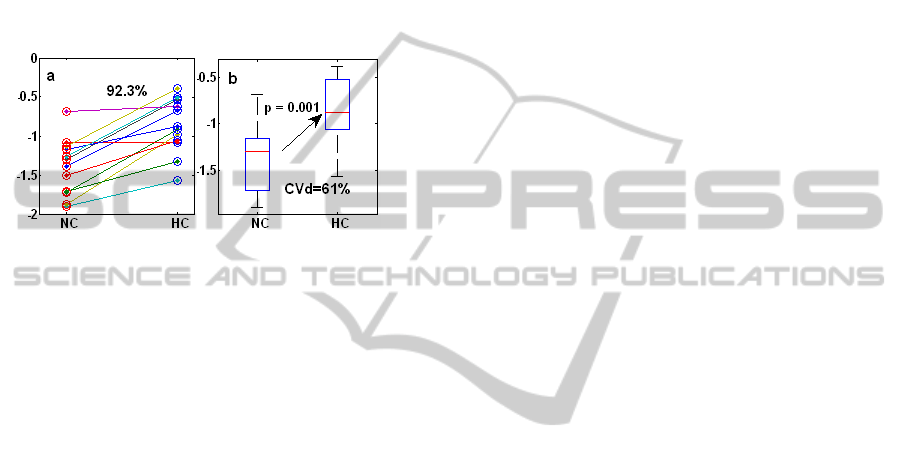
value of the step response, compared to some of the
measures extracted from the pulse response, as
indicated by CVd (see Figure 4). The simulations
indicate that intra-subject variability, due to assumed
additive noise in the recordings is a large contributor
the overall dispersion in results. However in this
respect, H1 outperformed all other measures. H1
was also among the best in terms of inter-subject
variability. It should also be noted that model fit
(Table 1) alone clearly is not a good indicator of
what makes for the best method in the assessment of
autoregulation.
Figure 5: a) Magnitude of H1 during normocapnia (NC)
and hypercapnia (HC). b) Box plots representation of the
median, quartile, minimum and maximum of H1.
A number of other parameters for assessing
autoregulation, including parameters taken directly
from the model (as H1 for the first order FIR filter)
were also investigated, but none proved superior to
the ones presented. The relatively small sample of
recordings available and relatively large number of
parameters tested is a limitation of the current study.
It is possible that the relative performance of the
methods reflects peculiarities or random effects in
the particular data set available. Given that
parameters estimated are not independent, the usual
methods for determining statistically significant
differences between the approaches are not
appropriate. However, the large differences observed
between methods, probably do indicate which
approaches are most promising to be taken on in a
further study on a larger data set.
6 CONCLUSIONS
In this work we have compared a number of
measures to evaluate autoregulatory activity. Some
of the parameters extracted from the proposed pulse
input show less variability compared with the more
conventional parameters extracted from the
frequency and step responses. Thus relatively small
variations across as well as within subjects were
found for the amplitude of the pulse response at
certain lags (A1.5, A6). These also showed a clearer
distinction between the different levels of
autoregulation (quantified by the p value). In
particular, for linear models A1.5 would be
recommended whereas A6 seemed to perform well
for nonlinear models. However, the results obtained
from H1 suggest that this parameter from a very
simple model might be the method of choice, with
small coefficients of variation (CVd, mCVd). This
method also allows the analysis of relatively short
data segments and thus lends itself to further studies
of time-varying (adaptive) estimates of dynamics in
cerebral blood flow control.
ACKNOWLEDGEMENTS
We would like to thank Drs. Stephanie Foster and
Lingke Fan and Prof. David Evans (Leicester Royal
Infirmary) for generously providing the anonymized
data used in this study and Innovation China UK for
funding support.
REFERENCES
Aaslid, R. et al., 1989 Cerebral autoregulation dynamics
in humans. Stroke, 20:45-52.
Angarita-Jaimes, N. et al., 2010. Nonlinear modelling of
CA using cascade models. Proc. Medicon 2010,
Greece.
Birch, A. et al., 2002. The repeatability of CA using
sinusoidal lower -ve pressure. Phys. Meas, 23,73-83
Liu, Y. et al., 2003 Dynamic cerebral autoregulation
assessed using an ARX model. Med. Eng. Phys., 25.
Liu, J. et al., 2005. High spontaneous fluctuation in ABP
improves the assessment of CA. Physiol. Meas, 26
Mitsis, G. et al., 2004 Nonlinear modelling of dynamic
CBF in healthy humans. Adv Exp Med Biol. 551:259-
265.
Panerai, R. et al., 1998. Grading of CA from spontaneous
fluctuations in ABP. Stroke, 29:2341-2346.
Panerai, R. 1998. Assessment of CA in humans -a review
of measurement methods. Physiol.Meas. 19.
Panerai, R. et al., 1999. Linear and nonlinear analysis of
human dynamic CA. Am J Heart Circ Physiol. 277
Panerai, R. et al., 2003. Variability of time-domain indices
of dynamic CA Physiol Meas, 24:367–381.
Piechnik, S. et al., 1999 The continuous assessment of
cerebrovascular reactivity Anesth Analg, 89:944–9
Simpson, D. et al., 2001. A parametric approach to
measuring CA Ann Biomed Eng, 29:18–25.
Simpson, D. and Birch, A., 2008. Optimising the
assessment of CA from black-box models. Proc.
MEDSIP 2008.
Tiecks, F. et al., 1995. Comparison of static and dynamic
CA measurements. Stroke 26: 1014-1019.
Westwick, D. and Kearney, R., 2006. Identification of
Nonlinear Physiological Systems. IEEE Press, 1st Ed.
Zhang R et al., 1998 Transfer function analysis of dynamic
CA in humans. Am. J. Physiol., 274.
BIOSIGNALS 2011 - International Conference on Bio-inspired Systems and Signal Processing
256