3):pp. 425
– 478.
Walczuch, R., Lemmink, J., and Streukens, S. (2007). The
effect of service employees’ technology readiness on
technology acceptance. Information and Manage-
ment, 44(2):206–215.
Yen, J.-y. and Chen, S.-c. (2010). Perspectives from the
TRAM Model on Adopting e-Learning: An Analysis
of the Chain and Franchise Industry in Taiwan.
Mobile Instant Messaging for Customer Service Interaction - Preparation of a Model-based Approach Exploring Behavioral Intention
49