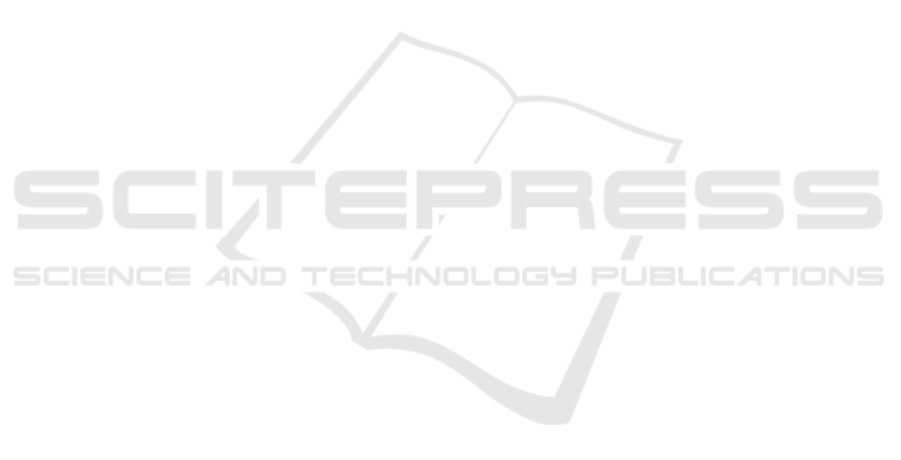
develop HBP. On the other hand, blood pressure in-
creases with age; specifically, nearly 60% of Span-
ish people older than 60 have HBP. Also important is
to remark that HBP can be associated to the onset of
other medical conditions such as chronic kidney dis-
ease, and it is also related to diabetes.
3 PATIENT CLASSIFICATION
SYSTEMS
Developed countries use PCSs for clinical purposes
and also as a basic tool for cost management. PCSs
are built from a set of clinical rules that assign each
patient to one of a limited collection of homoge-
neous groups in terms of resources use and/or health
status. The family based on DRGs (Diagnosis Re-
lated Groups) is the most used to analyze the hospital
case mix, as well as for reimbursement and financ-
ing healthcare processes. DRGs were created in Yale
University at the end of 60’s (Averill et al., 1999) as a
system to classify each healthcare episode at a hospi-
tal, creating groups with clinical coherence and sim-
ilar use of hospital resources (Averill et al., 1999).
Note that every episode of the same patient can be
assigned to a different DRG according to the type of
inpatient medical assistance. Each DRG is a group
with an associated weight reflecting the consumption
of resources respect to an average DRG considered as
a comparison unit. Some countries use this weight,
along with other metrics (hospital stay, and others), to
compare hospital activity and funding them.
One limitation of DRGs is that they only consider
the hospital environment, but in the healthcare system
there is much more activity (primary care, specialized
care, mental health, pharmacy). PCSs that consider
different fields and take into account all the patient en-
counters with the health system are named Population
Classification Systems or population groupers. As
previously indicated in Section 1, population groupers
usually consider age, gender, diagnoses and proce-
dures practiced during a certain period of time (Davis
and LaCour, 2016). Over the last years, pharmacy
dispensation has also been incorporated to the clini-
cal rules of some population groupers to have a more
complete knowledge about the health status.
Note that, since DRGs are based on individual
healthcare episodes, they do not take into account the
information of the same patient during a period of
time. Since chronic conditions tend to be reflected in
certain diagnosis and pharmacy dispensation, DRGs
are not the most appropriate system to identify pa-
tients with chronic conditions. A classification sys-
tem considers all encounters with the health system
is necessary to identify patients with chronic condi-
tions. Population groupers named CRGs (developed
by 3M
TM
) take into account data about the same pa-
tient for a period of time (usually one year (Newhouse
et al., 1997)) and assign the patient to a single mutu-
ally exclusive risk group which relates the clinical and
demographic characteristics of every individual. This
way, CRGs are suitable for identifying patients with
different chronic conditions and severity levels. This
will allow to relate an individual to the amount and
type of healthcare resources that the patient will need
in the future (Averill et al., 1999).
By way of a summary, the main differences be-
tween DRGs and CRGs are the following: (1) CRGs
categorize individuals whereas DRGs classify inpa-
tient medical assistance; (2) DRGs classify the pa-
tient into an inpatient service episode considering data
registered during the stay, whilst CRGs may consider
hospital environment in addition to primary care, out-
patient care, mental health, and pharmacy (Averill
et al., 1999).
Regarding CRGs, there are 9 core health status
groups, which are subdivided into 272 chronic condi-
tion categories (ccc). The core groups are hierarchi-
cally organized as follows: CRG-9 (catastrophic, 11
ccc), CRG-8 (metastatic malignancy, 22 ccc), CRG-
7 (chronic triplet, 21 ccc), CRG-6 (multiple signif-
icant chronic pair, 61 ccc), CRG-5 (single domi-
nant/moderate chronic, 107 ccc), CRG-4 (multiple
minor chronic pair, 1 ccc), CRG-3 (single minor
chronic, 41 ccc), CRG-2 (significant acute, 6 ccc),
and CRG-1 (healthy user and non-user, so 2 ccc). Ad-
ditionally, every chronic condition category is subdi-
vided into severity levels (up to 6, depending on the
type of condition), providing a total of 1080 groups.
A number of 5 digits is considered to code every of
the 1080 groups. First digit is associated to the core
group, next three digits refer to the ccc, and last digit
is associated to the severity level.Thus, CRG-51913
corresponds to the group of single dominant/moderate
chronic diseases (first digit), and specifically refers to
coronary atherosclerosis (next three digits), with the
third level of severity (last digit).
Patients with chronic condition of HBP can be
classified into 34 different groups (of a total of 1080):
lower CRG number refers to lower chronic condition.
Owing to the limited number of patients associated
to some severity levels, we chose to merge groups of
different severity levels when they are related to the
same chronic condition. From a statistical point of
view, it is important the availability of groups of rea-
sonable size. This way, our analysis will be focused
on the kind of chronic condition, and groups with the
same first four digits are merged to create what we
Clinical Risk Groups Analysis for Chronic Hypertensive Patients in Terms of ICD9-CM Diagnosis Codes
15