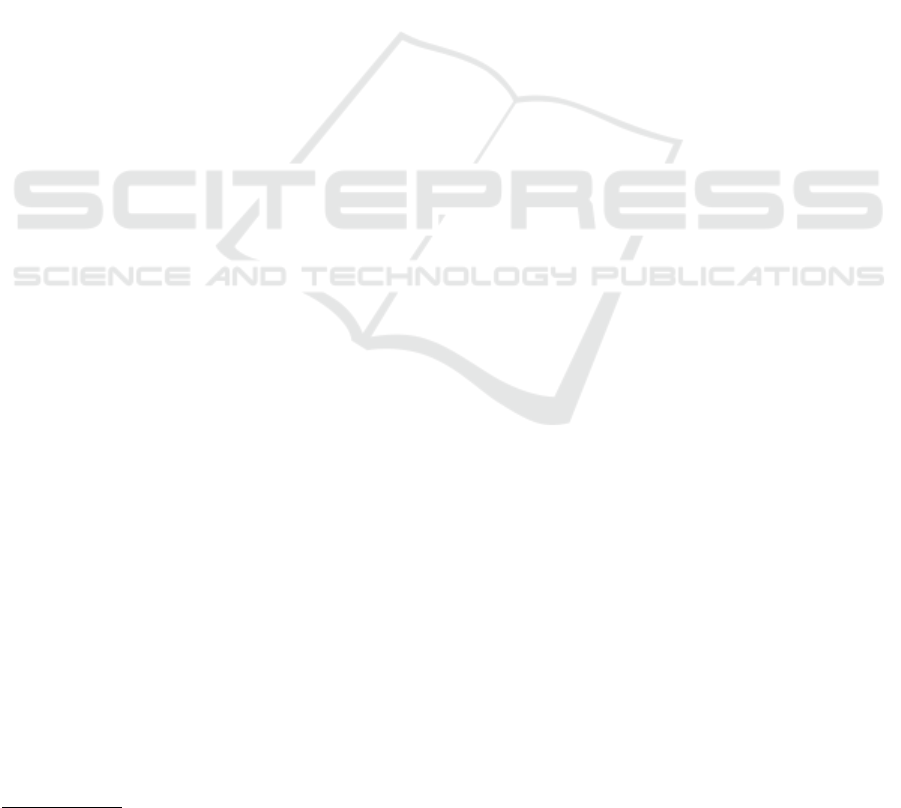
change strategies.
The general aim of the second study is to exam-
ine the path through which appraisals of the motiva-
tion afforded by the agent in a situation that leads
to a cognitive dissonance, affect people’s apprecia-
tion and cognitive consonance. We employed a sim-
ilar design as the previous study and asked partici-
pants to evaluate the different motivating agents that
were combined with the set of mathematical questions
used in the previous study. We assign randomly for
each question one of the 3 different agents. Follow-
ing previous findings from the literature, we expected
to find that appraisals of the motivating agents would
be positively associated with the appraisals of the cog-
nitive consonance obtained in the previous study. We
expected that this effect of perceived motivation af-
forded by the agent on perceived cognitive conso-
nance would, at least partially, be mediated by the ef-
fect of perceived motivation afforded by the agent on
the cognitive consonance-related characteristics (i.e.,
prospect, anxiety, and learned helplessness).
4.1 Method
We employed a within-subjects design in which par-
ticipants evaluated the perceived motivation afforded
by the agent while they are resolving the mathemati-
cal questions. The sample comprised 46 participants
(22 males and 24 females, M
age
= 30.37, SD
age
=
14.51, age range = 18 - 62 years). The participants
were registered in Ibnu Sina College.
4.2 Materials and Measures
We used the same set of mathematical questions used
in the previous study. Perceived motivation afforded
by the agent was measured using different five-point
response category format items ranging, for example,
from (1) ”Not interested” through (3) ”neutral” to (5)
”Interested”
11
. We calculated the average of the items
for each mathematical question and used this aggre-
gate score in our analysis (α = .87).
4.3 Procedure
The procedure and conditions of the second study
were analogous to those of the previous one, except
that while answering each question of the mathemati-
cal quizz, an agent speaks out loud a motivating mes-
sage so that we can ensure that the student keeps on
answering the quizz even if the questions are difficult.
In fact, if the question is difficult and the student rec-
ognizes that his answer is incorrect, he may feel dis-
11
https://goo.gl/forms/PYTnJLe44mIMkJN12
appointed. His mathematical preconceptions are de-
feated and he experiences a discrepancy between
what he believes and the answer. In such a case,
a successfully motivated student would answer the
same question that was previously answered incor-
rectly. All participants responded to the items of the
perceived motivation questionnaire.
4.4 Results and Discussion
We added the aggregated perceived motivation af-
forded by the agent measure’s score as a new vari-
able to the data set containing the prospect, anxiety,
learned helplessness, and perceived cognitive conso-
nance obtained in the previous study. Descriptives for
the measure of perceived motivation afforded by the
agent measure are presented in Table 4. We first ex-
amined the correlations between the perceived moti-
vation afforded by the agent’s measure and the mea-
sures from previous study (Table 5). We found that
perceived motivation afforded by the agent was posi-
tively correlated with perceived cognitive consonance
(r = .47, p ≤ .001) and prospect (r = .76, p ≤ .001),
and negatively correlated with anxiety (r = -.48, p ≤
.001) and learned helplessness (r = -.49, p ≤ .001).
To test whether appraisals of the perceived moti-
vation afforded by the agent predicted appraisals of
perceived cognitive consonance, we performed a re-
gression analysis. The regression model accounted
for approximately 20% of the variance in perceived
cognitive consonance with F(1,98) = 27.28, p ≤ .001,
R
2
= .22, and R
2
ad j
= .21. As expected, perceived mo-
tivation afforded by the agent was significantly related
to perceived cognitive consonance (β = .48, t = 5.22,
p ≤ .001). The regression model was moderately ro-
bust across 100 split sample validations (M
r
= .48,
SD
r
= .079, M
R
2
= .22).
Next, a multiple regression analysis was con-
ducted with both the perceived motivation afforded by
the agent and the cognitive consonance-related char-
acteristics (prospect, anxiety, learned helplessness)
as predictors. The combination of measures signifi-
cantly predicted perceived cognitive consonance with
F(4,95) = 72.31, p ≤ .001, R
2
= .75, and R
2
ad j
=
.74. However, while the measures of the cognitive
consonance-related characteristics predicted signifi-
cantly over and above the perceived motivation af-
forded by the agent measure with R
2
change = .54,
F(3, 95) = 68.53, p ≤ .001, the perceived motivation
afforded by the agent measure did not predict signif-
icantly over and above the measures of the cognitive
consonance-related characteristics with R
2
change =
.01, F(3, 95) = 1.99, p = .161. Based on these results,
perceived motivation afforded by the agent appears to
ICSOFT 2017 - 12th International Conference on Software Technologies
326