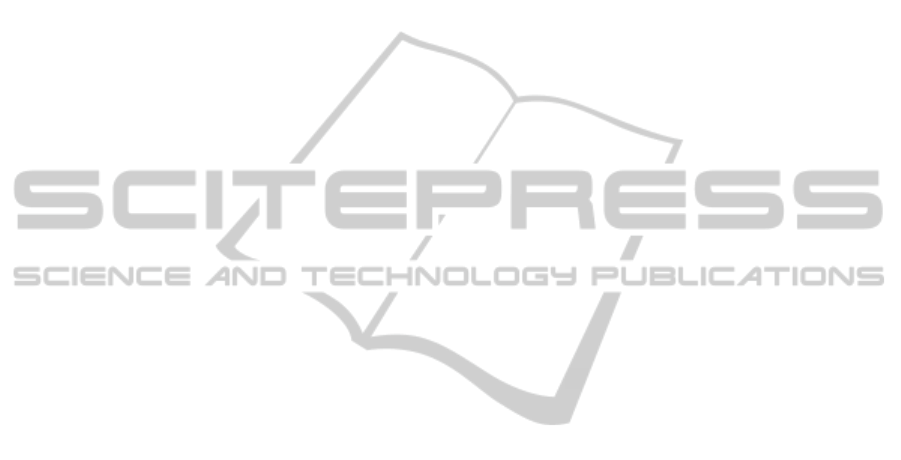
more closely to the characteristics of the
distributions associated with the noise level and the
speed of motion. In addition, the variable speed may
ensure optimal stimulation of the units in the brain,
sensitive to motion direction.
4 CONCLUSIONS
The common analytical approaches used to portray
the age-related changes in the different cognitive
processes provide a good description of the trends
associated with ageing and the experimental
conditions when differences occur. This makes them
a useful tool to characterize the process of ageing
and to seek association between the behavioural data
and the physiological changes in the brain. However,
these methods are less efficient when the individual
differences in the ageing process need to be
evaluated.
Our study is a first attempt to apply clustering
algorithms to differentiate the effects of age, gender
and individual differences on performance of a
behavioural task. The interpretation of the clustering
results allows detecting the deviation level of a
subject from the respective age group. Whether this
is related to degenerative processes or not, could not
be determined only by the results of the present
study; it requires tracking the changes in the
cognitive abilities of the participants in longitudinal
studies. However, our data analytic technique
provides opportunities to use psychophysical
methods for early diagnostics of the deterioration in
the cognitive abilities of the individual with age. The
results of the cluster analysis suggest also, that it is
easier to detect the individual deviations in
demanding tasks and difficult experimental
conditions.
ACKNOWLEDGEMENTS
This work was supported by grant TK01-200 of the
National Science Fund, Bulgaria.
REFERENCES
Habak, C., & Faubert, J., 2000. Larger effect of aging on
the perception of higher-order stimuli. Vision
Research, 40: 943–950.
Raz N., 2004. The aging brain observed in vivo:
differential changes and their modifiers. In: Cabeza R.,
Nyberg L. & Park D.C. eds. Cognitive neuroscience of
aging: linking cognitive and cerebral aging. New
York: Oxford University Press, 17-55.
Govenlock, S., Taylor, C. P., Sekuler, A. B. & Bennett , P.
J., 2009. The effect of aging on the orientation
selectivity of the human visual system. Vision
Research, 49: 2273-2284
Berard, J. R., Fung, J., McFadyen, B. J. & Lamontagne,
A., 2009. Aging affects the ability to use optic flow in
the control of heading during locomotion.
Experimental Brain Research, 194(2): 183-190.
Pilz, K. S., Bennett. P. J. & Sekuler, A. B., 2010. Effects
of aging on biological motion discrimination. Vision
Research, 50(2): 211-219
Allen, H. A., Hutchinson, C. V., Ledgeway, T., & Gayle,
P., 2010. The role of contrast sensitivity in global
motion processing deficits in the elderly. Journal of
Vision, 10: 15, 1–10,
Billino, J., Bremmer, F., & Gegenfurtner, K. R., 2008.
Differential aging of motion processing mechanisms:
Evidence against general perceptual decline. Vision
Research, 48: 1254–1261.
Rose, N. S., Rendell, P. G., McDaniel. M. A., Aberle, I., &
Kliegel, M., 2010. Age and individual differences in
prospective memory during a "Virtual Week": the
roles of working memory, vigilance, task regularity,
and cue focality. Psychology and Aging, 25: 595-605
Busey T., Craig J., Clark C. & Humes L., 2010. Age-
related changes in visual temporal order judgment
performance: Relation to sensory and cognitive
capacities. Vision Research, 50(17):1628-1640.
Hedden T. & Gabrieli, J. D., 2004. Insights into the ageing
mind: a view from cognitive neuroscience. Nature
Revues Neuroscience, 5: 87-96.
Watson, A. B. & Pelli, D. G., 1983. QUEST: a Bayesian
adaptive psychometric method. Perception &
Psychophysics, 33(2): 113-20.
Rencher, A. C., (1995). Methods of multivariate analysis.
John Wiley & Sons, Inc., New York.
Bezdek, J. C., 1981. Pattern Recognition with Fuzzy
Objective Function Algorithms. Plenum Press. New
York.
Babuska, R., 1998. Fuzzy Modeling for Control. Kluwer
Academic Publishers. Boston.
Lu, Z.-L., Dosher, B. A. 2009. Mechanisms of Perceptual
Learning. Learning and Perception, 1: 19–36.
DATA ANALYSIS OF AGE-RELATED CHANGES IN VISUAL MOTION PERCEPTION
561