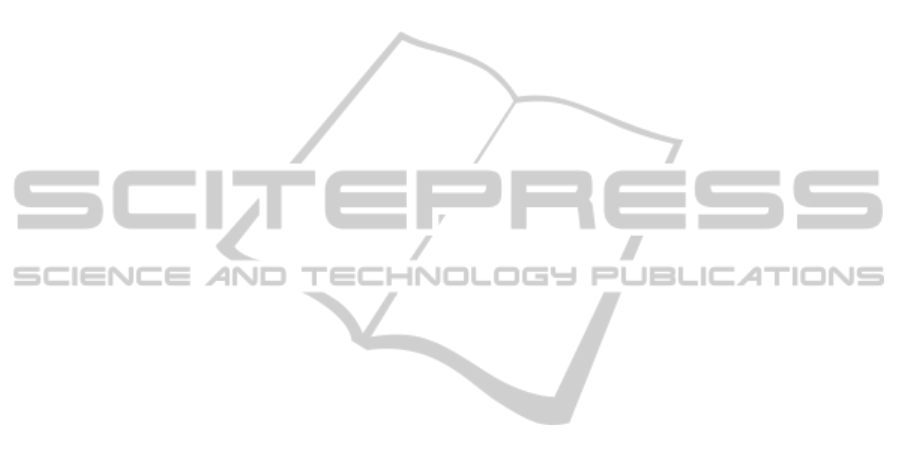
system in regard to knowledge management.
Subsequently, it introduces the requirements and
challenges of collective evaluation systems.
3.1 Terms and Definition
An evaluation procedure is a systematic process of
classifying the value judgement of an evaluating
system and a system to be evaluated (Bechmann,
1991, Bechmann, 1998). The evaluated system can
be represented as a model or it can arise as a value
system of the evaluating system (the evaluating
subject). In this case there is no limitation, whether
only experts or also ordinary persons are allowed to
participate in the evaluation process
The terms evaluation or assessment of objects
should be interpreted differently from other terms.
The description of objects is always based on
informative, factual, cognitive or indicative
statements. They are objectively comprehensible and
claim to be the description of the reality (Iwin,
1975).
Evaluations indicate what a particular person
count for as valuable, bad or indifferent. They
express convictions. Thus, every evaluation should
be put into perspective through an indication on the
evaluation person (Iwin, 1975).
Assessment or calculation defines the description
of an item, which recognizes a pure quantitative
relationship of a measurement entity. There exists a
clearly defined, mono-causal relation between the
objective of the description and the recognition of
the concrete characteristics for the actors. In this
case no value is determined but size. An evaluation
that aims to cover the result of an assessment or a
calculation is estimation (Keilhau, 1923).
Already the selection of the comparison or
evaluation criteria represents a subjective activity
and expresses the value judgment of the particular
evaluating person. Thus, evaluation and the
evaluation system lie in the focus of the operational
knowledge management.
3.2 Knowledge Management
and Evaluations
Operative knowledge management can be
characterized through the motivation of performing
a sustainable and efficient transformation of
knowledge, focusing on the company’s and process’
objectives and through the dissemination of
information through the accessibility to knowledge
(Gronau 2009; Gronau, 2010).
Knowledge is bound to person. It originates in
persons and is used by persons. Knowledge can be
based on data and information. It also represents a
combination of classified experiences, values,
context and expertise, which provides a framework
for the evaluation of these experiences and
information (Davenport and Prusak, 1998, p. 5).
Knowledge management should not be limited to
the content of knowledge. Each single actor or group
of knowledge workers contemplate the account of
knowledge for the completion of their tasks more or
less with awareness. Due to the high complexity and
dynamic of the application context, this
consideration does not emerge as an objective
assessment, calculation or estimation. It is always an
evaluation that is based on individual experiences,
insights and value judgements. The same thing
applies to performing the tasks of knowledge
management.
Knowledge management tasks include:
knowledge acquisition, knowledge preservation,
knowledge transfer, knowledge processing,
knowledge identification, knowledge evaluation,
knowledge sorting, making knowledge transparent
for others, supporting knowledge application,
determining knowledge needs and assignment of
knowledge strategies (Gronau, 2009).
Although the assessment of the result may not
always represent a subjectively performed
evaluation, it is as such for the basic evaluation
system and the implementation decision.
Objective assessments and calculations can be
helpful for certain fields of application in order to
preserve or reach competitive advantages. More
important are the many minor knowledge-based
value judgements in the day-to-day work and the
knowledge-based evaluation of complex situations.
In order to understand the decisions, we need not
only to process the knowledge content and
infrastructure but also the performed evaluation and
the applied evaluation systems. Subjective
evaluations are crucial, especially in decision
situations without sufficient knowledge basis. These
are also available for documentation.
Collective evaluations are a similar case. The
variety of information that results from a group
evaluation is even more enormous, as shown in the
next sections of this paper. This information is
available for an evaluation process controlling as
well as for a description of a collective knowledge basis.
3.3 Common Challenges
of Evaluation Systems
The evaluation describes a system and its characteristics
KMIS 2011 - International Conference on Knowledge Management and Information Sharing
160