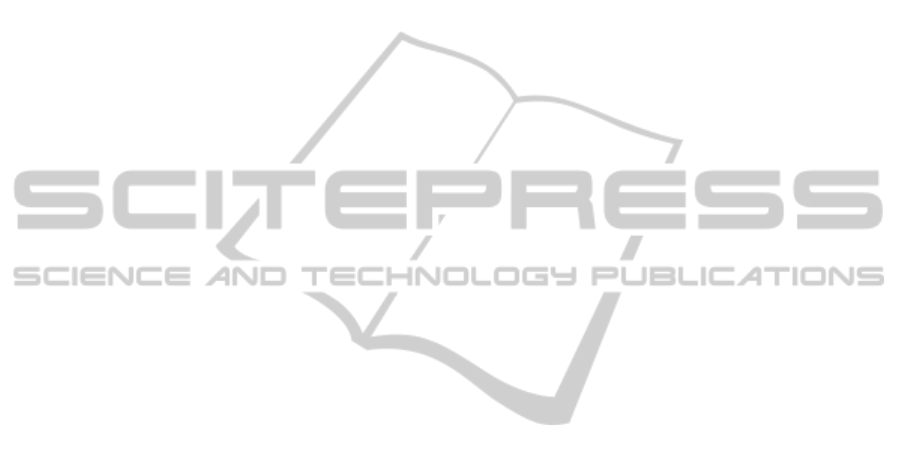
• many stressful emotions have been identified,
these may be different kinds of stress; and
• restrictions applying to storytelling for emotion
elicitation may have influenced the results.
These three restrictions can be seen as future research
challenges. Namely, to use other patient groups, emo-
tions, and emotion elicitation techniques.
Using the acoustic profile, one can arrive at an
ATA for the diagnosis and treatment of stress-related
psychiatric disorders. An ATA can help the clinical
setting in several ways, to:
1. support the measurement of stress responses;
2. give decision support on whether a patient suffers
from excessive stress;
3. aid the treatment of stress disorders; and
4. improve self-help and minimal-contact therapy
methods (Newman et al., 2010).
Through making the measurement objective, the mea-
surement of stress becomes more reliable; i.e., no
longer solely relying on introspection. Objective mea-
surement also increases inter- and intra-expert relia-
bility. Moreover, diagnosis, decision-making in gen-
eral, and treatment could become more fine-grained.
Concluding, an important and significant step to-
wards an ATA for stress-related psychiatric disorders
has been made. This study has shown that an objec-
tive measurement of stress through speech is feasible.
Par excellence, the feasibility of objective stress mea-
surement illustrates the possibility of more objective
measures for the generally subjective fields of psy-
chology and psychiatry.
ACKNOWLEDGEMENTS
We gratefully acknowledge the PTSD patients for vol-
untarily participating in this research. We thank Lynn
Packwood for proof reading this article.
REFERENCES
American Psychiatric Association (2000). DSM-IV-TR: Di-
agnostic and Statistical Manual of Mental Disorders.
Washington, DC, USA: American Psychiatric Pub-
lishing, Inc., 4 (Text Revision) edition.
Banse, R. and Scherer, K. R. (1996). Acoustic profiles in
vocal emotion expression. Journal of Personality and
Social Psychology, 70(3):614–636.
Boersma, P. (1993). Accurate short-term analysis of the fun-
damental frequency and the harmonics-to- noise ratio
of a sampled sound. In Proceedings of the Institute of
Phonetic Sciences, volume 17, pages 97–110. Univer-
sity of Amsterdam.
Boersma, P. P. G. and Weenink, D. J. M. (2006). Praat
4.0.4. http://www.praat.org (Last accessed on Octo-
ber 22, 2010).
Caffi, C. and Janney, R. W. (1994). Toward a pragmatics of
emotive communication. Journal of Pragmatics, 22(3–
4):325–373.
Cohen, P. R. and Oviatt, S. L. (2002). The role of voice
input for human-machine communication. Proceed-
ings of the National Academy of Sciences (PNAS),
92(22):9921–9927.
Cousineau, D. (2005). Confidence intervals in within-
subject designs: A simpler solution to Loftus and
Masson’s method. Tutorials in Quantitative Methods
for Psychology, 1(1):42–46.
El Ayadi, M., Kamel, M. S., and Karray, F. (xxxx). Survey
on speech emotion recognition: Features, classifica-
tion schemes, and databases. Pattern Recognition, [in
press].
Everly, Jr., G. S. and Lating, J. M. (2002). A clinical guide
to the treatment of the human stress response. The
Plenum series on stress and coping. New York, NY,
USA: Kluwer Academic / Plenum Publishers, 2nd edi-
tion.
Harrell, Jr., F. E. (2001). Regression modeling strategies
– with applications to linear models, logistic regres-
sion, and survival analysis. Springer Series in Statis-
tics. New York, NY, USA: Springer-Verlag New York,
Inc., 1st; 6th printing edition.
Kedem, B. (1986). Spectral analysis and discrimina-
tion by zero-crossings. Proceedings of the IEEE,
74(11):1477–1493.
Kessler, R. C. (1997). The effects of stressful life
events on depression. Annual Review of Psychology,
48(1):191–214.
Lader, M. (1975). The psychophysiology of mental illness.
London, Great Britain: Routledge & Kegan Paul Ltd.
Lazarus, R. S. (1993). From psychological stress to the
emotions: A history of changing outlooks. Annual
Review of Psychology, 44(1):1–22.
Lyons, R. G. (2004). Understanding Digital Signal Process-
ing. Upper Saddle River, NJ, USA: Prentice Hall PTR,
2nd edition.
Newman, M. G., Szkodny, L. E., Llera, S. J., and Prze-
worski, A. (2010). A review of technology-assisted
self-help and minimal contact therapies for anxiety
and depression: Is human contact necessary for ther-
apeutic efficacy? Clinical Psychology Review, [in
press].
Scherer, K. R. (2003). Vocal communication of emotion:
A review of research paradigms. Speech Communica-
tion, 40(1–2):227–256.
Van den Broek et al., E. L. (2009/2010). Prerequisites
for Affective Signal Processing (ASP) – Parts I–IV.
In Fred, A., Filipe, J., and Gamboa, H., editors,
BioSTEC 2009/2010: Proceedings of the Interna-
tional Joint Conference on Biomedical Engineering
HEALTHINF 2011 - International Conference on Health Informatics
362