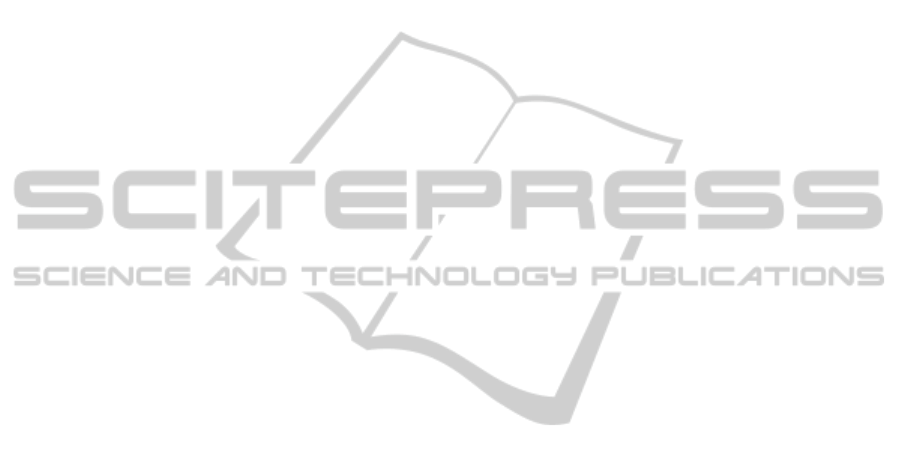
Doultsinou, A., Roy, R., Baxter, D., Gao, J. and Mann, A.,
2009. Developing a service knowledge reuse
framework for engineering design, Journal of
Engineering Design, vol. 20, no. 4, pp. 389-411.
Gruber, T. R., 1993. A translation approach to portable
ontology specifications, Knowledge acquisition, vol. 5,
no. 2, pp. 199-220.
Guarino, N., Oberle, D. and Staab, S., 2009. What is an
Ontology?, in Handbook on ontologies, Springer,
Springer, Berlin Heidelberg, pp. 1-17.
Jasper, R. and Uschold, M., 1999. A framework for
understanding and classifying ontology applications,
Proceedings 12th Int. Workshop on Knowledge
Acquisition, Modelling, and Management KAW,
Stockholm, Sweden, vol. 99, pp. 16-27.
Jiang, J., 2012. Information extraction from text, in
Mining text data, Springer, Berlin Heidelberg, pp. 11-
41.
Kim, S., Aurisicchio, M. and Wallace, K., 2009. Towards
automatic causality boundary identification from root
cause analysis reports, Journal of Intelligent
Manufacturing, vol. 20, no. 5, pp. 581-591.
Lanfranchi, V., Bhagdev, R., Chapman, S., Ciravegna, F.
and Petrelli, D., 2007. Extracting and searching
knowledge for the aerospace industry, Proceedings of
1st European Semantic Technology Conference, .
Liu, Y., Zhang, J., Tjondronegoro, D. and Geve, S., 2007.
A shape ontology framework for bird classification,
Digital Image Computing Techniques and
Applications, 9th Biennial Conference of the
Australian Pattern Recognition Society, IEEE, pp.
478-484.
Maedche, A. and Volz, R., 2001. The ontology extraction
& maintenance framework Text-To-Onto, Proc.
Workshop on Integrating Data Mining and Knowledge
Management, California, USA.
Medjaher, K., Tobon-Mejia, D. A. and Zerhouni, N., 2012.
Remaining Useful Life Estimation of Critical
Components With Application to Bearings, IEEE
Transactions on Reliability, vol. 61, no. 2, pp. 292-
302.
Norman, E. D., 2013. Mechanical Behavior of Materials,
4th ed, Pearson, England.
Okoh, C., Roy, R., Mehnen, J. and Redding, L., 2014.
Overview of Remaining Useful Life Prediction
Techniques in Through-Life Engineering Services, 6th
CIRP Conference on Industrial Product-Service
Systems (IPSS 2014), Windsor, Ontario, Canada, 1-2
May.
Pomeroy, M. J., 2005. Coatings for gas turbine materials
and long term stability issues, Materials & Design,
vol. 26, no. 3, pp. 223-231.
Q-BPM, 2014. AS-IS and TO-BE Process model, available
at: http://en.q-bpm.org/mediawiki/index.php/As-is#
Overview (accessed March/18).
Rausand, M. and Høyland, A., 2004. System reliability
theory: models, statistical methods, and applications,
New Jersey, 2
nd
edition, John Wiley & Sons.
Roy, R., Shaw, A., Erkoyuncu, J. A. and Redding, L.,
2013, Through-Life Engineering Services, Journal of
Measurement and Control, vol. 46, no. 6, pp. 172-175.
Ryu, P. and Choi, K., 2006. Taxonomy learning using
term specificity and similarity, Proceedings of the 2nd
Workshop on Ontology Learning and Population:
Bridging the Gap between Text and Knowledge,
Syndey, Australia, pp. 41-48.
Sabou, M., Wroe, C., Goble, C. and Mishne, G., 2005.
Learning domain ontologies for web service
descriptions: an experiment in bioinformatics,
Proceedings of the 14th international conference on
World Wide Web, ACM, Chiba, Japan, pp. 190-198.
Saleem, K. and Bellahsene, Z., 2008. Automatic extraction
of structurally coherent mini-taxonomies, Conceptual
Modeling-ER 2008, Springer, Berlin Heidelberg, pp.
341-354.
Serra, I., Girardi, R. and Novais, P., 2013. PARNT: A
Statistic based Approach to Extract Non-Taxonomic
Relationships of Ontologies from Text, Information
Technology: New Generations (ITNG), 2013 Tenth
International Conference, Las Vagas, Navada, pp.
561-566,
UNAS, S. M., 2000. Ontological engineering: common
approaches and visualisation capabilities, Informatica,
Vilnius, Lithuania, vol. 11, no. 1, pp. 41-48.
Uschold, M. and Gruninger, M., 2004. Ontologies and
semantics for seamless connectivity, ACM SIGMod
Record, vol. 33, no. 4, pp. 58-64.
Wang, T., Li, Y., Bontcheva, K., Cunningham, H. and
Wang, J., 2006. Automatic extraction of hierarchical
relations from text, The Semantic Web: Research and
Applications, Springer, Berlin Heidelberg, pp. 215-
229.
Zhang, Z., Gentile, A. L. and Ciravegna, F., 2011.
Harnessing different knowledge sources to measure
semantic relatedness under a uniform model,
Proceedings of the Conference on Empirical Methods
in Natural Language Processing, Association for
Computational Linguistics, pp. 991-1002.
DevelopmentofanOntologyforAerospaceEngineComponentsDegradationinService
119