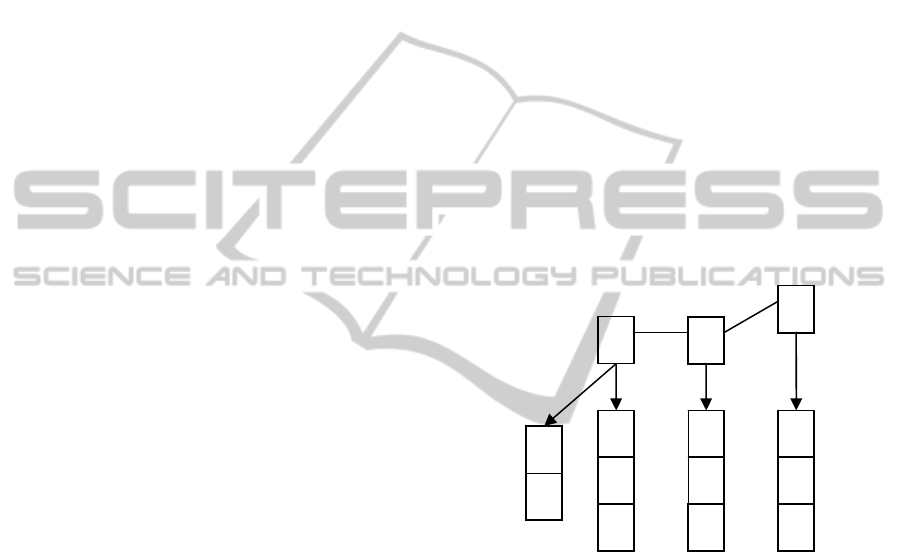
small groups in the network which has excessive
structural holes. If the network which the actor
embeds is a reciprocal relationship network, he will
pass information between two small groups which
have no strong relationship, and the actor's role
become a "bridge "—a position that can stimulate
the circulation and sharing of knowledge. In a large
creative team, the bridges are essential. For example,
some key members of different groups play a bridge
role. Through the bridge, small groups can get some
overlapping research knowledge to promote
innovation activities.
2.2 Small Group
Small group (subgroup or cliques) is a sub-group in
which the relationships of the members are
particularly close. Small groups can match the
factions, which is the overall structure indicators of
network.
There are two kinds of methods for the
calculation of small groups. First, calculate the node
degree, and view a group of connected nodes as a
small group. Second, nodes that can be achieved in
the distance will be viewed as a small group. In this
paper, we select the most commonly used method—
K-plex.
K-plex is a Sub-graph that contains g
s
nodes. In
the graph, each node is connected with g
s
-k nodes in
the same sub-graph.
The presence of small groups of
knowledge-sharing activities has both positive and
negative effects. On the one hand, the small group
members can maintain a high level of strong ties, to
strengthen knowledge sharing effect, and to
stimulate knowledge innovation within small groups.
In a large innovation team, members of different
sub-groups easily form small groups, which is
conducive to concentrate their limited forces and
improve the stage activities. Another aspect is that if
a small group is too self-closing, the knowledge of
outside groups can not enter, and the knowledge
within the group can not be shared. Extreme cases
are: in a team that lacks of a common vision, each
member come together only for research or
development, and even the division of labor and
cooperation can not be fully realized. In such team,
knowledge sharing will not be able to achieve, and
accumulation of knowledge and innovation also can
not be achieved for the whole team.
3 BACKGROUND AND DATA
PROCESSING
In this paper, we select a university innovation
research team as a knowledge-sharing networks
quantitative study object. The purpose of the study is
to discover the team's knowledge sharing structure
and the status, to identify and solve problems in the
knowledge flow and to improve the flow efficiency
within the organization.
In the team, there are 14 members engaged in the
development of an information system project. We
use letters A ~ N to indicate the members. The
team's organizational structure is shown as figure 1.
A is the leader of the team, while leading a team
contained of B, K and H. C and D are assistants of A
and they both lead a research team. C’s group is the
largest, and responsible for the most expensive part
of the project. The organizational structure will be
compared to the knowledge-sharing network in
order to discover problems in the knowledge flow.
Figure 1: The team's organizational structure.
In accordance with the requirements of the
whole network analysis, we first determine the
analysis unit and social network boundary. This
network is a closed social network composed of 14
members. Then, we determine the important
relationship dimension to be analyzed. In this paper
we use the 1 - mode network, which only measure
the exchange of knowledge among the team
members. Then, we design the questionnaire. There
are 3 survey questions to answer, including "When
confronted with the knowledge and technology
difficulties, who would you ask for help?", “Who do
you often get the most substantive help from? " and
" who do you discuss the situation of the project
with?". Three questions are designed to get more
knowledge sharing status. Based on the analysis,
ultimately the relationship between members is a
C
D
A
I
M
L
G
N
F
E
J
B
K
H
ICEIS 2011 - 13th International Conference on Enterprise Information Systems
440