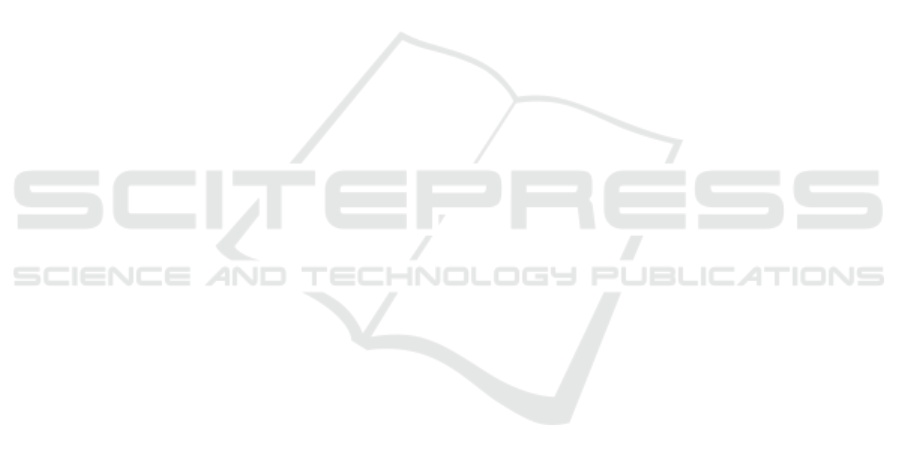
P2). 100% (in P1 and P2) means that the cerebral
tumor component is totally resected and thus the
instance of cerebral tumor component no longer
exists.
4 DISCUSSION
Our study of the VASARI terminology shows that a
concept-based approach cannot insure a faithful
representation of neuroimaging observation and that
a realism-based orientation should be adopted in this
context. We can list three main challenging points
that can be avoided when such an approach is
adopted: 1) a formal and an explicit description of
imaging features' meanings, 2) a distinction between
existing and non existing entities and 3) a following
of entities’ evolution.
In this paper, we have first discussed the
advantages and limits of concept-based and realism-
based approaches, second we have outlined the
procedure that should be followed to track relevant
entities in the domain of neuroimaging and finally
we have explained how the realism-based
orientation could be used to answer to the domain’s
requirements?
We think that the adoption of a realistic view can
help automating the generation of neuroimaging
assessments, via image processing techniques, and
covering important domain’s needs (tracking
particulars over the whole patient’s lifetime,
detecting absent entities, representing complex
situations, etc.). The transformation of neuroimaging
labels into quantitative and qualitative information
will: reduce ambiguity in clinical statements,
improve the reproducibility of assessments in
computer assisted detection and enable semantic
reasoning about involved particulars.
The main limit of our proposal is that we have
not addressed the problem of variability between
annotators. This problem of logical contradiction,
studied in (Rector et al., 1991), is due to the fact
that radiologists describe what they observe based
on their thoughts and experiences, as consequence
they may describe differently identified entities (for
example, cerebral tumors) and produce different
assertions about these entities. In this case, a
problem of logical conflicts may occur, for example,
David's cerebral tumor contains an enhancing
cerebral tumor component and David's cerebral
tumor does not contain an enhancing cerebral tumor
component. As underlined in (Smith et al., 2006),
this problem of logical contradiction is not handled
in concept-based nor in realism-based approaches.
The second limit of our proposal, implemented
in OWL, is that it does not generate temporalized
instances with this logic-based language (Smith et
al., 2006). We think that taking into consideration
the temporal aspect in the representation of
neuroimaging features is needed especially in
longitudinal imaging studies to, for example,
evaluate cancer treatment response. In this context,
we recommend to select a logic-based language that
is capable to represent ternary relationships.
To conclude, the management of information
about imaging features (measurement values,
qualities, lesion components, lesion localization,
etc.) will support clinical research on the discovery
and the validation of new imaging biomarkers.
Moreover, such information may also be used for
clinical decision support, for example, predicting
patient survival based on GBM features.
REFERENCES
Brandon, B., Vinay, C., Nikhil, D., 2013. A Vocabulary of
Topological and Containment Relations for a Practical
Biological Ontology. Spat. Inf. Theory, Lecture Notes
in Computer Science 418–437.
Ceusters, W., Elkin, P., Smith, B., 2006. Referent
tracking: the problem of negative findings. Stud.
Health Technol. Inform. 124, 741–746.
Ceusters, W., Smith, B., 2005. Tracking referents in
electronic health records. Stud. Health Technol.
Inform. 116, 71–76.
Cimino, J.J., 2006. In defense of the Desiderata. J.
Biomed. Inform. 39, 299–306..
Clunie, D.A., 2007. DICOM Structured Reporting and
Cancer Clinical Trials Results. Cancer Inform. 4, 33–
56.
Frederick Nat. Lab for Cancer Research, 2014. VASARI
Research Project - The Cancer Imaging Archive
(TCIA) [WWW Document]. URL
https://wiki.cancerimagingarchive.net/display/Public/
VASARI+Research+Project (accessed 7.14.16).
Grenon, P., Smith, B., Goldberg, L., 2003. Biodynamic
ontology: applying BFO in the biomedical domain.
Stud. Health Technol. Inform. 102, 20–38.
Rector, A., Nowlan, W., Kay, S., 1991. Foundations for an
electronic medical record. Methods Inf. Med. 30, 179–
186.
Rubin, D.L., Willrett, D., O’Connor, M.J., Hage, C.,
Kurtz, C., Moreira, D.A., 2014. Automated Tracking
of Quantitative Assessments of Tumor Burden in
Clinical Trials. Transl. Oncol. 7, 23–35.
Rubin, D., Mongkolwat, P., Channin, D., 2008. A
semantic image annotation model to enable integrative
translational research. Summit Transl. Bioinforma.
2009, 106–110.
KEOD 2016 - 8th International Conference on Knowledge Engineering and Ontology Development
184