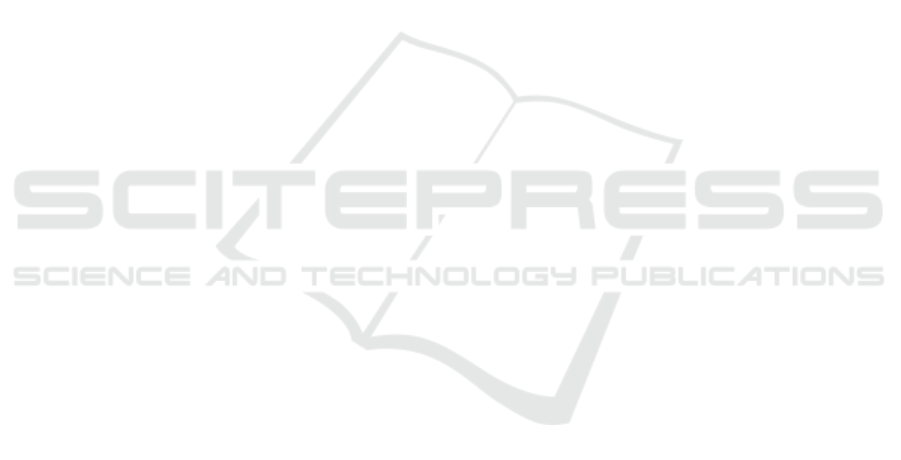
specific industry or buyers segments by understand-
ing their operating needs based on history. Remote
setting of parameter or operating conditions or pro-
viding early warning in case of machine is over used
or wrongly used as compared to what it is made for.
They can also send their maintenance staff to repair
or diagnose the problem. Such data can be collected
by the machines and transmitted to the machine man-
ufacturer. Other option is such data is collected by
the production facility and then those enterprises can
collaborate to produce or offer better services.
6 SUMMARY AND OUTLOOK
Industry 4.0 and smart manufacturing started to show
their importance in manufacturing industry. In our pa-
per, we provide an iterative approach for industry 4.0
projects. In our study, we investigate what are the
current challenges. We also discuss the new scenarios
which are possible in manufacturing industry to gain
the benefit from industry 4.0. Although, we listed
only current challenges but in future these new sce-
narios may also pose some challenges. In future, we
want to explain our approach in detail and to develop
a industry 4.0 project prototype (proof of concept) to
demonstrate the utility of our approach.
REFERENCES
Benbasat, I., Goldstein, D. K., and Mead, M. (1987). The
case research strategy in studies of information sys-
tems. MIS quarterly, pages 369–386.
Bonomi, F., Milito, R., Zhu, J., and Addepalli, S. (2012).
Fog computing and its role in the internet of things. In
Proceedings of the first edition of the MCC workshop
on Mobile cloud computing, pages 13–16. ACM.
Cisco (2015). Internet of Things(IoT). http://www.cisco
.com/web/solutions/trends/iot/portfolio.html.
Day, G. and Moorman, C. (2010). Strategy from the out-
side in: Profiting from customer value. McGraw Hill
Professional.
Eisenhardt, K. M. (1989). Building theories from case study
research. Academy of management review, 14(4):532–
550.
Eisenhardt, K. M. and Graebner, M. E. (2007). Theory
building from cases: opportunities and challenges.
Academy of management journal, 50(1):25–32.
Falk, M., Klien, M., and Schwarz, G. (2015). Large man-
ufacturing firms plan to increase their investments
in 2015. results of the wifo spring 2015 investment
survey. WIFO Monatsberichte (monthly reports),
88(7):581–591.
Figalist, D. H. (2015). On the way to industrie 4.0. driving
the digital enterprise. VDMA Informationstag, Indus-
trie 4.0 Konkret.
Hofmann, J. and Bick, P. D. W. (2015). Are variant produc-
ers the pioneers? ROI Dialog, (43):6–7.
Hofreiter, B. and Huemer, C. (2010). Flexible workflow
management in service oriented environments. In
Agent-Based Service-Oriented Computing, pages 81–
111. Springer.
Kagermann, H., Helbig, J., Hellinger, A., and Wahlster,
W. (2013). Recommendations for implementing the
strategic initiative industrie 4.0: Securing the future
of german manufacturing industry; final report of the
industrie 4.0 working group.
Kallenbach, D. R. (2015). The internet of things: Con-
nected products, connected manufacturing. internet.
Bosch Software Innovations GmbH.
Lee, E. et al. (2008). Cyber physical systems: Design
challenges. In Object Oriented Real-Time Distributed
Computing (ISORC), 2008 11th IEEE International
Symposium on, pages 363–369. IEEE.
Lee, J., Bagheri, B., and Kao, H.-A. (2015). A cyber-
physical systems architecture for industry 4.0-based
manufacturing systems. Manufacturing Letters, 3:18
– 23.
Lodhi, A., K
¨
oppen, V., Wind, S., Saake, G., and Turowski,
K. (2014). Business process modeling language for
performance evaluation. In System Sciences, 47th
Hawaii International Conference on, pages 3768–
3777.
MacDougall, W. (2014). Industrie 4.0 smart manufacturing
for the future. Germany Trade and Invest.
Madu, B. C. (2013). Vision: The relationship between a
firm’s strategy and business model. Journal of Behav-
ioral Studies in Business, 6:1. Academic and Business
Research Institute (AABRI).
Tassey, G. (2014). Competing in advanced manufacturing:
The need for improved growth models and policies.
The Journal of Economic Perspectives, pages 27–48.
Tseng, M. M. and Hu, S. J. (2014). Mass customization. In
CIRP Encyclopedia of Production Engineering, pages
836–843. Springer.
Wang, T., Yu, J., Siegel, D., and Lee, J. (2008). A similarity-
based prognostics approach for remaining useful life
estimation of engineered systems. In Prognostics and
Health Management, 2008. PHM 2008. International
Conference on, pages 1–6.
Zetter, K. (2011). Serious security holes found in
siemens control systems targeted by stuxnet.
http://arstechnica.com/security/2011/08/serious-
security-holes-found-in-siemens-control-systems-
targeted-by-stuxnet/.
Zhang, Y., Huang, G., Qu, T., and Sun, S. (2013). Real-time
work-in-progress management for ubiquitous manu-
facturing environment. In Li, W. and Mehnen, J.,
editors, Cloud Manufacturing, Springer Series in Ad-
vanced Manufacturing, pages 193–216. Springer Lon-
don.
IoTBD 2016 - International Conference on Internet of Things and Big Data
448